Data Normalization
Data normalization definition:
Data normalization is the process of transforming data into a common scale. It is an important technique in data processing, as it ensures that different features of the data contribute equally to the analysis. Data normalization can be achieved through several techniques, such as Min-Max scaling, Z-score normalization, and Robust scaling.
Normalization is achieved through a set of rules known as normal forms. The most commonly used normal forms are first,second and third normal form (1NF,2NF,3NF), and Boyce-Codd normal form (BCNF).
Data normalization example using Python:
Please note that you need to have the necessary Python libraries installed in your Python environment to run the following code examples.
Lets look at two examples of data normalization using Python:
Suppose we have a dataset of customers' orders with the following columns: customer ID, order ID, order date, product name, and quantity. We can normalize this data by separating it into two tables: customers and orders. The customers table would have columns for customer ID, customer name, and customer address. The orders table would have columns for order ID, customer ID (which would be a foreign key referencing the customer ID column in the customers table), order date, product name, and quantity.
Here's the code to normalize this data:
import pandas as pd
# create a dataframe with the original data
data = {'customer_id': [1, 1, 2, 3, 3],
'order_id': [1001, 1002, 1003, 1004, 1005],
'order_date': ['2021-01-01', '2021-01-02', '2021-01-03', '2021-01-04', '2021-01-05'],
'product_name': ['product1', 'product2', 'product3', 'product1', 'product2'],
'quantity': [2, 1, 3, 1, 2]}
df = pd.DataFrame(data)
# create the customers table by dropping duplicates and resetting the index
customers = df[['customer_id']].drop_duplicates().reset_index(drop=True)
# add columns for customer name and address
customers['customer_name'] = ['John', 'Samantha', 'Peter']
customers['customer_address'] = ['123 Main St', '456 Maple Ave', '789 Oak Rd']
# create the orders table by merging the original dataframe with the customers table
orders = pd.merge(df, customers, on='customer_id')
# drop the customer ID column from the orders table
orders = orders.drop(columns=['customer_id'])
# print the resulting tables
print(customers)
print(orders)
This code will output the following tables:
customer_id customer_name customer_address
0 1 John 123 Main St
1 2 Samantha 456 Maple Ave
2 3 Peter 789 Oak Rd
order_id order_date product_name quantity customer_name customer_address
0 1001 2021-01-01 product1 2 John 123 Main St
1 1002 2021-01-02 product2 1 John 123 Main St
2 1003 2021-01-03 product3 3 Samantha 456 Maple Ave
3 1004 2021-01-04 product1 1 Peter 789 Oak Rd
4 1005 2021-01-05 product2 2 Peter 789 Oak Rd
In this example, we normalized the data by creating two tables: one for customers and one for orders.
Here is a second practical example in Python using Min-Max scaling:
from sklearn.preprocessing import MinMaxScaler
import pandas as pd
# create a sample dataframe with two columns
df = pd.DataFrame({'column1': [12, 28, 31, 49], 'column2': [50, 60, 70, 80]})
# create a MinMaxScaler object
scaler = MinMaxScaler()
# fit and transform the data
normalized_data = scaler.fit_transform(df)
# create a new dataframe with the normalized data
df_normalized = pd.DataFrame(normalized_data, columns=df.columns)
print(df_normalized)
Here, we use the MinMaxScaler
object from the sklearn.preprocessing
module to scale the data. We fit the scaler object to the original dataframe and transform the data into a normalized scale. Finally, we create a new dataframe with the normalized data and print it to the console. The output will look like this:
column1 column2
0 0.000000 0.000000
1 0.432432 0.333333
2 0.513514 0.666667
3 1.000000 1.000000
The resulting dataframe will have the same number of rows and columns as the original dataframe, but with values in the range [0, 1].
Best practices for normalizing data include:
- Choosing the appropriate normalization technique: There are various normalization techniques, including min-max normalization, z-score normalization, and log transformation. Choose the appropriate normalization technique based on the data distribution, the intended use case, and the desired outcome.
- Handling missing data: Normalization techniques can be sensitive to missing data. Decide on a strategy to handle missing data, whether it's through imputation or exclusion of missing data points.
