Dagster Data Engineering Glossary:
Data Lineage
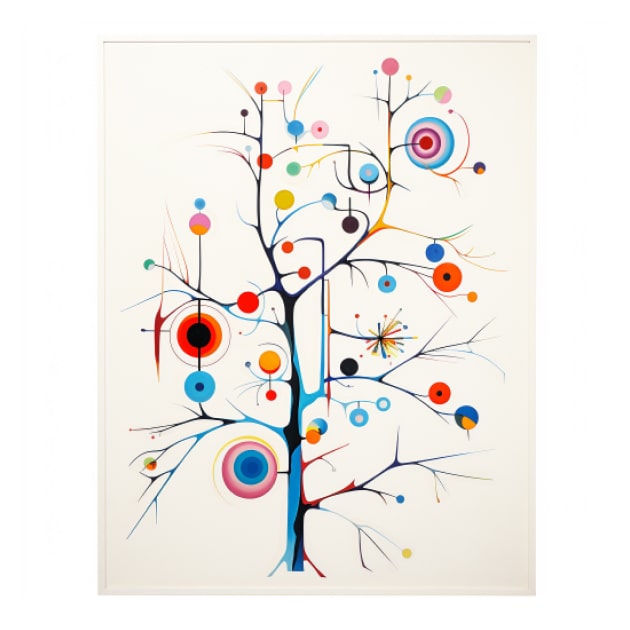
Data Lineage definition:
Data lineage refers to the visualization and understanding of how data moves through an ecosystem, including its origin, transformations, dependencies, and ultimate consumption. Effective data lineage provides a clear and comprehensive view of where data comes from, how it moves and changes, and where it ends up within a system or workflow.
Lineage is a critical concept in data engineering, data analysis, and data governance.
Here's a breakdown of the concept:
Origin or Source: Where does the data come from? This could be databases, external systems, flat files, APIs, or other sources.
Transformations: As data moves from its origin to its final destination, it might go through various transformations. This could be an ETL (Extract, Transform, Load) processes, data cleaning operations, or any other type of manipulation a data engineer or data scientist might define.
Dependencies: Understanding what datasets, processes, or tools depend on each other is key. If a source changes or has an issue, it's vital to know what downstream processes or datasets might be affected.
Consumption: This refers to the final resting place of the data or where it gets consumed. It might end up in a data warehouse, a report, a machine learning model, or any other type of application or system.
Why is data lineage important?
Trust & Data Quality: Understanding the full path of data helps ensure data quality and build trust in the data. If there's an anomaly in a report, you can trace back to see where the data came from and how it was transformed.
Impact Analysis: If a system or process needs to change, data lineage can show what downstream processes or datasets might be affected.
Compliance & Audit: Many industries have regulatory requirements around data. Knowing the full lineage of data can help ensure compliance, as you can demonstrate where data comes from and how it's used.
Optimization & Debugging: Having a clear understanding of data lineage speeds up troubleshooting.
Knowledge Sharing: For large organizations, not everyone might be aware of all data processes. You might have data scientists, data engineers, software engineers and data architects all collaborating on building data pipelines. Data lineage provides a clear map for everyone to understand the data flow, and hels answer pointed questions from data consumers.
To sum this up, data lineage is all about transparency, trust, and understanding in a data-driven ecosystem. Given the increasing complexity of modern data pipelines and the critical importance of data in decision-making, maintaining clear lineage is essential for most organizations and data teams.
Data lineage in Machine Learning
Lineage plays a significant role in machine learning (ML). Given that machine learning models are data-driven, understanding where data comes from, how it's processed, and how it's used is crucial for both model development and deployment. Here's an exploration of the role of data lineage in machine learning:
Reproducibility: One of the data engineering challenges in ML is ensuring that results (model training, evaluations, predictions) can be reproduced. Knowing the lineage of the data used to train and test a model provides clear documentation of the data's origins and the transformations it underwent, which is essential for reproducing results.
Model Interpretability: If an ML model produces unexpected or erroneous results, understanding lineage can help pinpoint potential issues in the data sourcing or preprocessing stages that might be contributing to the problem.
Model Auditing: For regulatory and compliance purposes, organizations might need to audit ML models, especially in critical domains like finance or healthcare. Data lineage provides a trail of evidence showing where the model's data originated and how it was processed, aiding in transparency and accountability.
Data Quality Assurance: Poor data quality can significantly degrade the performance of ML models. Tracking data lineage allows teams to trace back and identify sources of noise, missing values, or anomalies that might affect model quality.
Feature Engineering: Features, derived from raw data, play a crucial role in ML. Understanding the lineage of features—how they were derived, transformed, and encoded—is essential for model tuning and optimization.
Model Versioning: Just as code versioning is crucial in software development, model versioning is essential in ML, and lineage is a significant part of this. If you retrain a model on new data or with different preprocessing, tracking lineage can help you understand differences between model versions.
