Dagster Data Engineering Glossary:
Data Denormalization
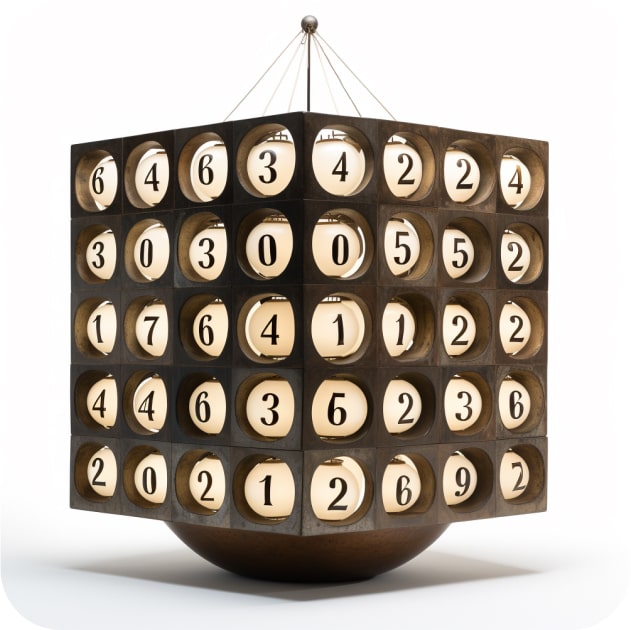
Data denormalization definition:
Denormalization is the process of adding redundant data to a database to improve read performance. It involves breaking with normalization rules in order to reduce the number of joins needed to retrieve data. This can be important for improving query performance in data pipelines that have to handle large amounts of data.
Denormalization can be a useful technique for improving query performance in your data pipelines, but it should be used judiciously and with an understanding of the trade-offs involved. Denormalizing data can lead to increased data redundancy and the risk of data inconsistencies.
Data denormalizing in Python:
Please note that you need to have the necessary Python libraries installed in your Python environment to run the code examples below.
In Python, one way to denormalize data is to use the pandas.merge()
function to combine data from multiple tables into a single DataFrame. For example, if you have a table of customers and a table of orders, you could denormalize the data by merging the two tables based on the customer ID column:
Given input files of customer.csv as:
customer_id,name,total_orders
1,Mary,42
2,Brian,28
3,Mercedes,7
4,Rose, 20
And an orders.csv
file of:
customer_id,item,value
1,cat food,12
1,apples,6
1,books,24
2,bananas,12
2,candles,12
2,coffee,4
3,nails,7
4,paper,18
4,pencils,2
You can merge this data as follows:
import pandas as pd
# Load the customer and order tables into DataFrames
customers = pd.read_csv('customers.csv')
orders = pd.read_csv('orders.csv')
# Merge the two tables based on the customer ID column
merged_data = pd.merge(customers, orders, on='customer_id')
print(merged_data)
This would create a new DataFrame that includes all of the columns from both the customers and orders tables, with the data merged together based on the customer_id
column and would look like this:
customer_id name total_orders item value
0 1 Mary 42 cat food 12
1 1 Mary 42 apples 6
2 1 Mary 42 books 24
3 2 Brian 28 bananas 12
4 2 Brian 28 candles 12
5 2 Brian 28 coffee 4
6 3 Mercedes 7 nails 7
7 4 Rose 20 paper 18
8 4 Rose 20 pencils 2
Another way to denormalize data in Python is to use a document-oriented database like MongoDB, which allows you to store data in a denormalized format as documents. This can be useful for handling complex, nested data structures that don't fit well into traditional relational database tables. With MongoDB, you can store related data together in a single document, rather than splitting it up into multiple tables with foreign key relationships.
Suppose we have two collections in our MongoDB database: "users" and "orders". The "users" collection contains documents with information about each user, such as their name and email address. The "orders" collection contains documents with information about each order, including the user ID of the user who placed the order.