- Scaling and centering the data: Normalization often involves scaling and centering the data, so the values are comparable and have the same mean and standard deviation. Use Python libraries like Scikit-learn or Pandas to perform scaling and centering.
- Evaluating the effect on the data distribution: Normalization can change the distribution of the data, which can impact downstream analysis. Evaluate the effect of normalization on the data distribution to ensure that it aligns with the intended outcome.
- Addressing outliers: Outliers can affect the normalization process and the results of the analysis. Consider removing or transforming outliers before normalization or using a normalization technique that is robust to outliers.
- Ensuring the normalization is reversible: The normalization process should be reversible to ensure that the data can be reconstructed in its original form if needed. Ensure that the normalization method used can be easily reversed.
- Testing and validating the normalization process: It's essential to test and validate the normalization process to ensure that it's producing the desired outcome. Use automated testing and validation techniques to ensure that the normalization is accurate and consistent.
Align
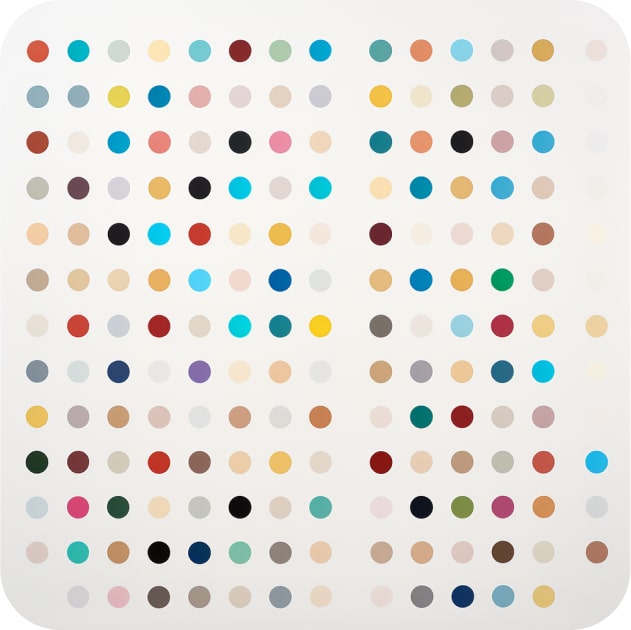
Clean or Cleanse