Collaboration & Knowledge Transfer: ML teams often comprise multiple roles - data engineers, data scientists, ML engineers, and domain experts. A clear understanding of data lineage ensures that all team members have a shared understanding of the data, its sources, and its transformations.
Operational Monitoring: After deploying an ML model, it's crucial to monitor its performance. If the model starts to drift or produce unexpected results, data lineage can help teams quickly determine if changes or anomalies in input data might be the cause.
Bias & Fairness: Ensuring models are fair and unbiased is crucial. Successful ML engineering means tracing potential sources of bias in the data collection or preprocessing stages.
Data lineage in computer science provides transparency, traceability, and accountability. As ML models become increasingly integrated into critical applications, the need for clear documentation and understanding of data sources and transformations grows more vital. Data lineage tools and practices help meet this need.
Read more about orchestrating Machine Learning pipelines in Dagster.
Data Lineage Tools & Technologies:
Many data governance and data management tools offer data lineage features. These tools can automatically track and visualize data lineage, helping data engineers and architects understand complex data flows. Dagster builds data lineage directly into the orchestration process thanks to its unique asset-centric approach.
Coding example of data lineage in Python
Data lineage is a hard concept to capture in a single coding example, but we can illustrate the idea, even though real-world data engineering jobs involve more complexity and typically employ specialized tools.
Let's consider a data pipeline with multiple data sources and data processing stages. We'll use Python classes to encapsulate these stages and maintain lineage metadata along the way. We will mock a data warehouse and an API.
Here's a simple mock-up of a data pipeline that tracks lineage:
class DataSource:
def __init__(self, name, data):
self.name = name
self.data = data
self.lineage = [f"Source: {name}"]
def get_data(self, user_id=None):
if user_id is None:
return self.data, self.lineage
return [record for record in self.data if record['user_id'] == user_id][0], self.lineage
class Transformation:
def __init__(self, name, function):
self.name = name
self.function = function
def apply(self, data, lineage):
transformed_data = self.function(data)
lineage.append(f"Transformed by: {self.name}")
return transformed_data, lineage
class DataSink:
def __init__(self, name):
self.name = name
def save(self, data, lineage):
# For simplicity, we're just printing here.
print(f"Data saved to {self.name}: {data}")
print("Lineage:")
for step in lineage:
print(" -> ", step)
# Mock multiple data entries for Database_A
data_entries_db_a = [
{"user_id": 1, "name": "Thom Yorke", "use_case": "lead singer"},
{"user_id": 2, "name": "Jonny Greenwood", "use_case": "guitarist"},
{"user_id": 3, "name": "Ed O'Brien", "role": "guitarist" },
{"user_id": 4, "name": "Philip Selway", "role": "drums"},
{"user_id": 5, "name": "Colin Greenwood", "role": "bass"}
]
# Mock multiple data entries for API call
data_entries_api_b = [
{"user_id": 1, "born": "1968"},
{"user_id": 2, "born": "1971"},
{"user_id": 3, "born": "1968"},
{"user_id": 4, "born": "1967"},
{"user_id": 5, "born": "1969"}
]
source1 = DataSource("Database_A", data_entries_db_a)
source2 = DataSource("API_B", data_entries_api_b)
transform1 = Transformation("Merge Data", lambda data: {**data[0], **data[1]})
transform2 = Transformation("Add Status", lambda data: {**data, "status": "processed"})
sink = DataSink("Warehouse_X")
def pipeline():
# Process each entry from Database_A
for data1_entry in source1.data:
user_id = data1_entry["user_id"]
data1, lineage1 = data1_entry, source1.lineage
data2, lineage2 = source2.get_data(user_id)
merged_data, merged_lineage = transform1.apply([data1, data2], lineage1 + lineage2)
processed_data, final_lineage = transform2.apply(merged_data, merged_lineage)
sink.save(processed_data, final_lineage)
print("-----------------------") # Separator for clarity
pipeline()
In this example:
- We've structured
DataSource
,Transformation
, andDataSink
classes to maintain lineage information. - Each time data is pulled or transformed, its lineage is updated with relevant metadata.
- The pipeline combines data from two sources, applies two transformations, and then saves the data. The lineage is printed, showing the journey of the data.
The output of our example would be:
Data saved to Warehouse_X: {'user_id': 1, 'name': 'Thom Yorke', 'use_case': 'lead singer', 'born': '1968', 'status': 'processed'}
Lineage:
-> Source: Database_A
-> Source: API_B
-> Transformed by: Merge Data
-> Transformed by: Add Status
-----------------------
Data saved to Warehouse_X: {'user_id': 2, 'name': 'Jonny Greenwood', 'use_case': 'guitarist', 'born': '1971', 'status': 'processed'}
Lineage:
-> Source: Database_A
-> Source: API_B
-> Transformed by: Merge Data
-> Transformed by: Add Status
-----------------------
[...]
