Dagster Data Engineering Glossary:
Data Export
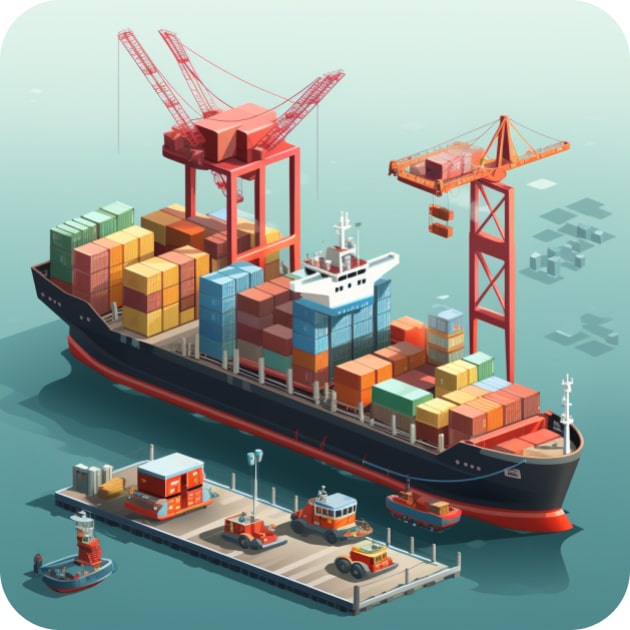
Data export definition:
Data export involves moving data from one system to another, often for sharing or analysis purposes. Exporting data involves converting the data into a specific format that can be easily imported by the target system. This can involve various steps such as selecting the relevant data, cleaning and transforming it, and formatting it for export.
There are several techniques for data export when designing your pipelines, depending on the requirements and constraints of the system. Some common techniques include:
- Writing data to a file: This is a simple and widely used technique where data is written to a file in a specific