Here's how we can denormalize this data by embedding user information within each order document:
import pymongo
# Connect to MongoDB
client = pymongo.MongoClient("mongodb://localhost:27017/")
db = client["mydatabase"]
# Get collections
users_col = db["users"]
orders_col = db["orders"]
# Find all orders
orders = orders_col.find()
# Denormalize data
for order in orders:
user = users_col.find_one({"_id": order["user_id"]})
order["user_name"] = user["name"]
order["user_email"] = user["email"]
# Update order document with denormalized user data
orders_col.update_one(
{"_id": order["_id"]},
{"$set": {
"user_name": order["user_name"],
"user_email": order["user_email"]
}}
)
In this example, we first connect to our MongoDB database and get references to the "users" and "orders" collections. We then find all orders in the orders
collection and denormalize each order document by embedding the user's name and email within the order document. Finally, we update the orders
collection with the denormalized data.
Denormalizing data in this way can be useful for optimizing read performance, as it reduces the need to perform multiple queries to retrieve related data. However, it can also increase write complexity and potentially lead to data inconsistency if the denormalized data is not kept up-to-date with changes to the original data. Therefore, it's important to carefully consider the trade-offs before denormalizing data.
Align
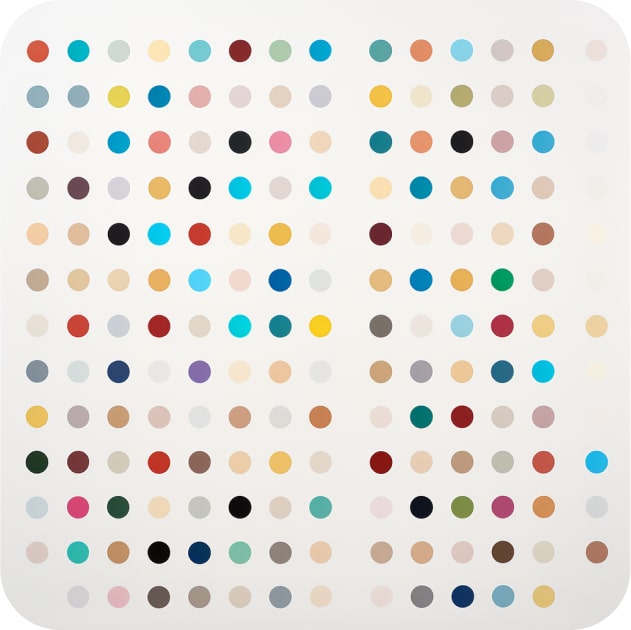
Clean or Cleanse
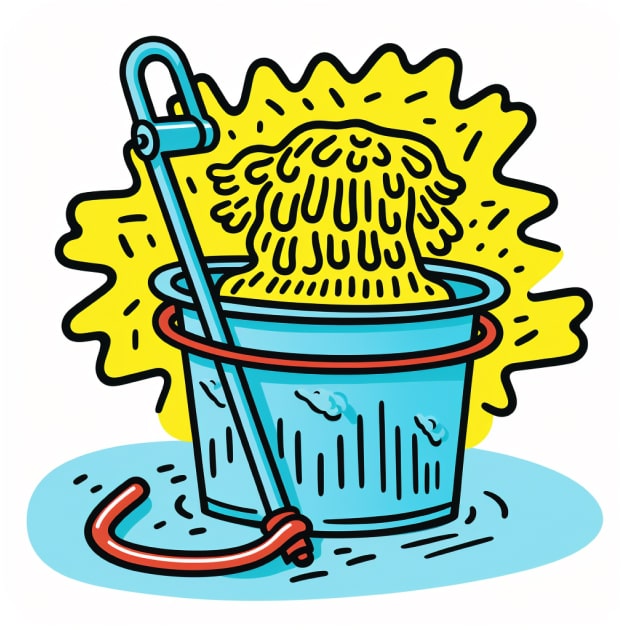
Cluster
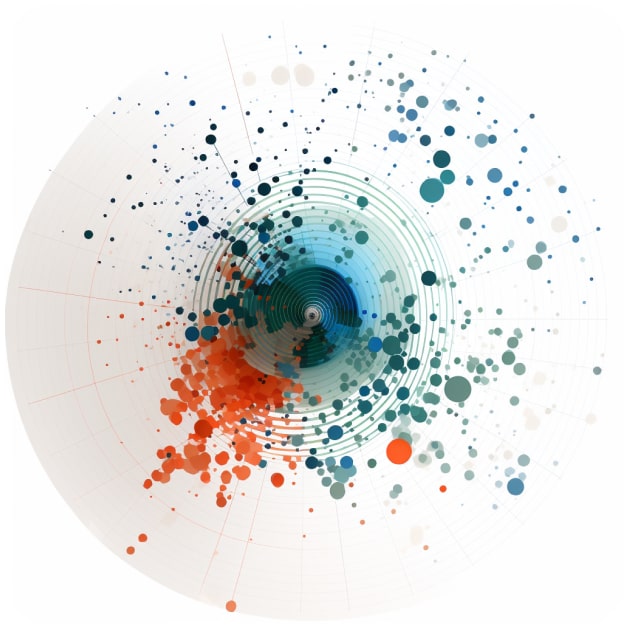
Curate
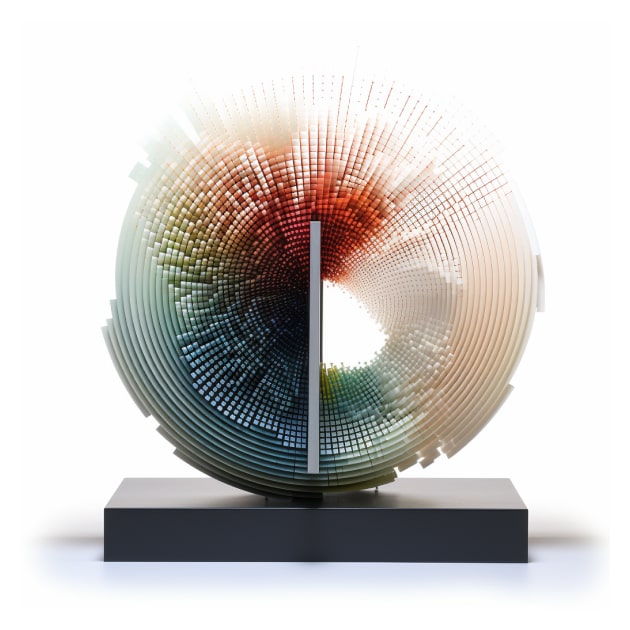
Denoise
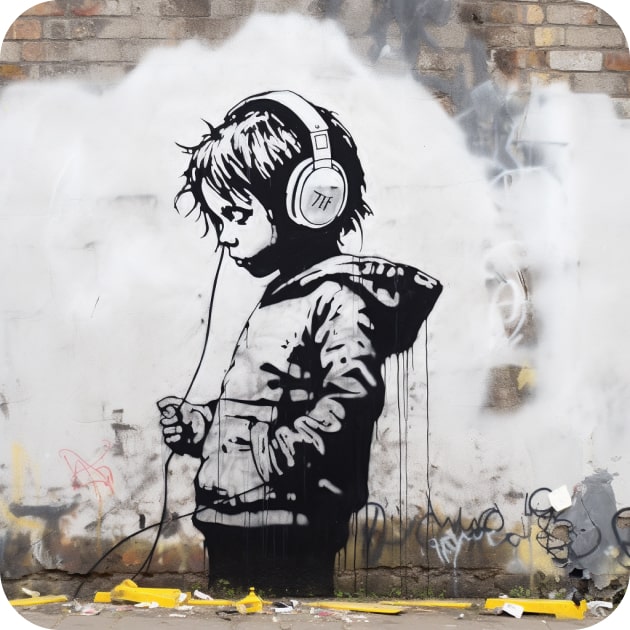
Derive
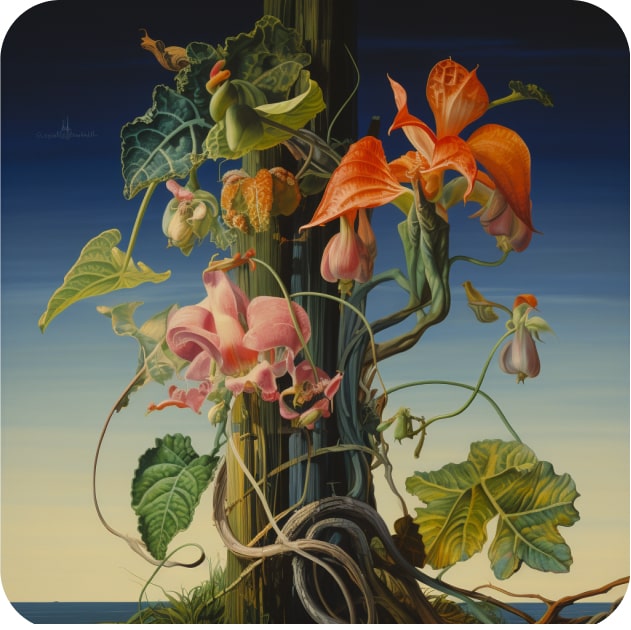
Discretize
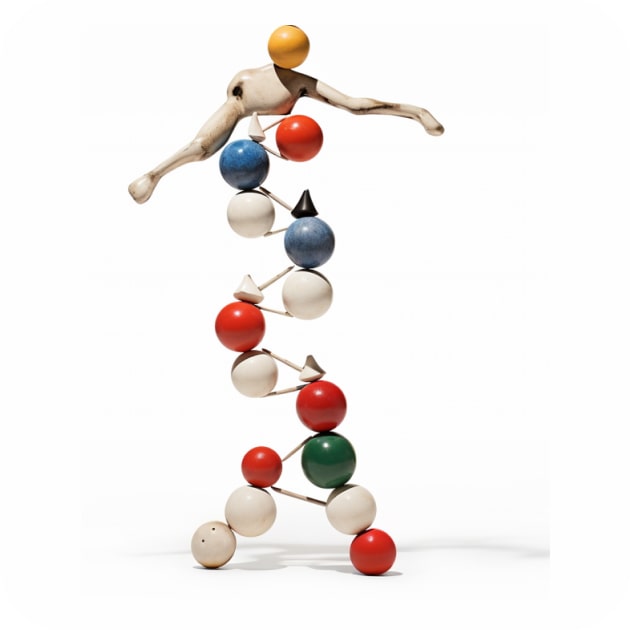
ETL