Naturally, real-world data pipelines would likely be more complex, involve permanent data storage, and might not involve Radiohead band members. Advanced data engineers would typically employ dedicated tools for data modeling and analyzing data, especially in larger data ecosystems with cloud computing.
A solution like Dagster naturally tracks lineage for you and provides the tools for data engineers to rapidly observe, track and debug data pipelines. Furthermore, data lineage is directly related to the set of dependencies that exist within data pipelines, and Dagster's built-in tracking of dependencies allows data engineers, software engineers, and data architects to tap into these when building data pipelines.
We hope this overview helps you in building your data engineering skills. Mapping data provenance will help manage your data processing systems, support data analysts, maintain solid data architecture, and keep track of sources like data warehouses and data lakes.
Append
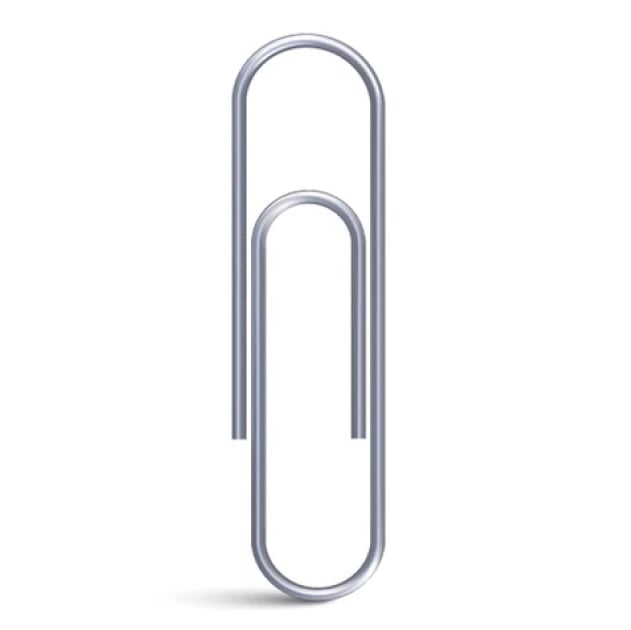
Archive