format (e.g., CSV, JSON, Parquet). The file can then be used by downstream systems or loaded into a database.
- Exporting data to a database: This involves exporting data directly to a database, where it can be used by downstream systems. The database can be a traditional relational database or a NoSQL database, depending on the data and use case.
- Publishing data to a messaging system: In this technique, data is published to a messaging system like Apache Kafka or RabbitMQ. This allows other systems to consume the data in real-time.
- Streaming data to a cloud-based data warehouse: This technique involves streaming data directly to a cloud-based data warehouse like Amazon Redshift or Google BigQuery. This allows for real-time analysis and reporting.
In each of these techniques, the data is typically transformed and processed before being exported to ensure that it is in the correct format and contains the required information. Python libraries like pandas, pyodbc, and sqlalchemy can be used to facilitate data export to various sources.
Data exports in Python
Python provides a variety of libraries and tools to export data in various formats, including CSV, Excel, JSON, and more. Other libraries, such as openpyxl or xlsxwriter, can be used to export data to Excel files, and json library can be used to export data in JSON format.
When managing multiple environments like production, staging, and development in a data pipeline, it's important to have a system in place for exporting data that is appropriate for each environment. Here are some techniques for data export in the context of managing multiple environments:
Parameterization: One approach is to parameterize the data export process so that it can be configured differently for each environment. For example, you might define a set of environment-specific configuration files that specify the output format, file location, and other details for each environment.
Automated deployment: Another technique is to use an automated deployment system that can handle the process of exporting data as part of the overall deployment process. This approach can help ensure that the correct data is exported for each environment and that the process is repeatable and consistent across all environments.