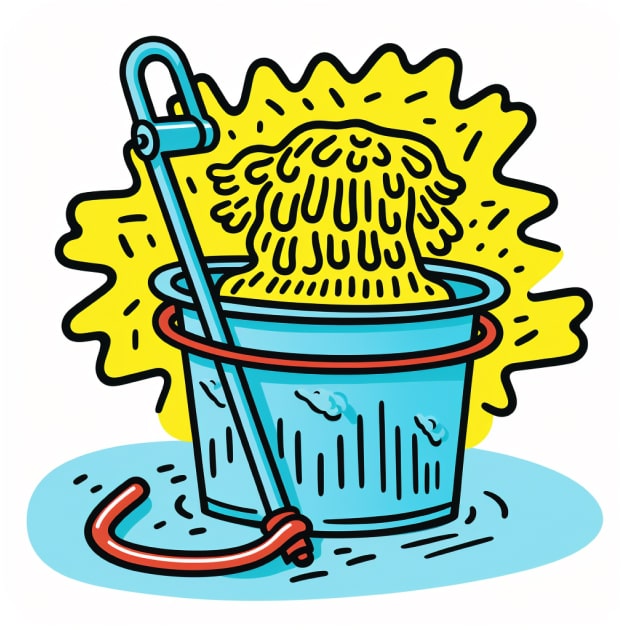
Cluster
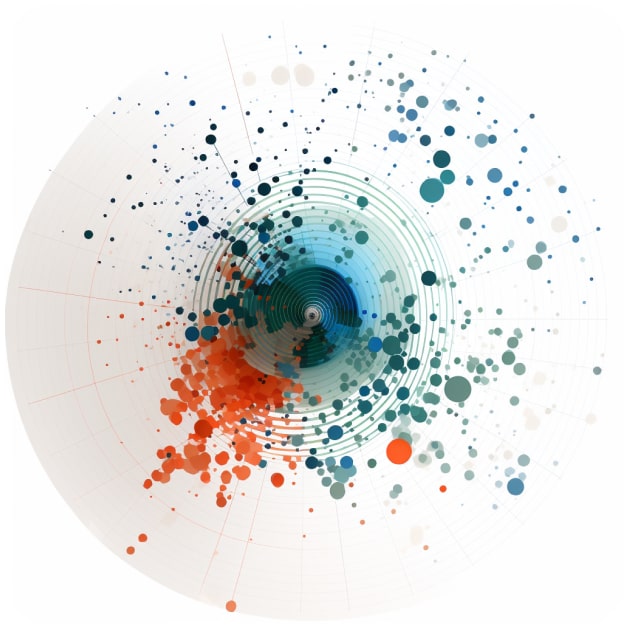
Curate
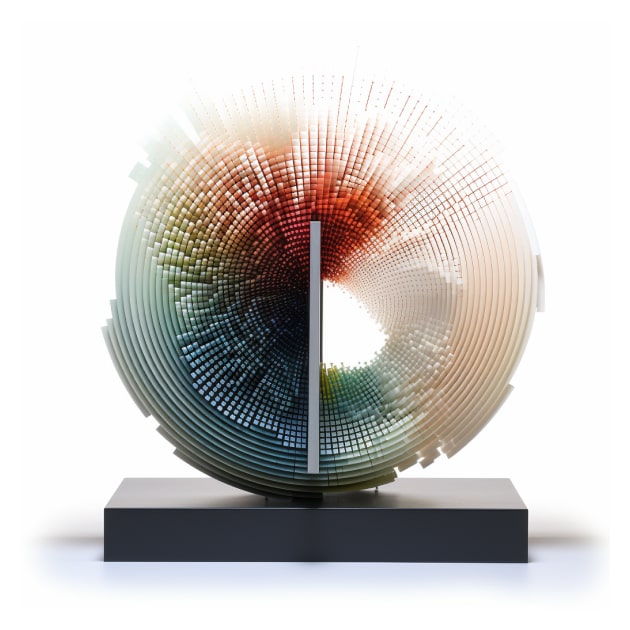
Denoise
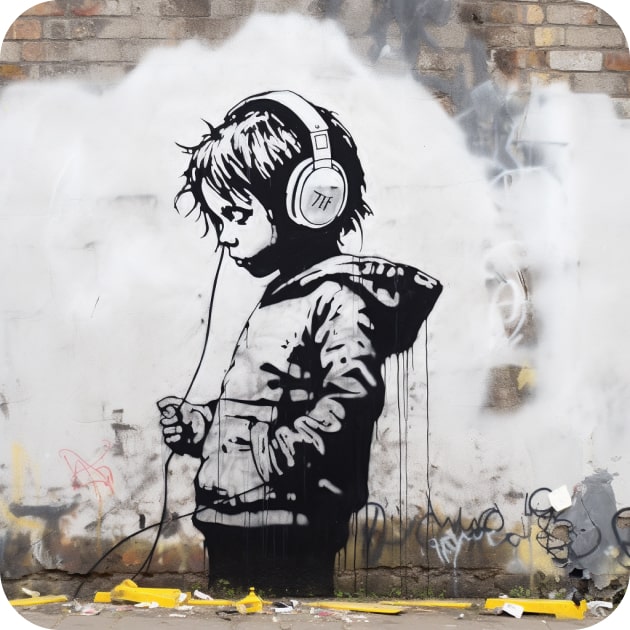
Denormalize
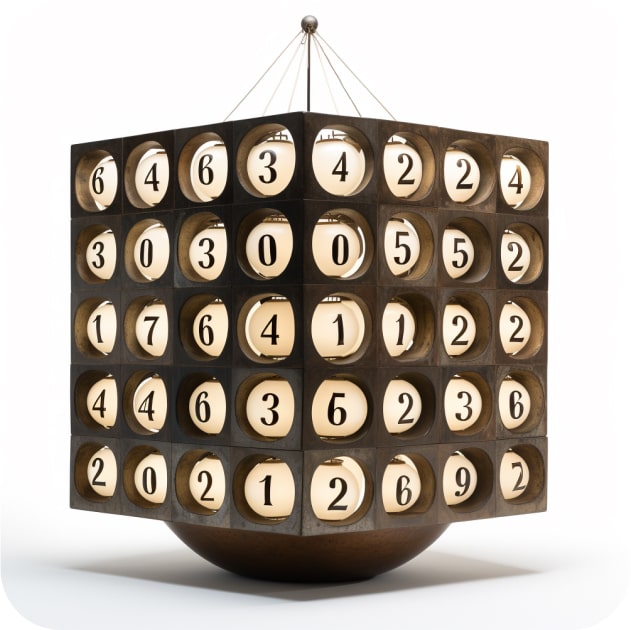
Derive
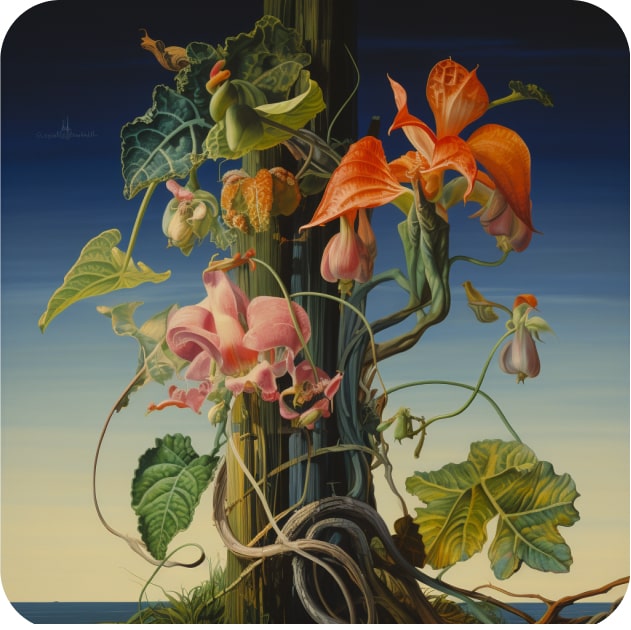
Discretize
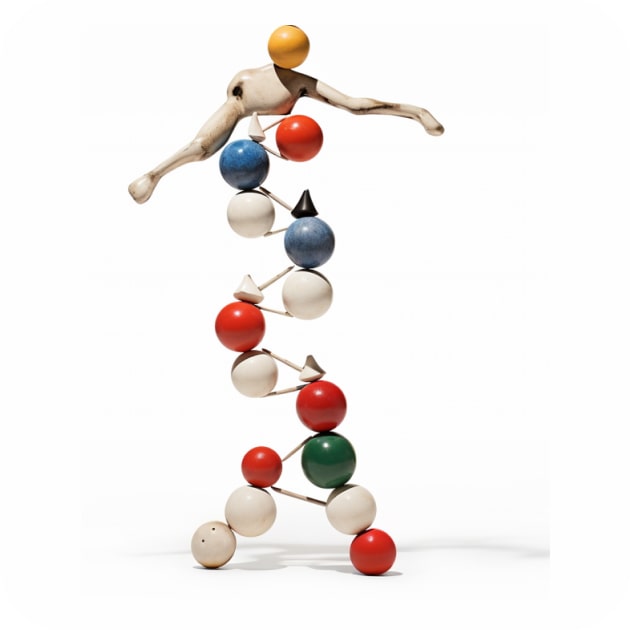
ETL