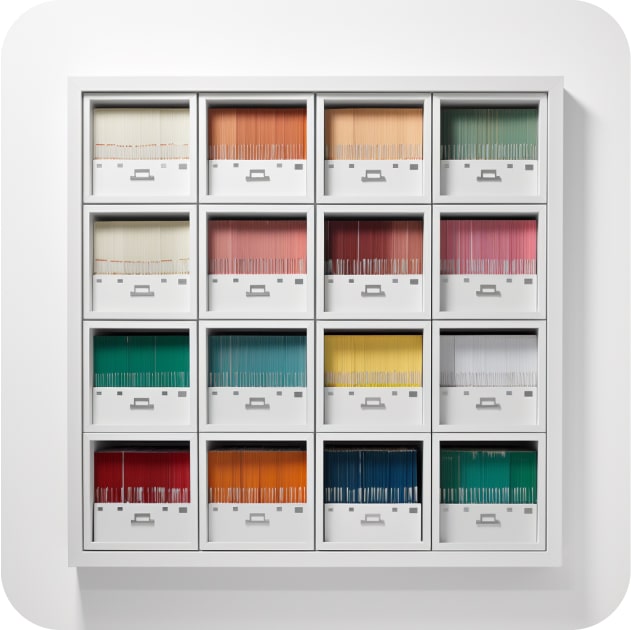
Augment
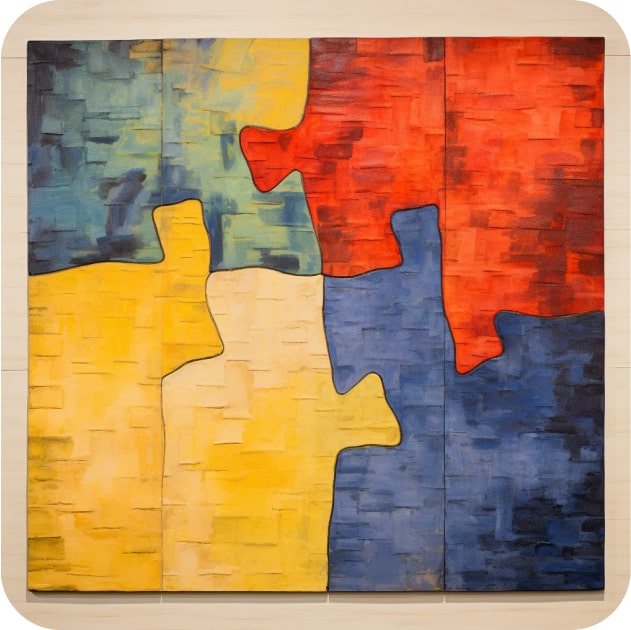
Auto-materialize
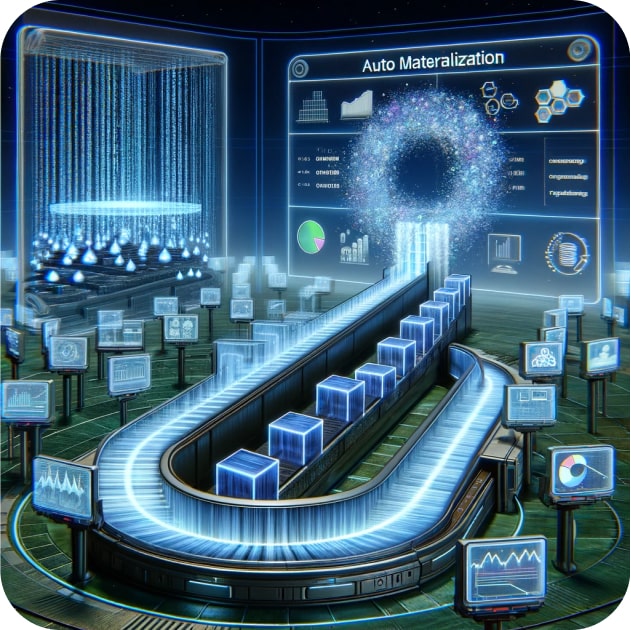
Backup
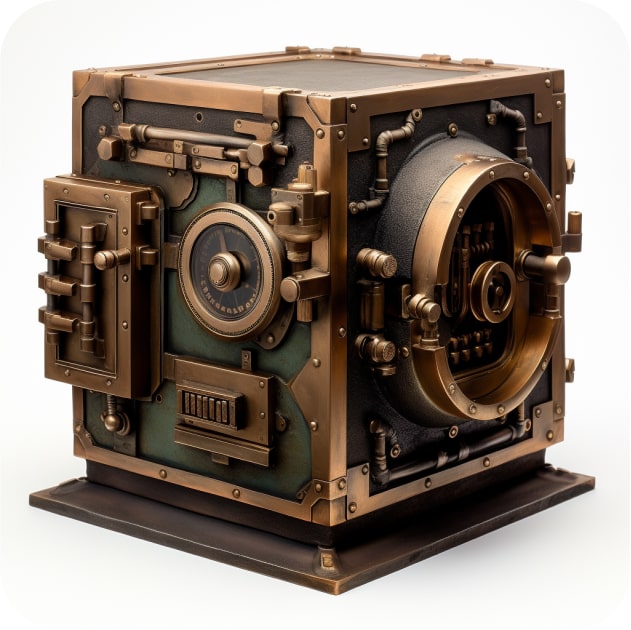
Batch Processing
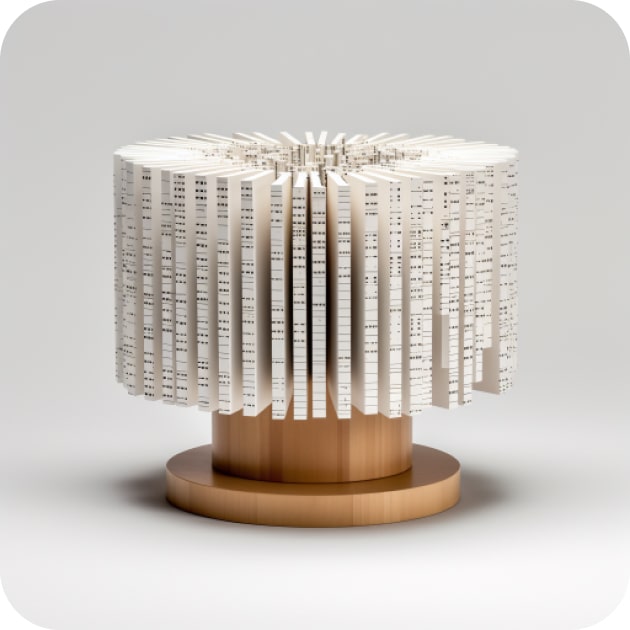
Cache
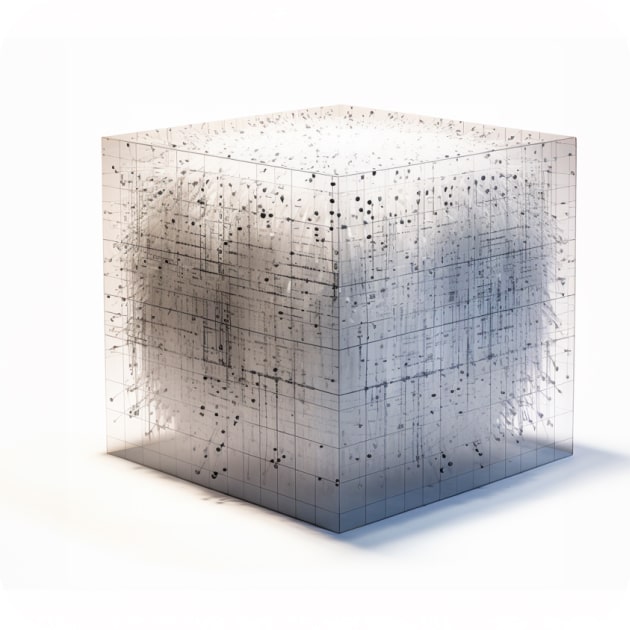
Categorize
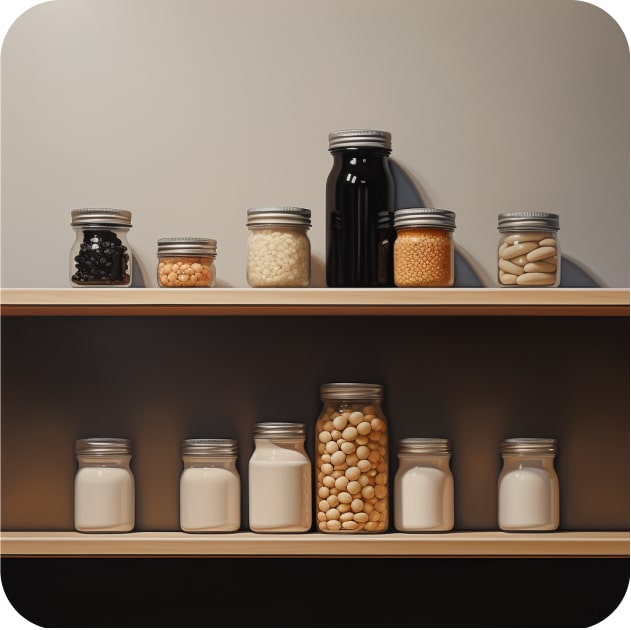