Data archiving: In some cases, it may be necessary to archive data from one environment and import it into another. This might involve exporting data from a production environment and then importing it into a staging or development environment for testing purposes.
Export logging: It's important to keep track of which data has been exported from each environment and when. This can be done using logging tools that record the details of each export operation, including the data exported, the output format, and the destination location.
You can write scripts that automate the data export process, making it easy to manage and export data across multiple environments. Dagster provides functionality for managing data pipelines and automating the export process across different environments.
Dagster provides a main abstraction called iO Managers for separating storage logic for exporting data from your main business logic. Such abstractions can be used to dramatically accelerate your development lifecycle by helping you work efficiently across environments.
Append
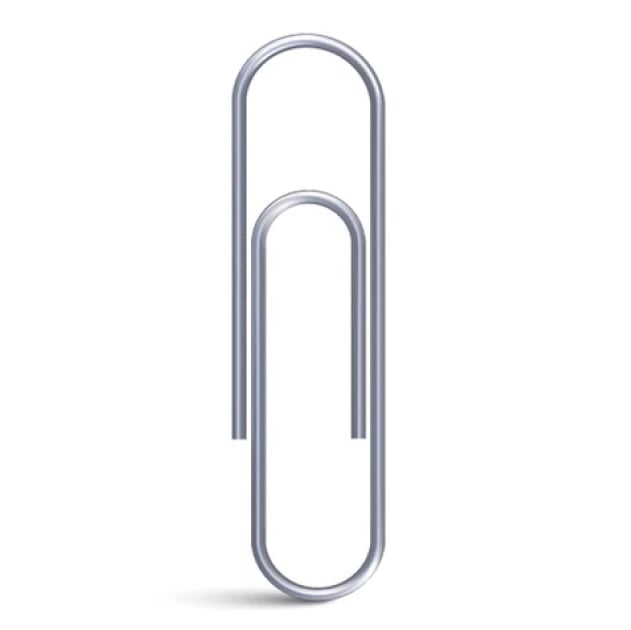
Archive
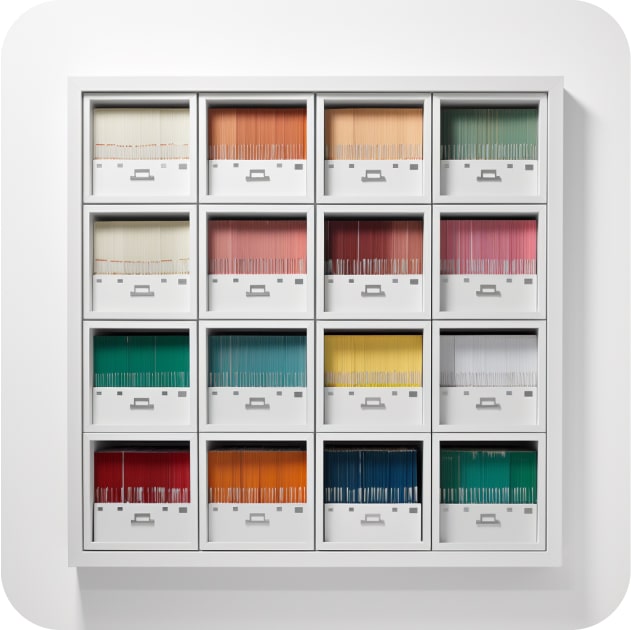
Augment
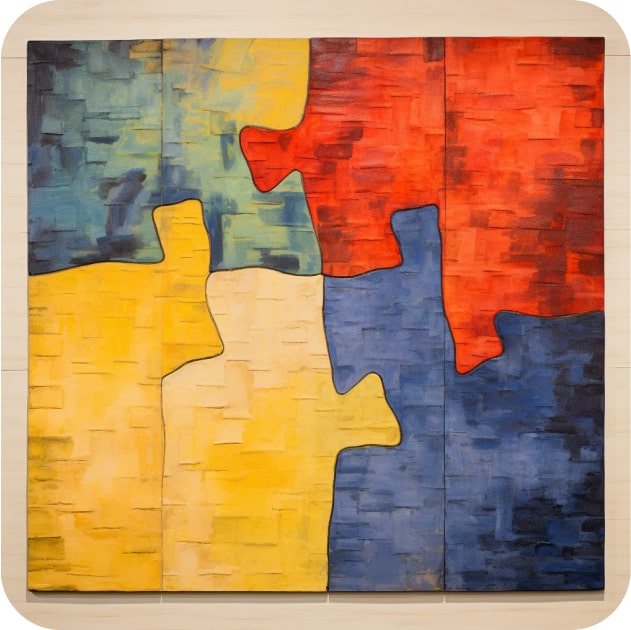
Auto-materialize
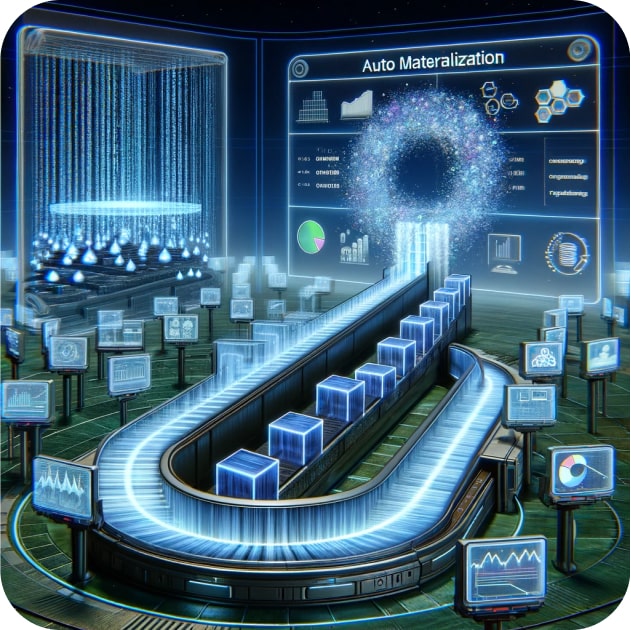
Backup
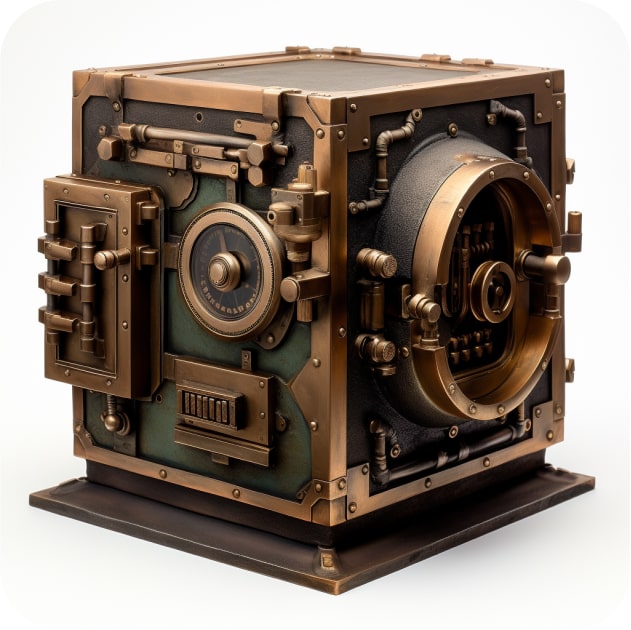
Batch Processing
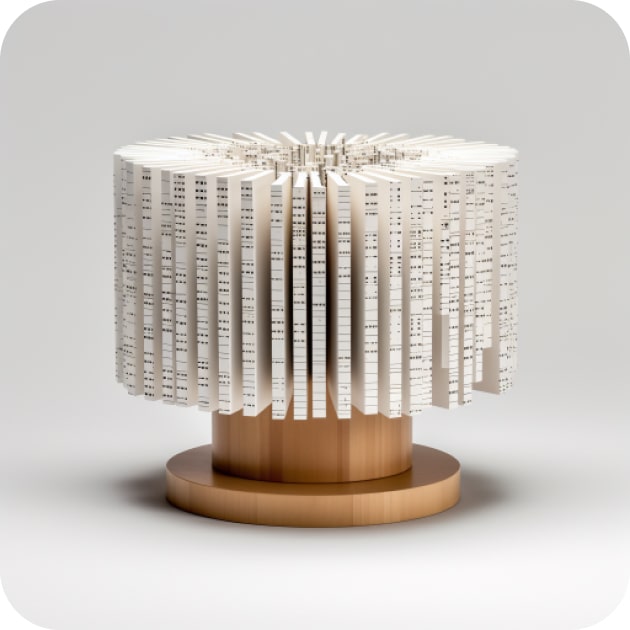
Cache
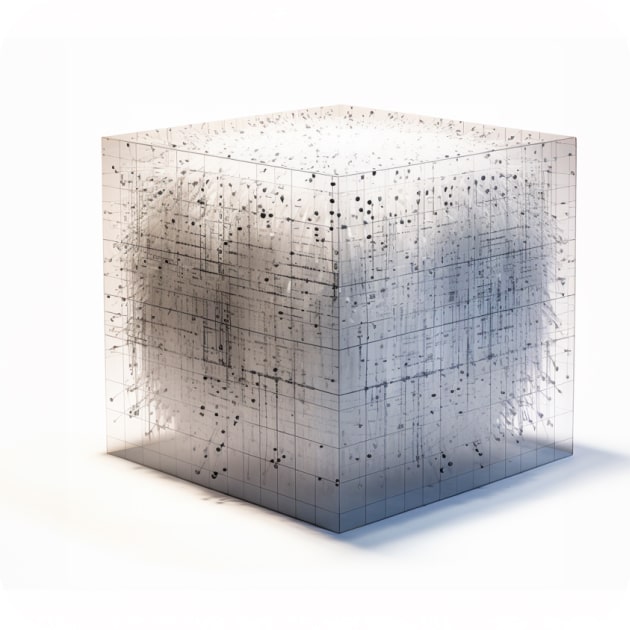