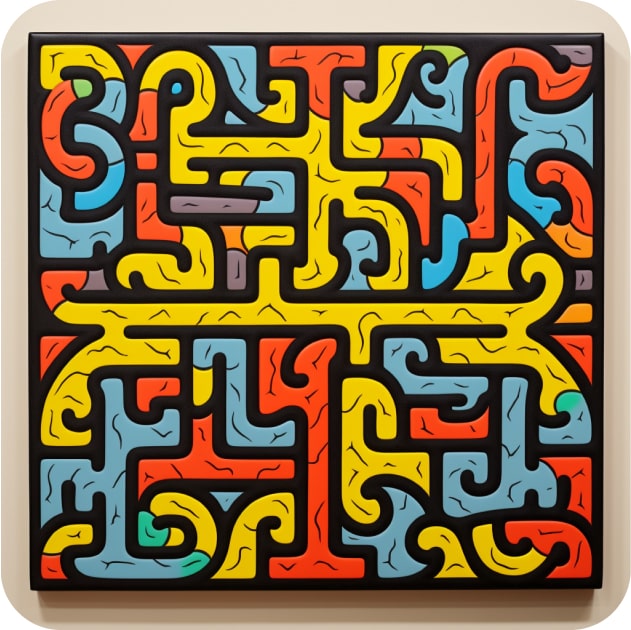
Encode
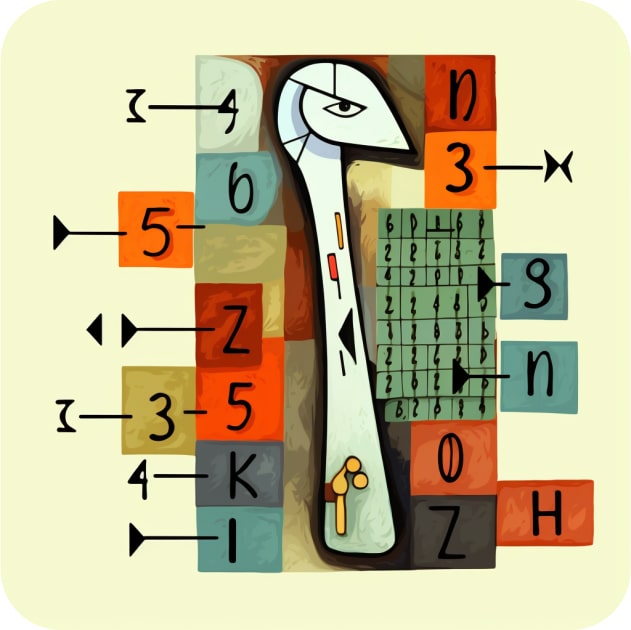
Filter
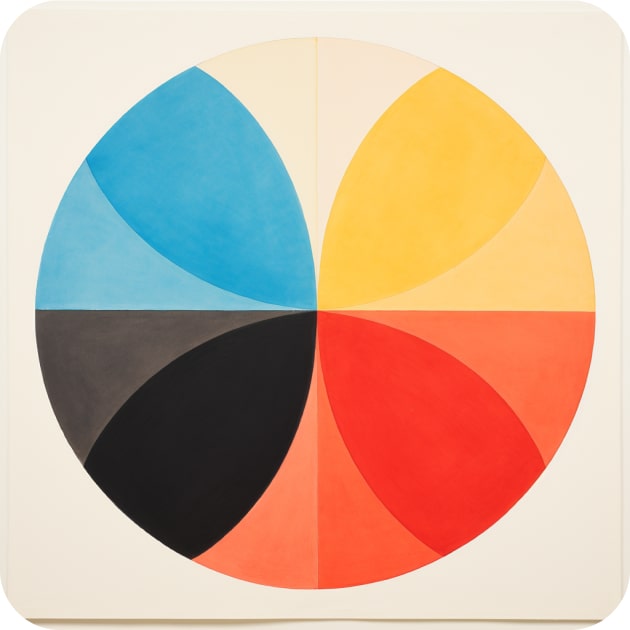
Fragment
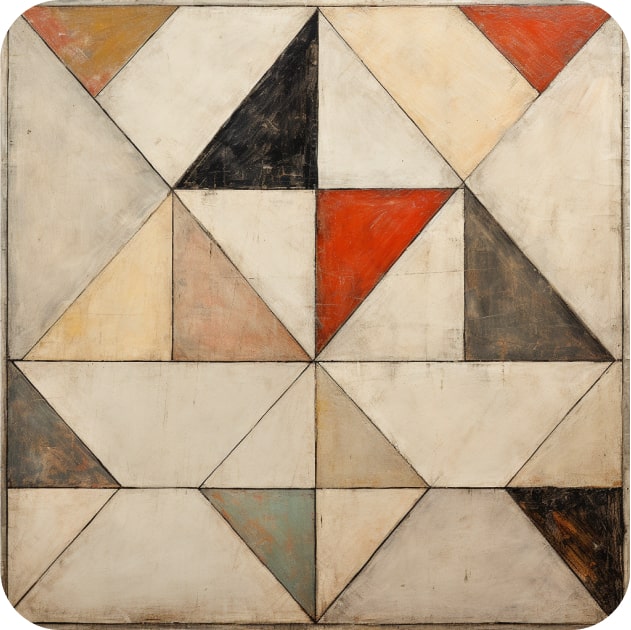
Homogenize
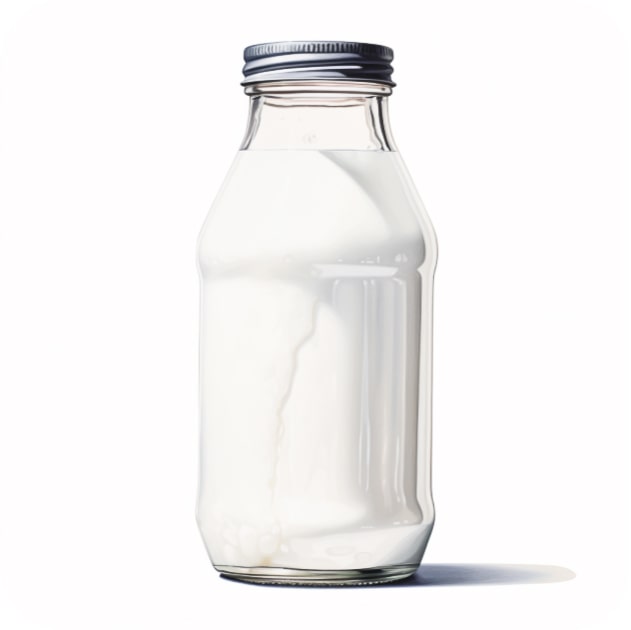
Impute
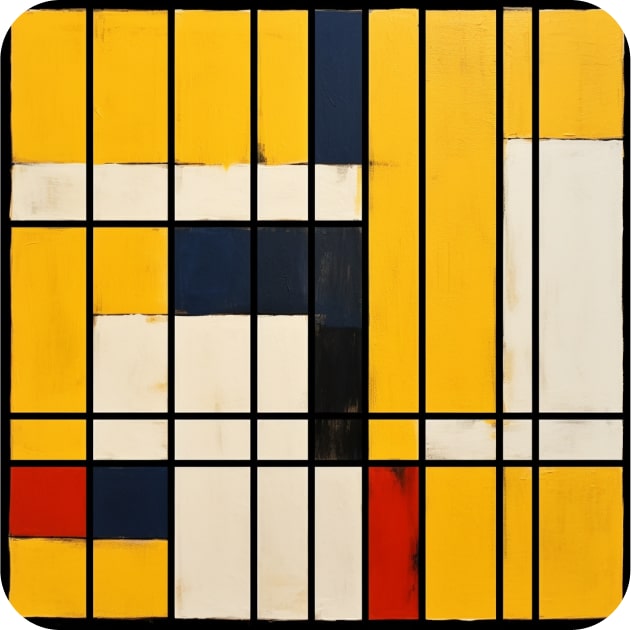
Linearize
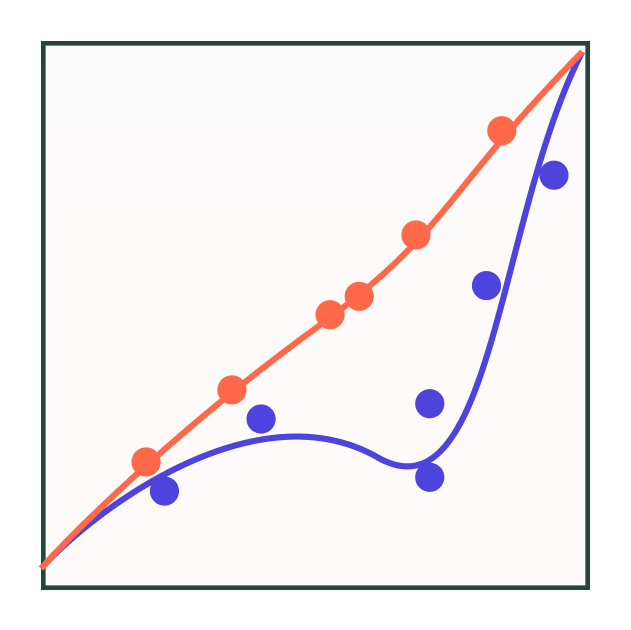