Checkpointing
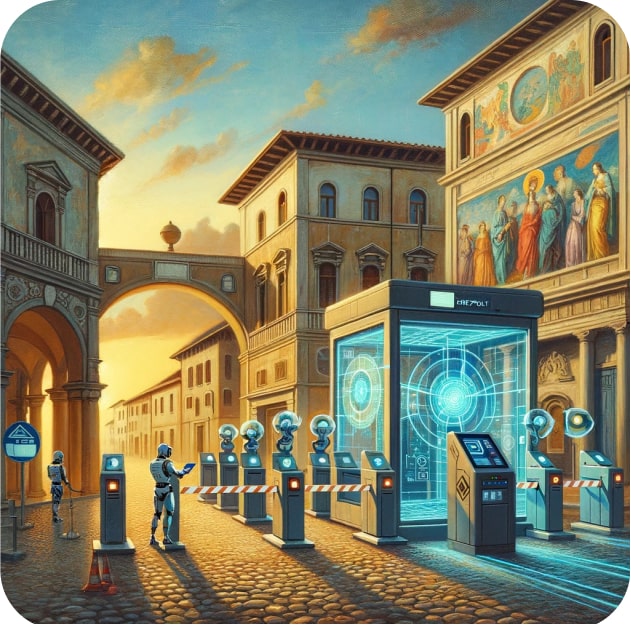
Deduplicate
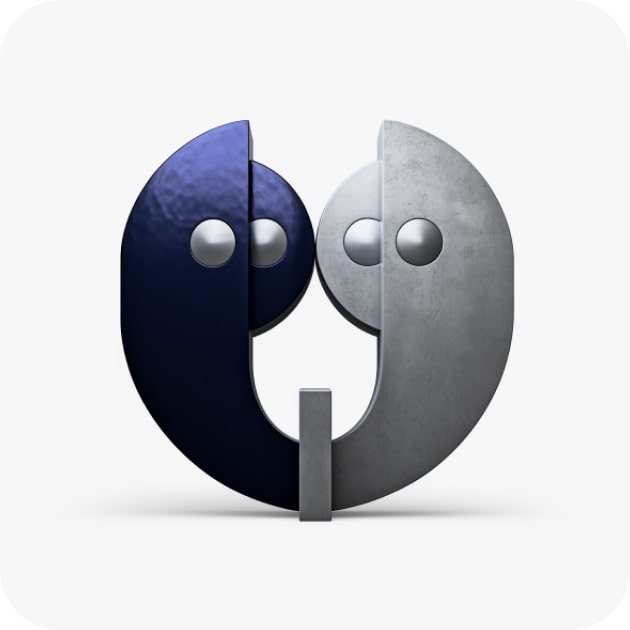
Deserialize
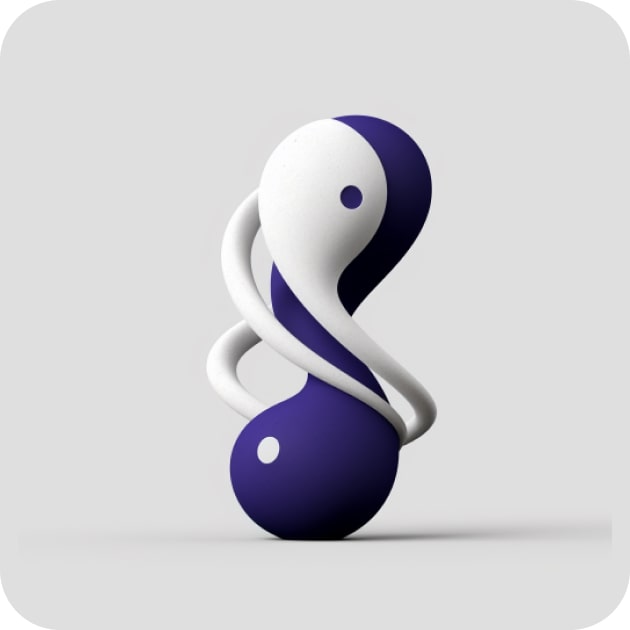
Dimensionality
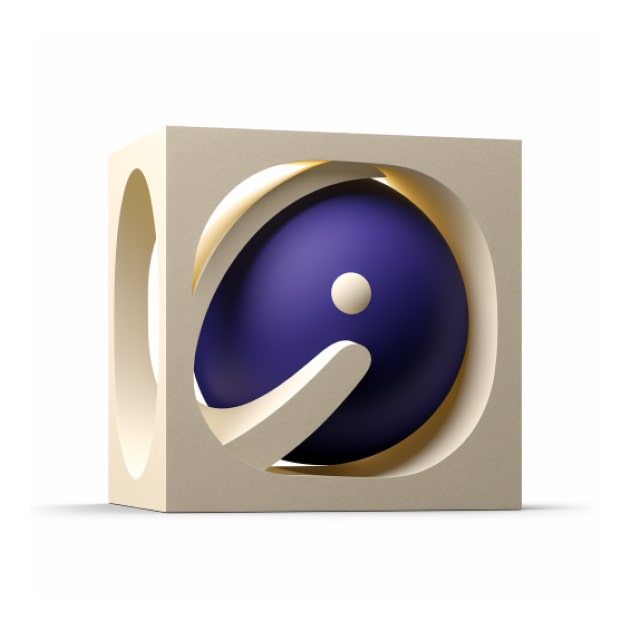
Encapsulate
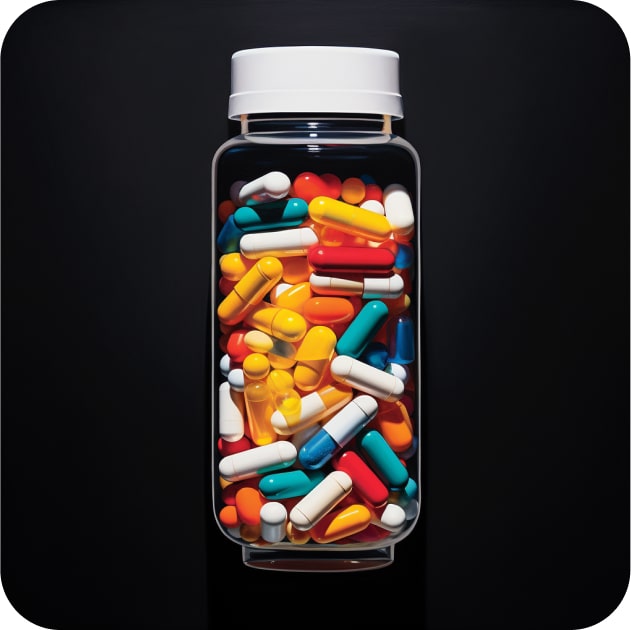
Enrich
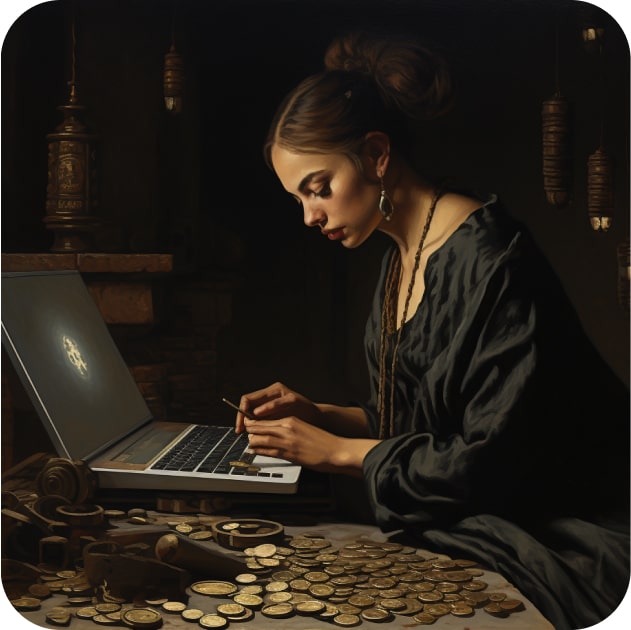
Export