Categorize
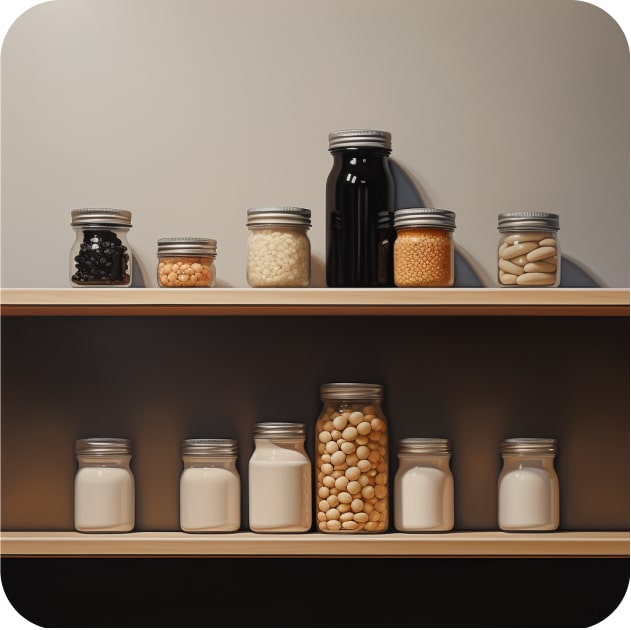
Checkpointing
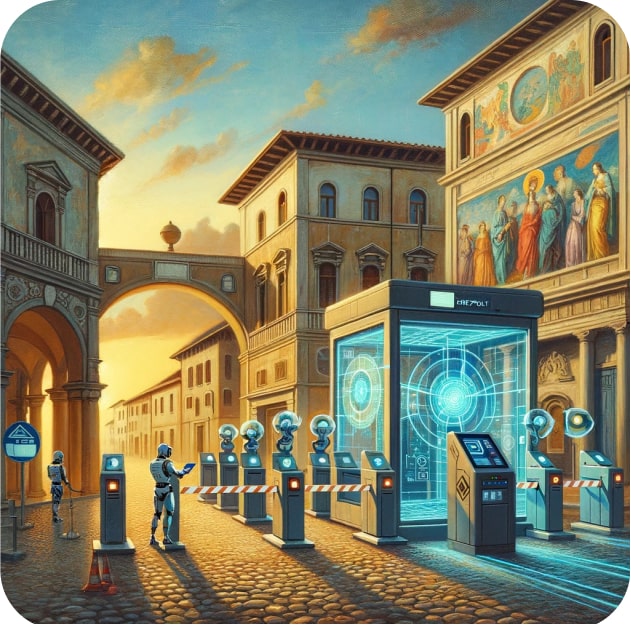
Deduplicate
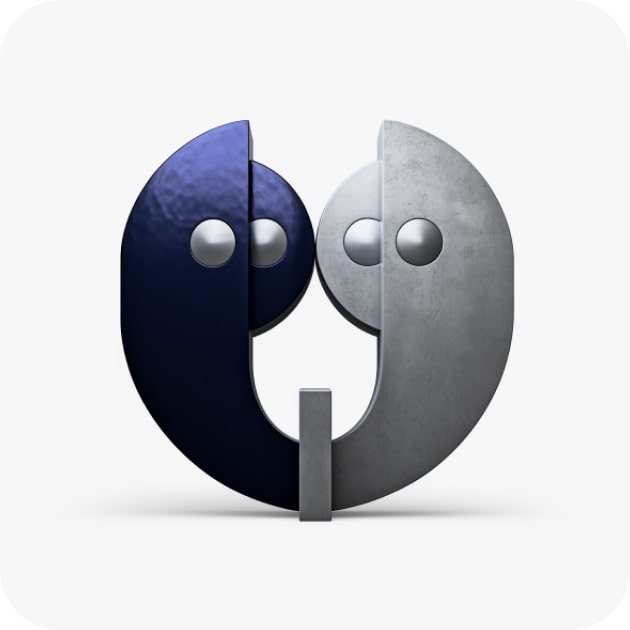
Deserialize
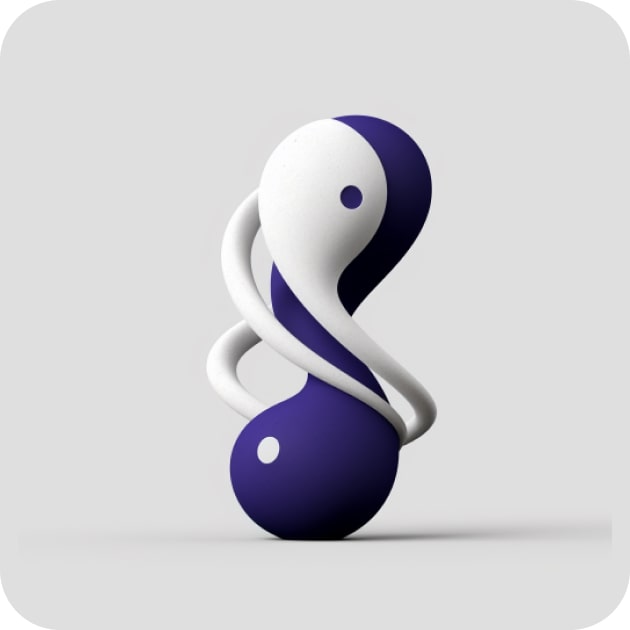
Dimensionality
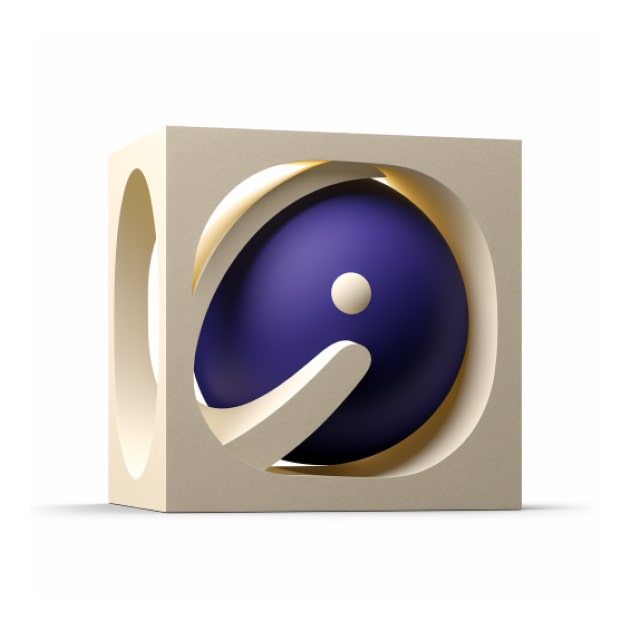
Encapsulate
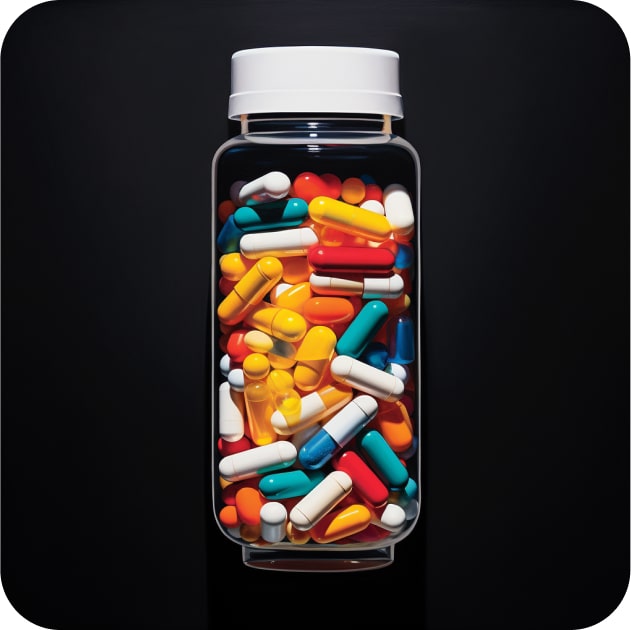
Enrich
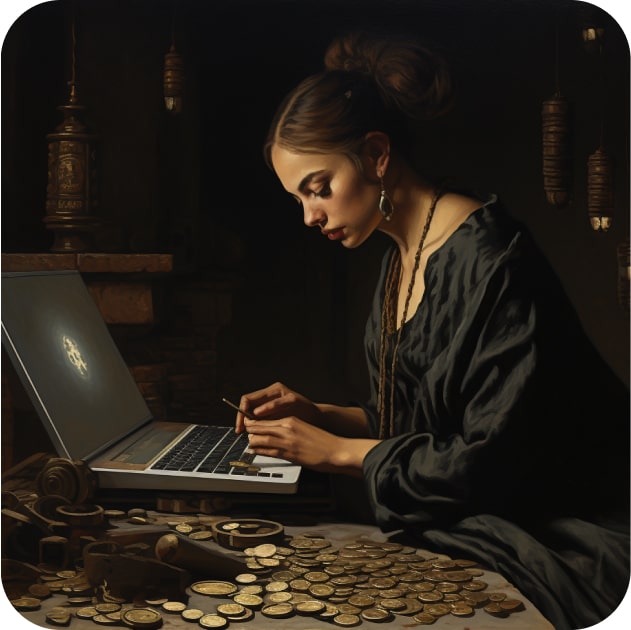
Graph Theory
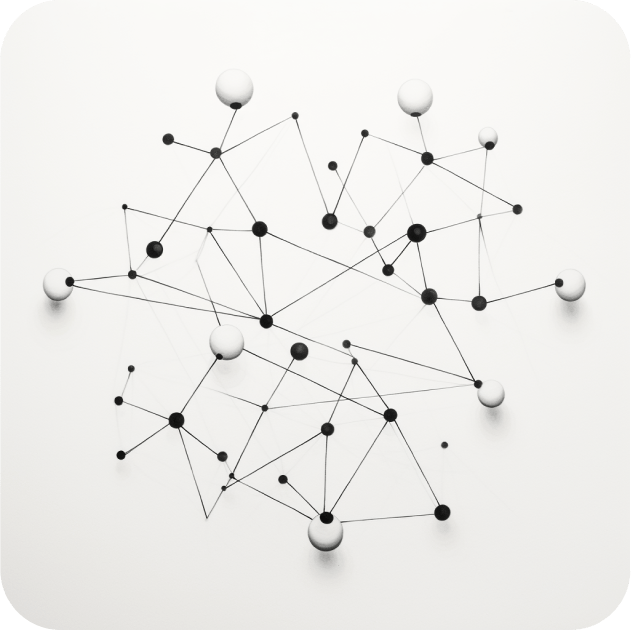
Idempotent
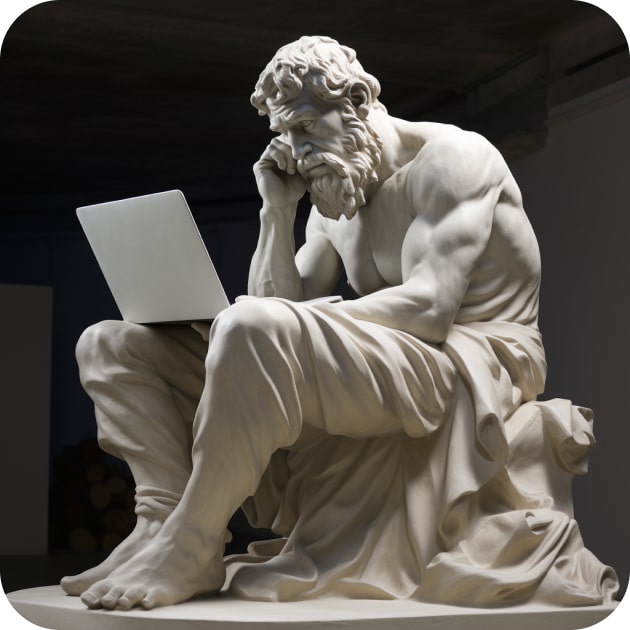
Index
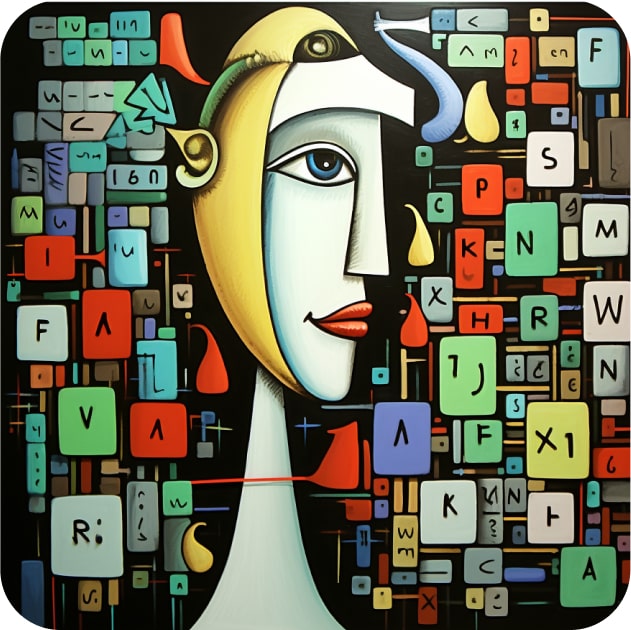
Integrate
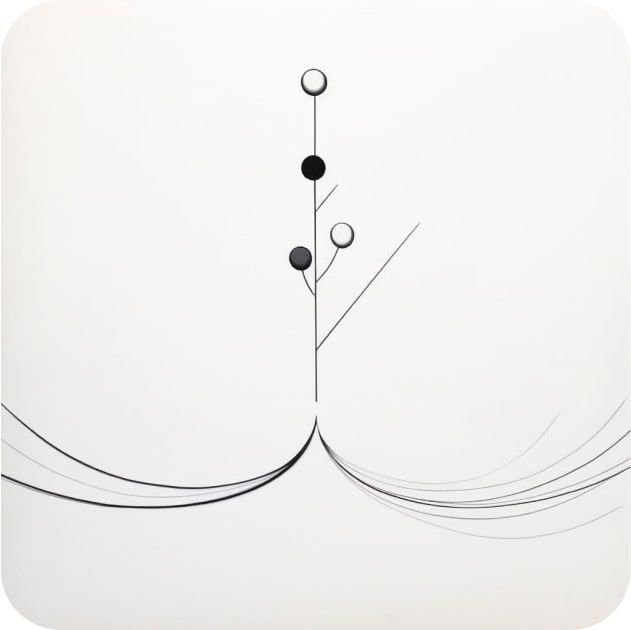
Lineage
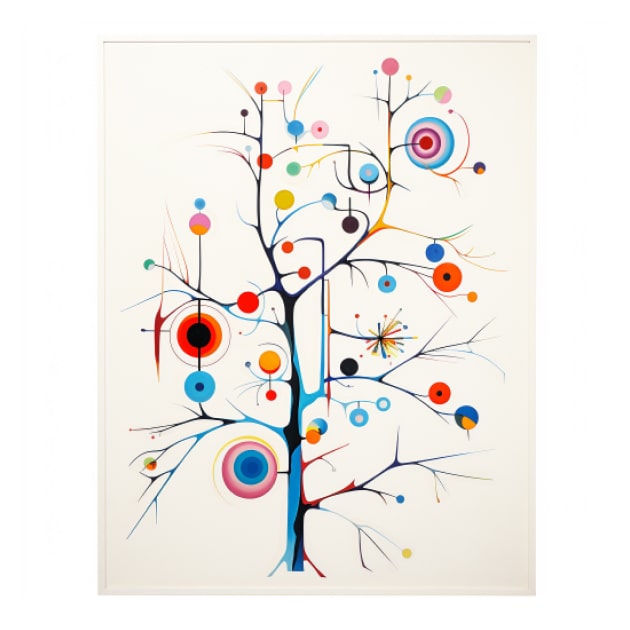
Linearizability
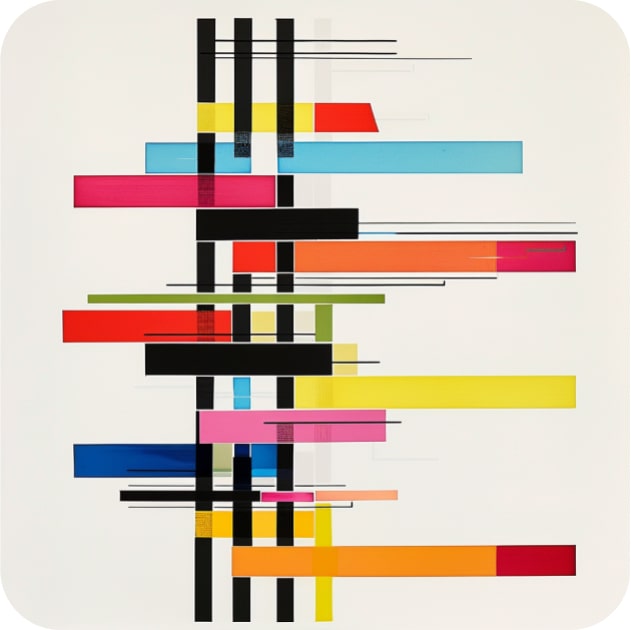
Materialize
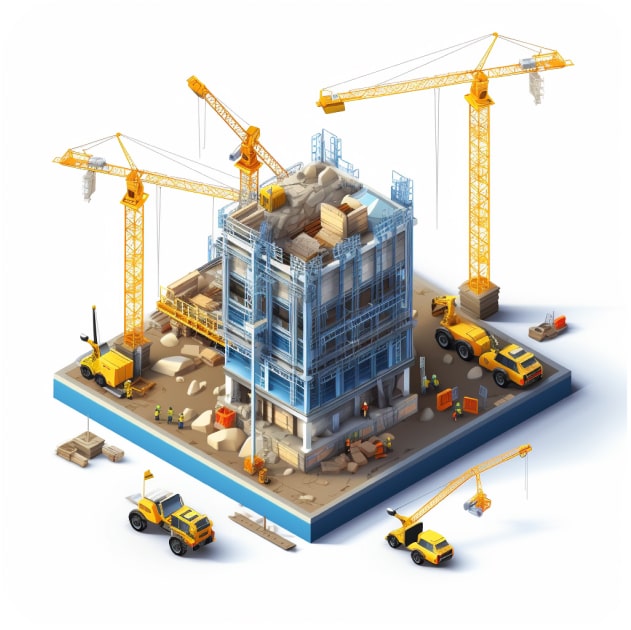
Memoize