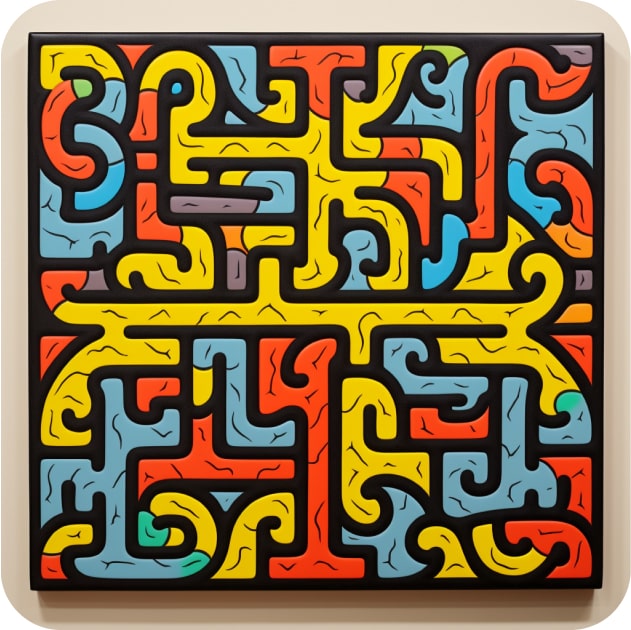
Encode
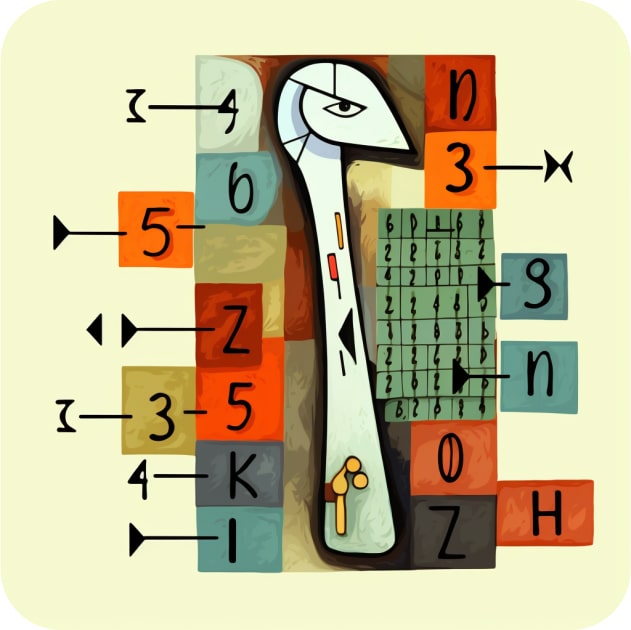
Filter
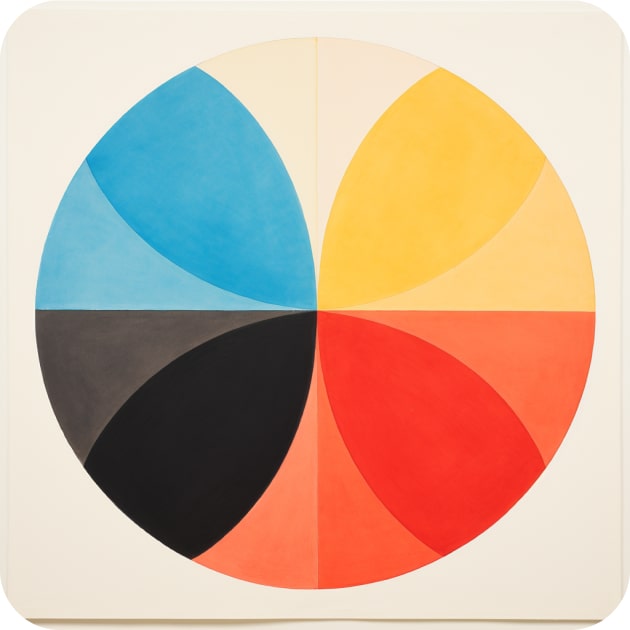
Fragment
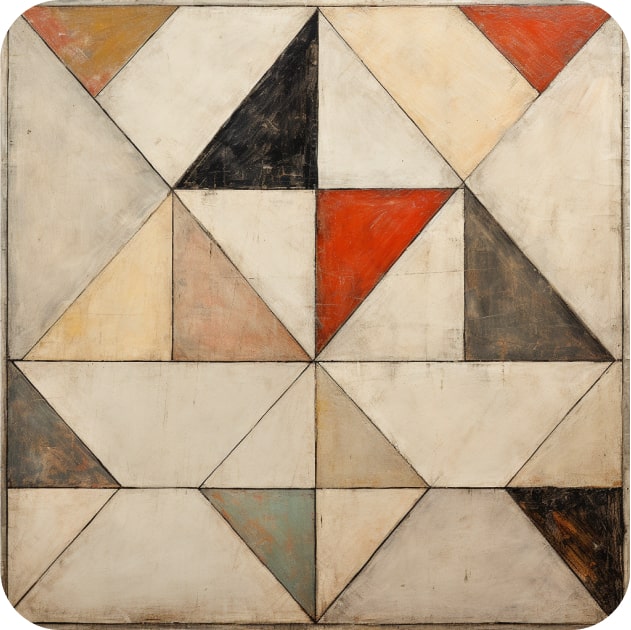
Homogenize
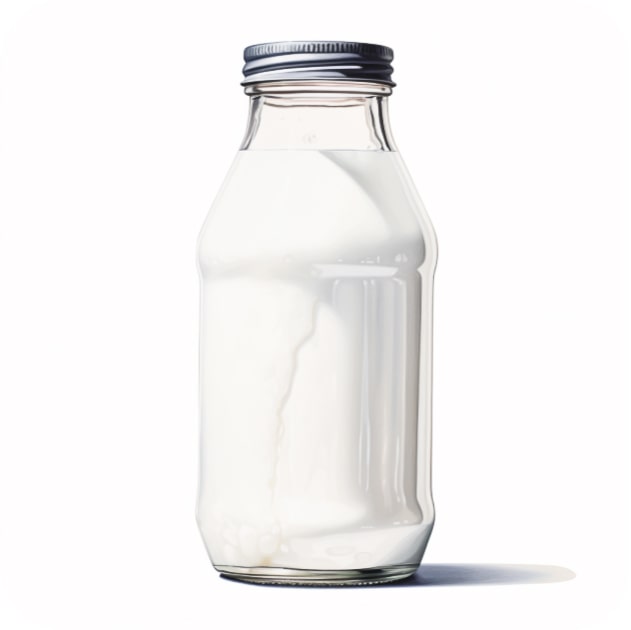
Impute
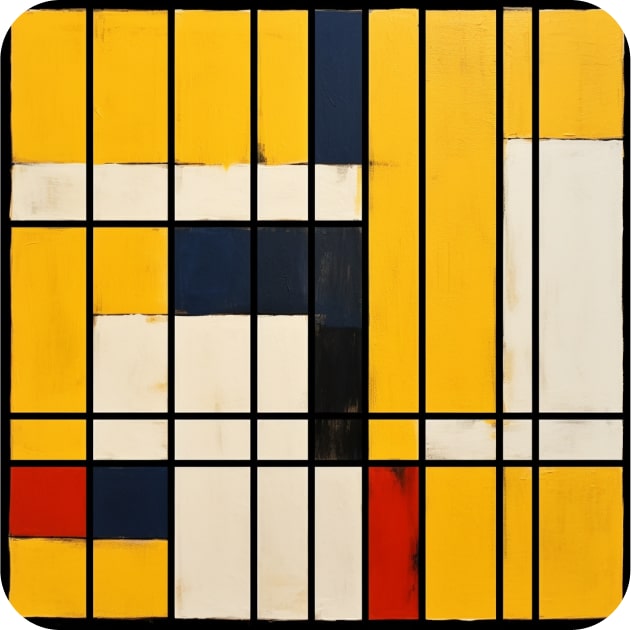
Linearize
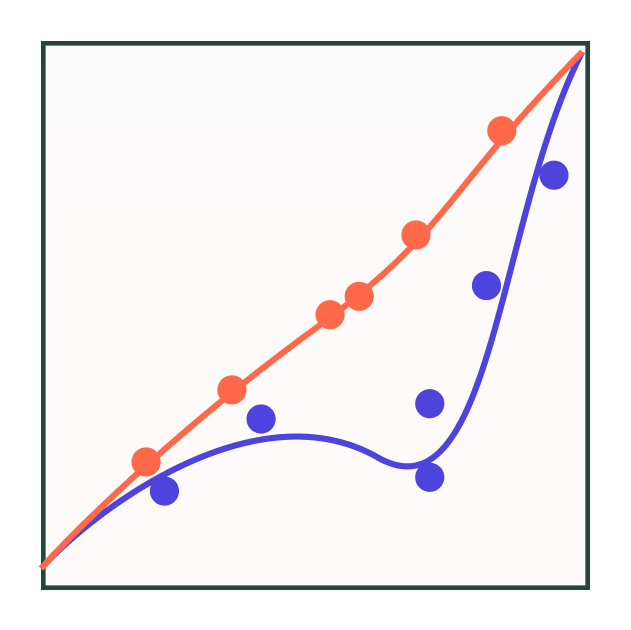
Munge