Munge
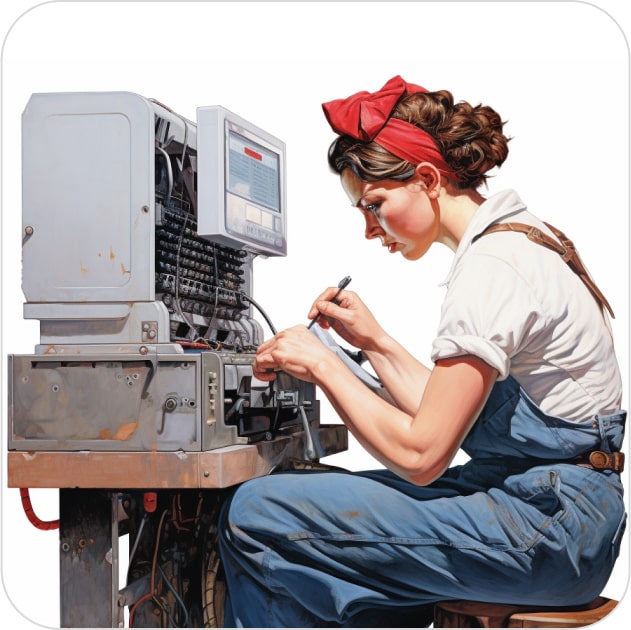
Reduce
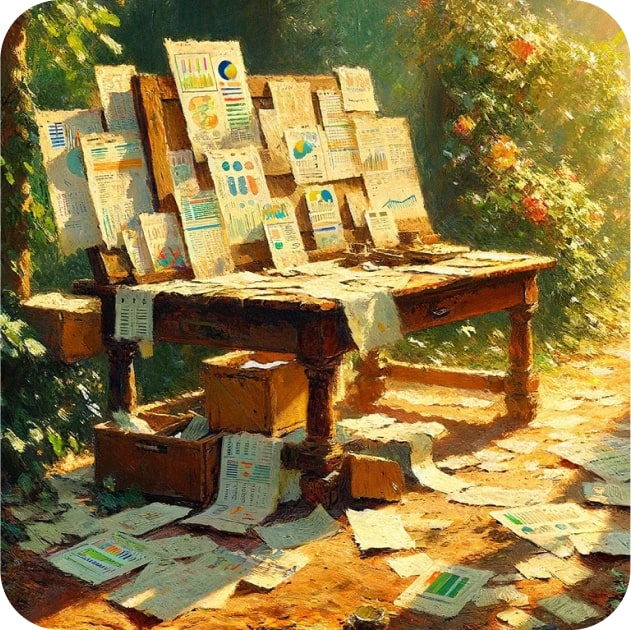
Reshape
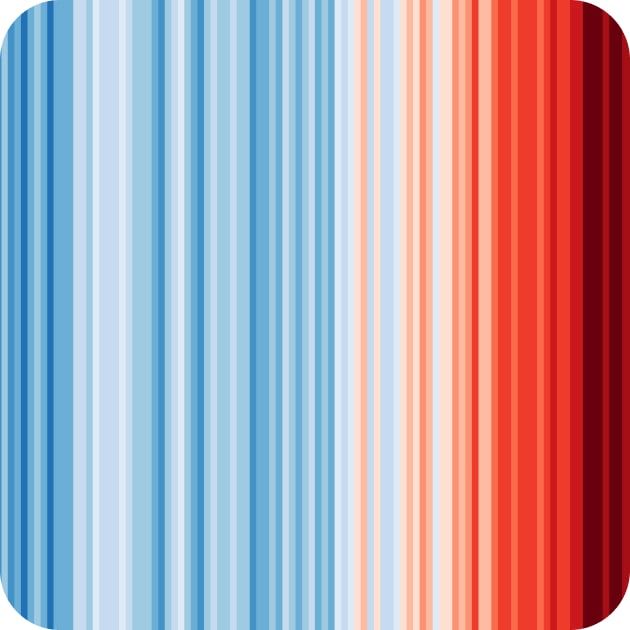
Serialize
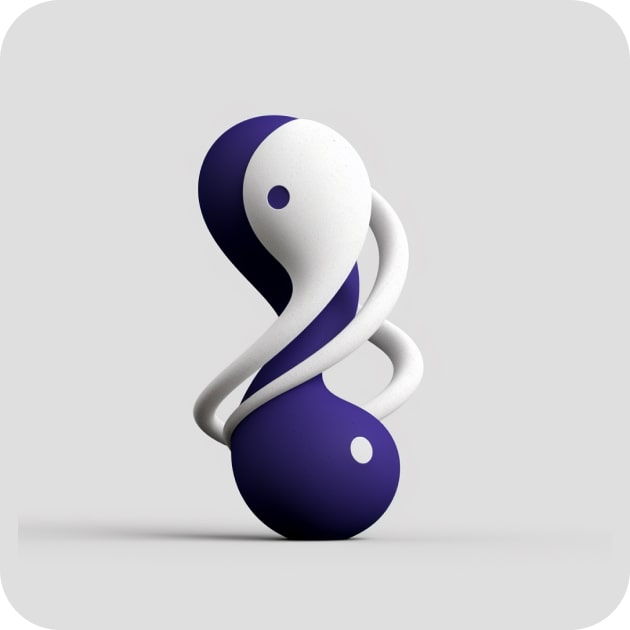
Shred
Skew
Split
Standardize
Tokenize
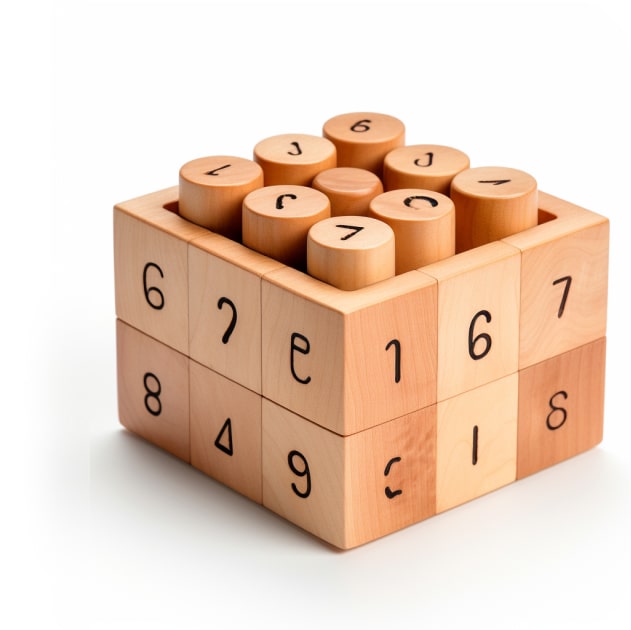
Transform
Wrangle
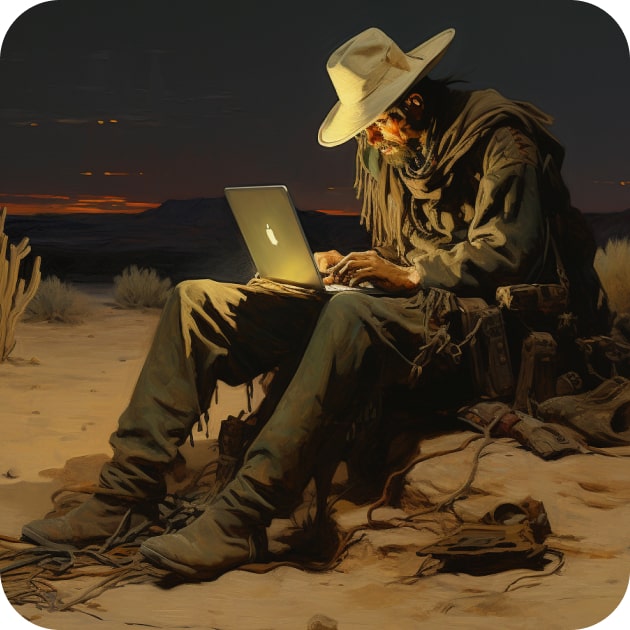