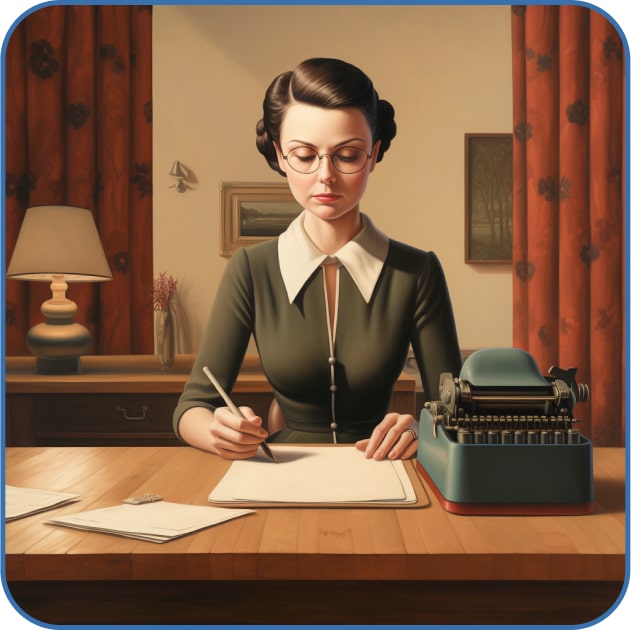
Merge
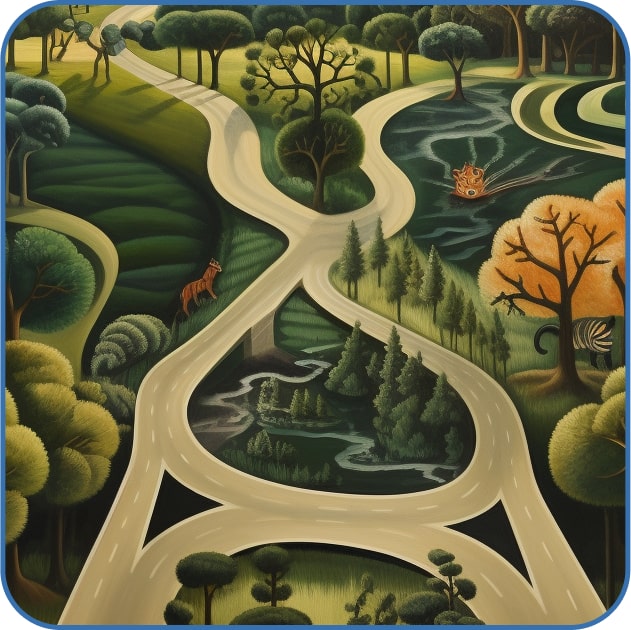
Model
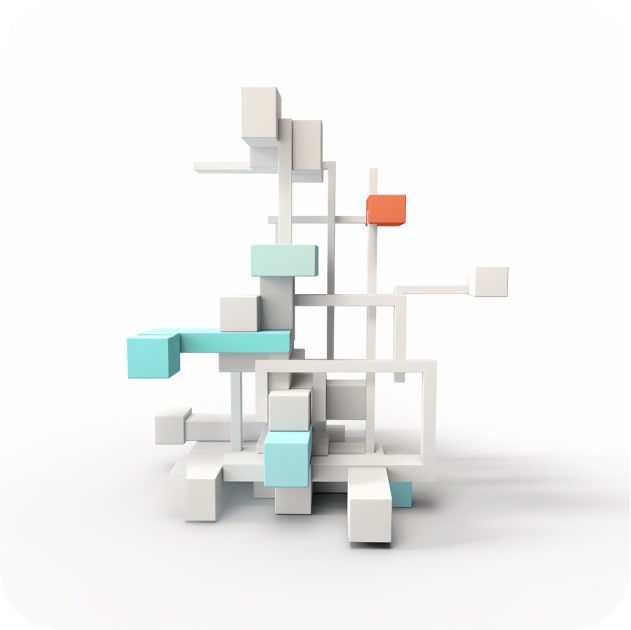
Monitor
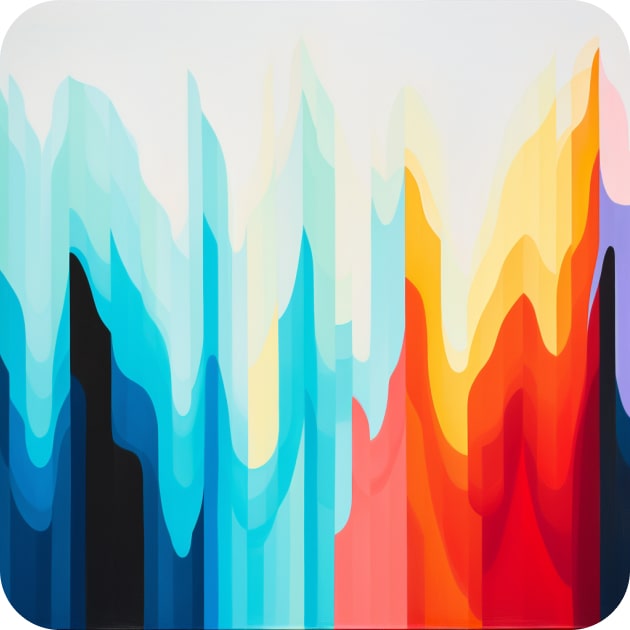
Named Entity Recognition
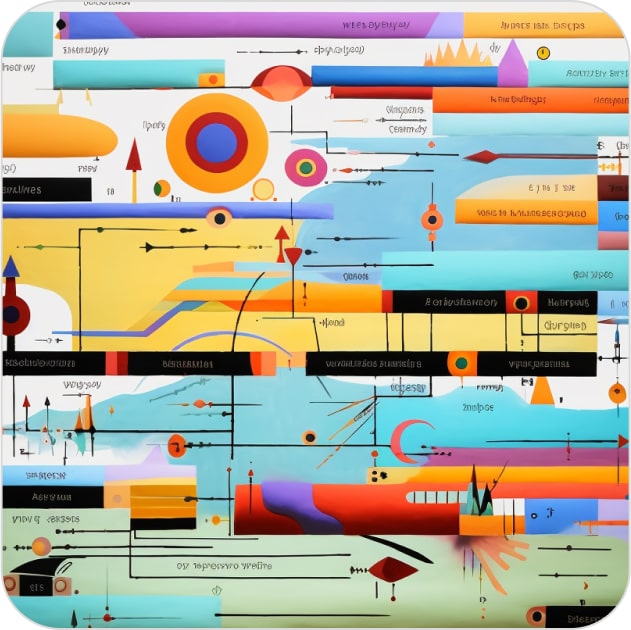
Parse
Partition
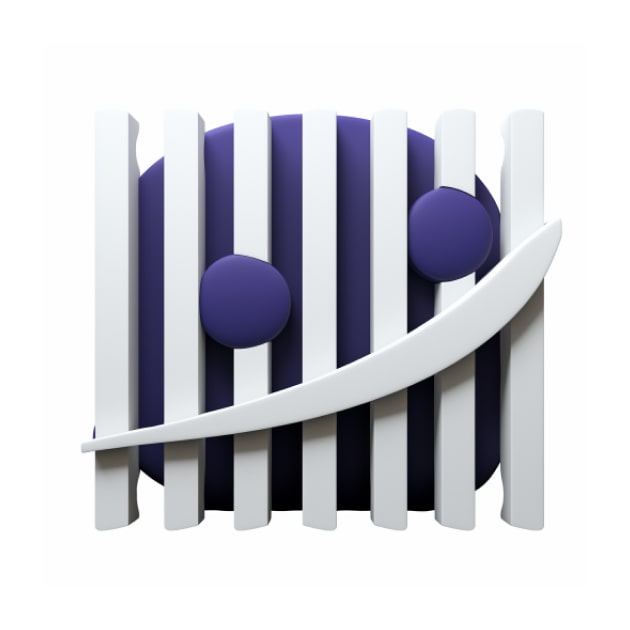
Prep
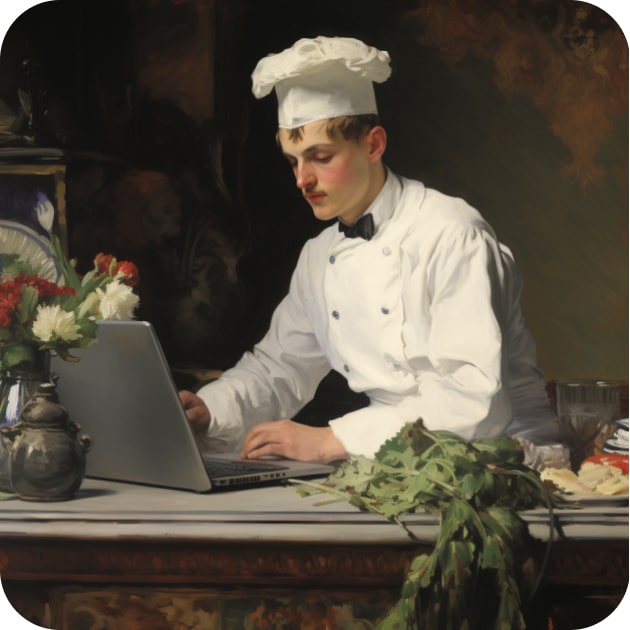
Preprocess
Primary Key
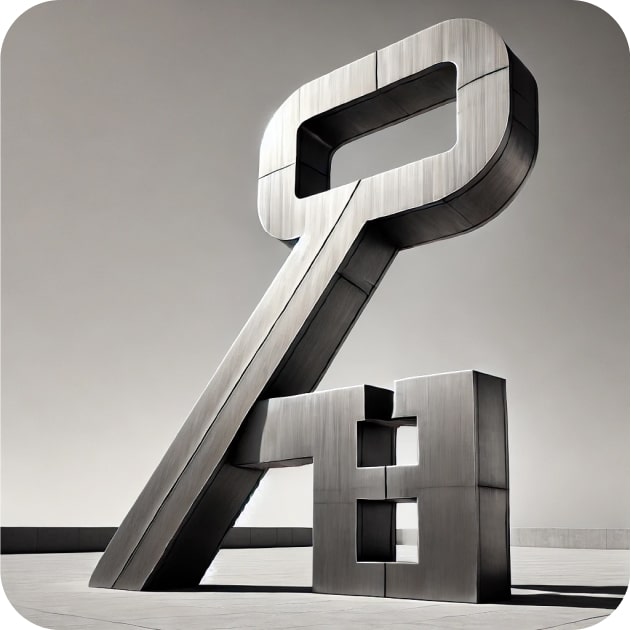
Replicate
Scaling
Schema Inference
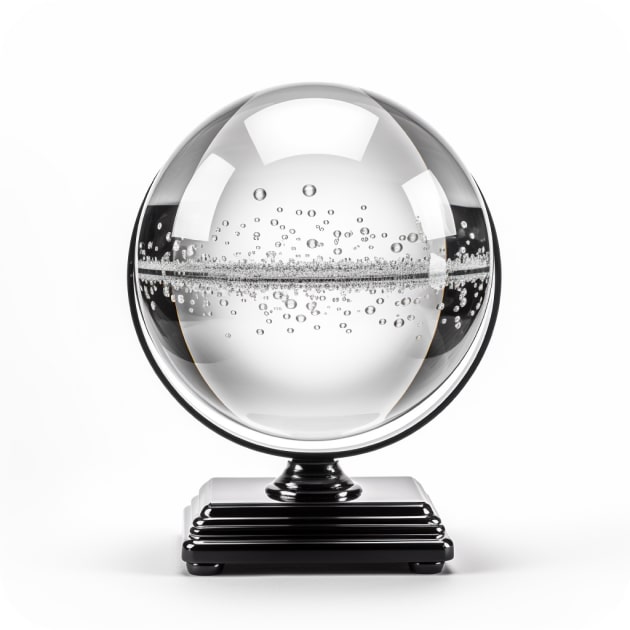
Schema Mapping
Secondary Index
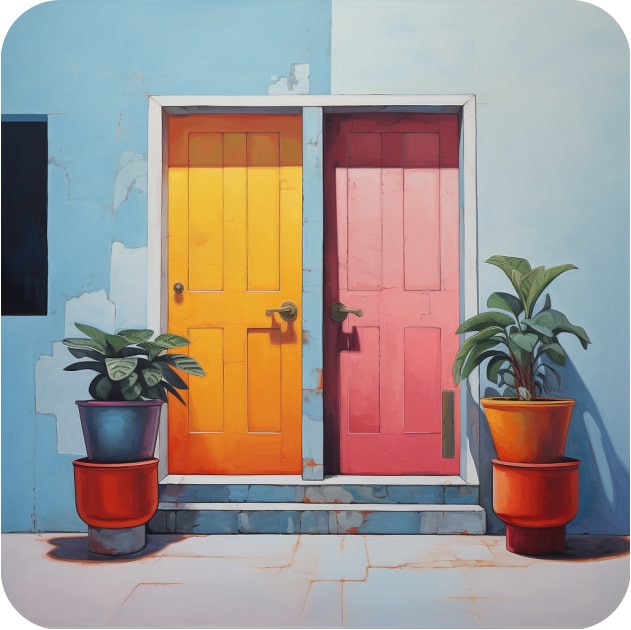
Software-defined Asset
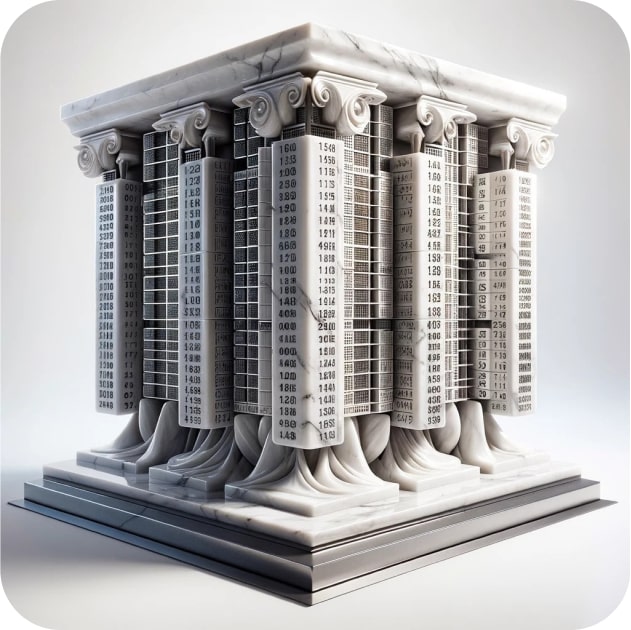
Synchronize
Validate
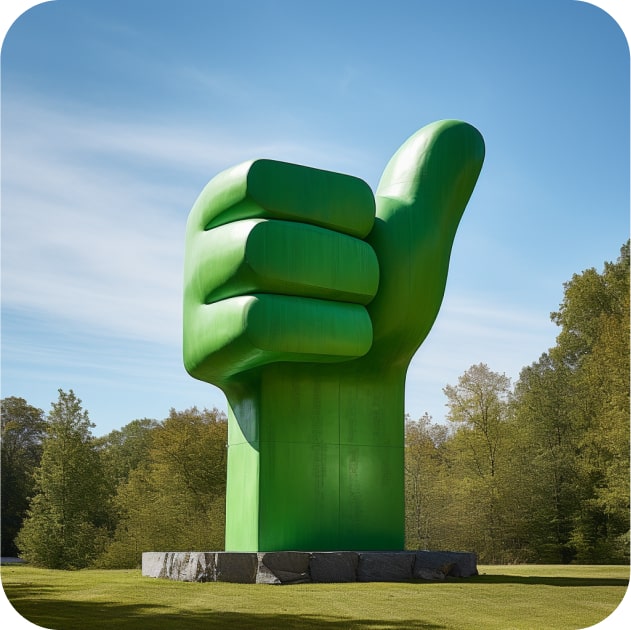
Version
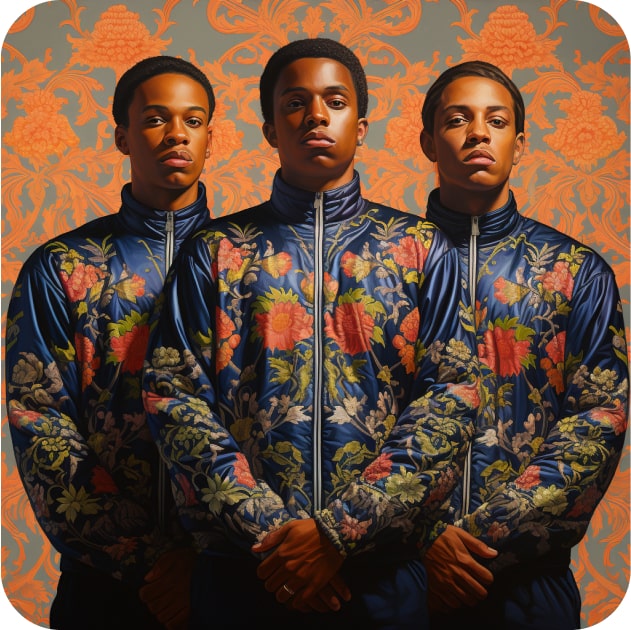