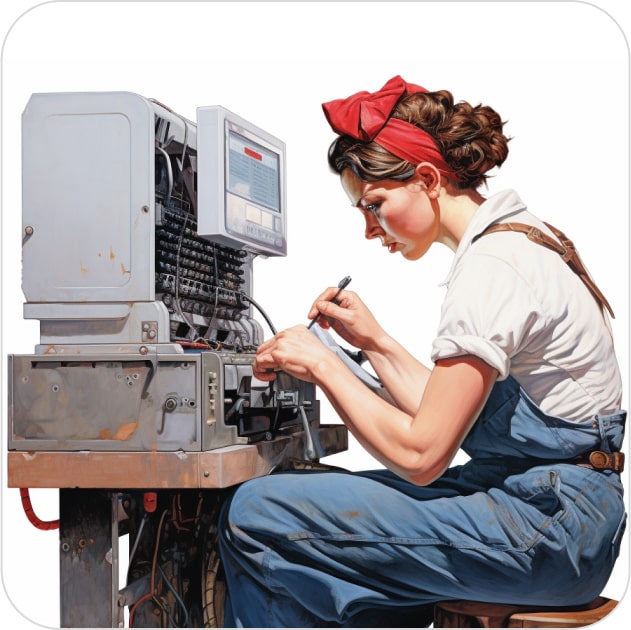
Normalize
Reduce
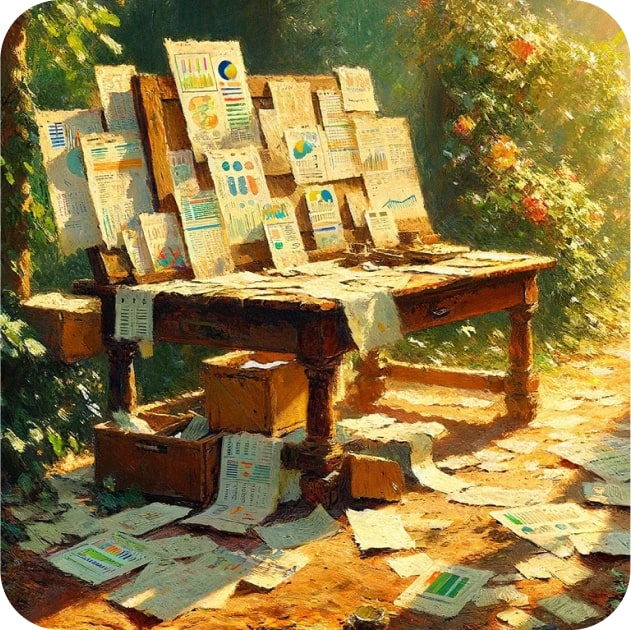
Reshape
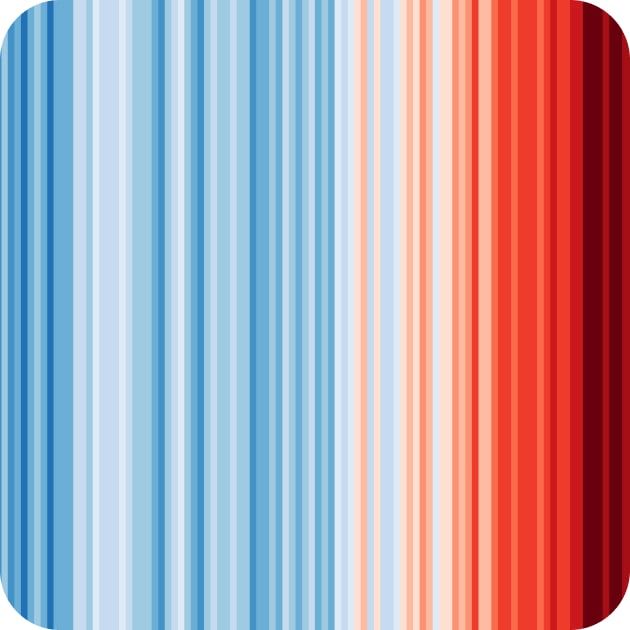
Serialize
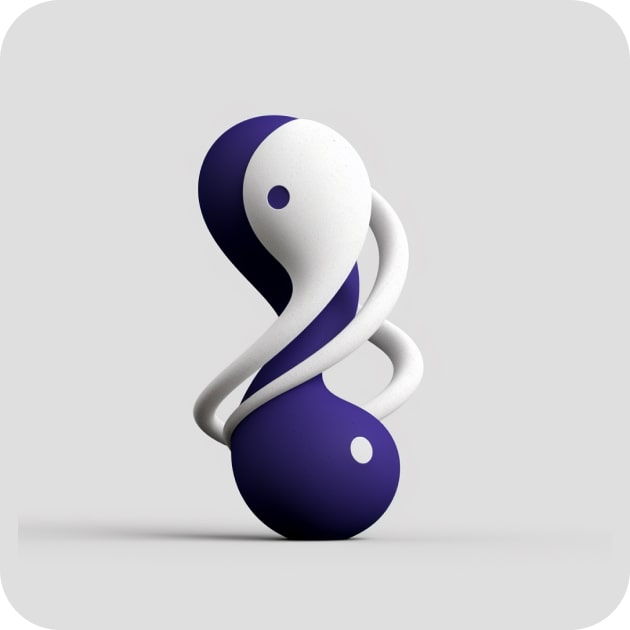
Shred
Skew
Split
Standardize
Tokenize
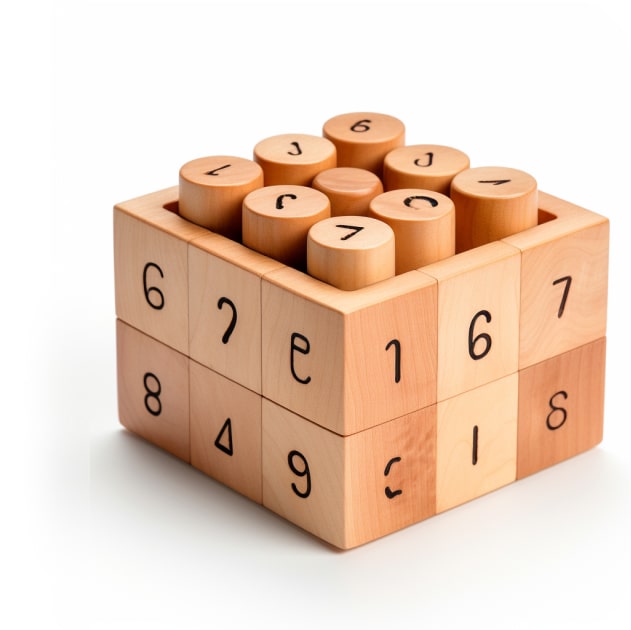
Transform
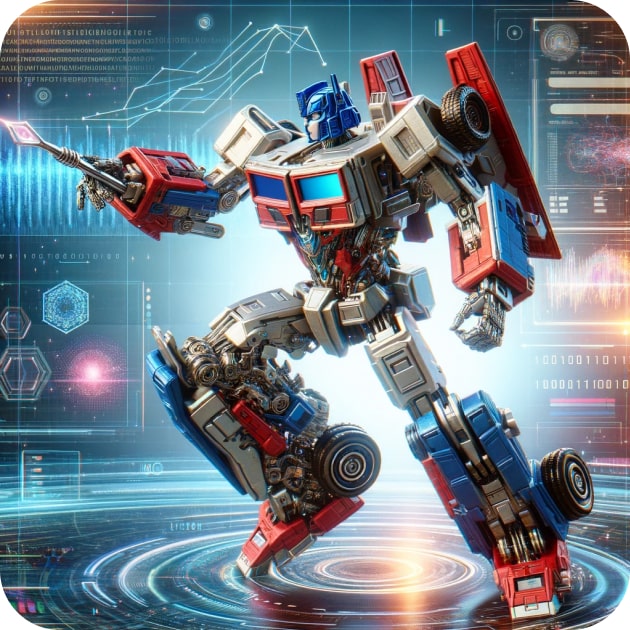
Wrangle
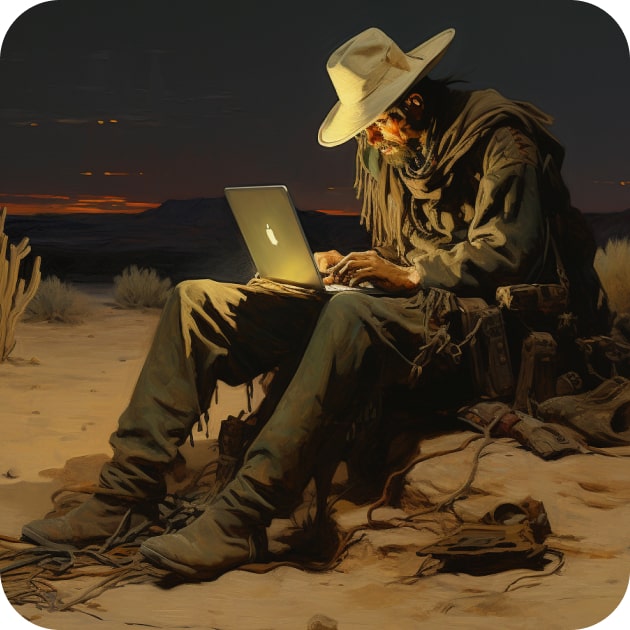