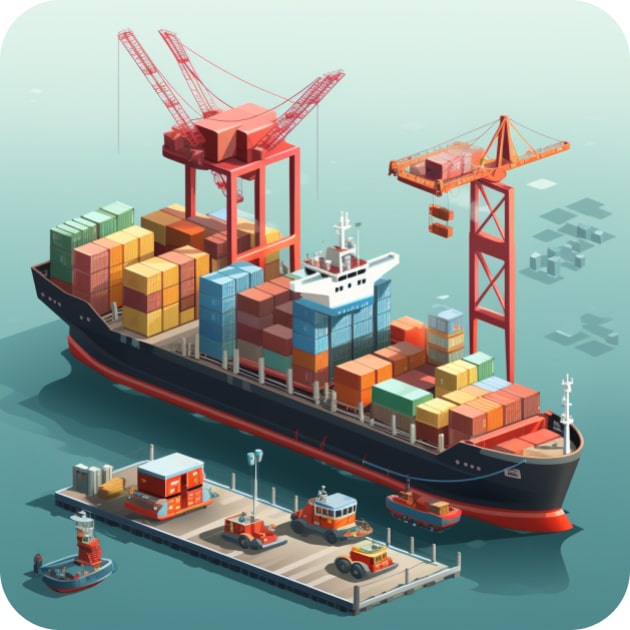
Graph Theory
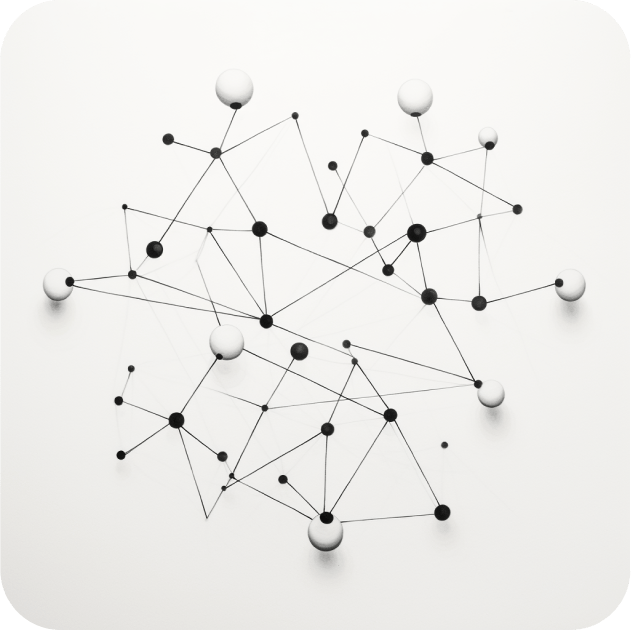
Idempotent
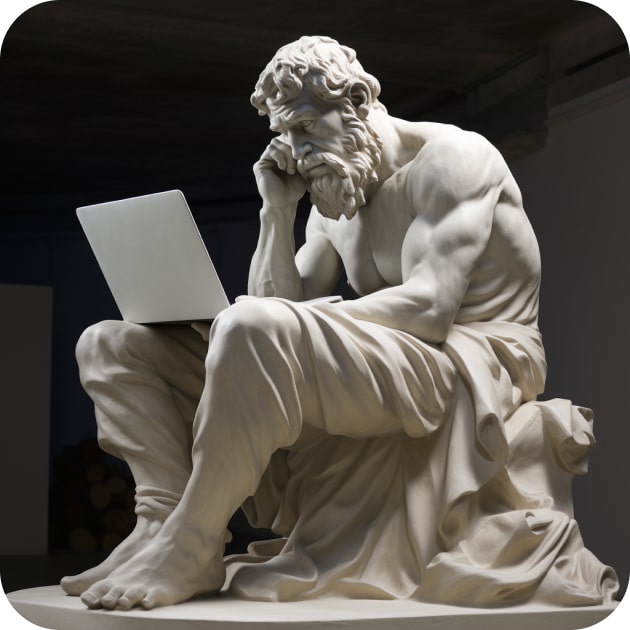
Index
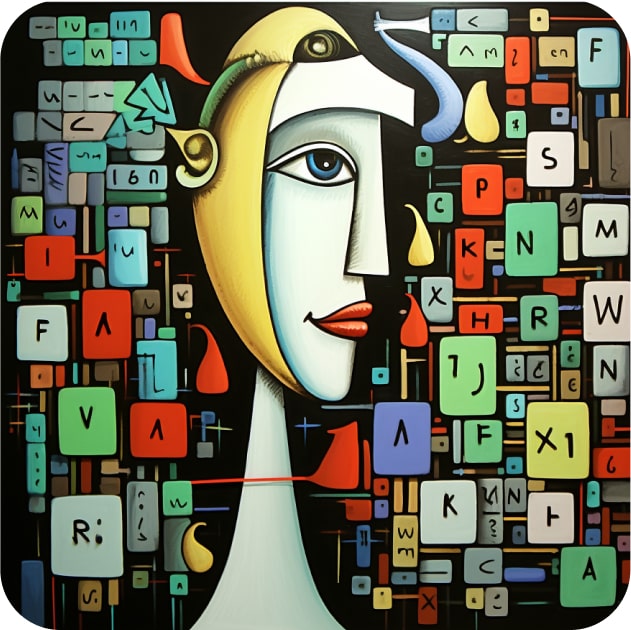
Integrate
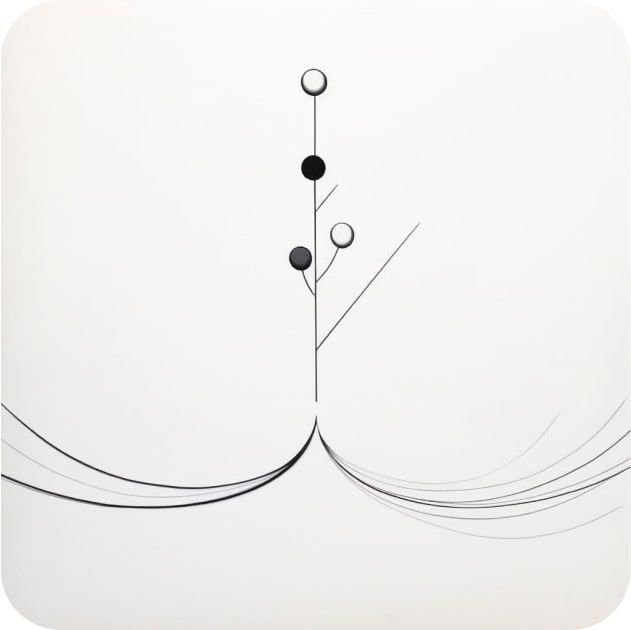
Linearizability
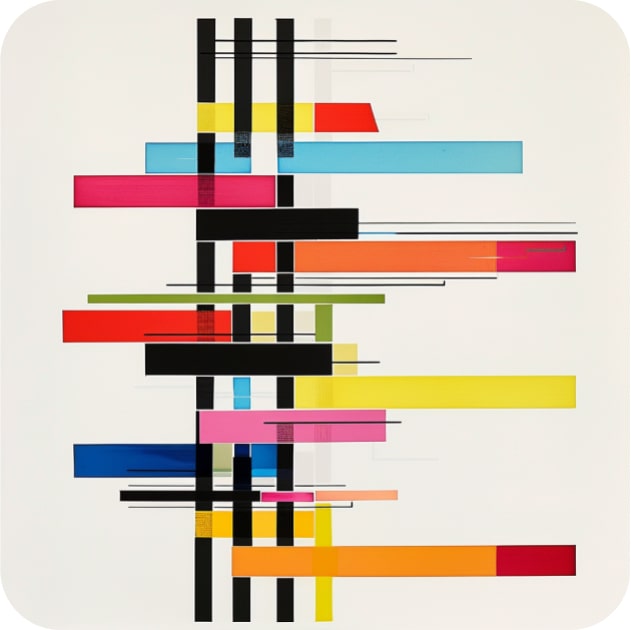
Materialize
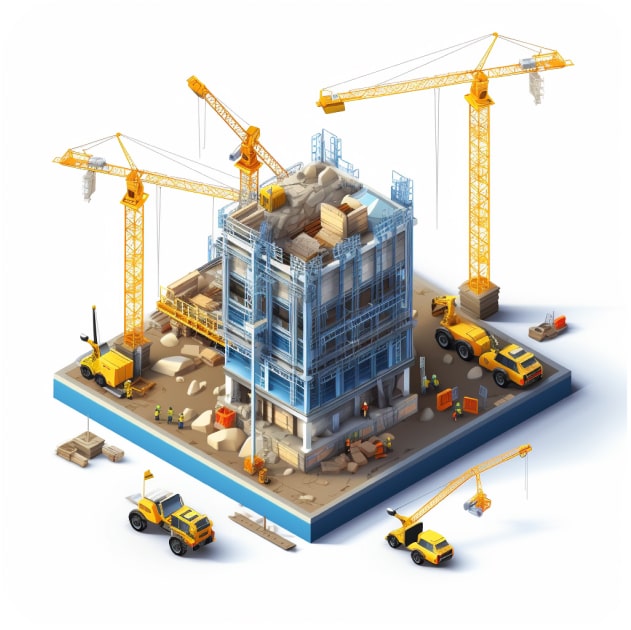
Memoize
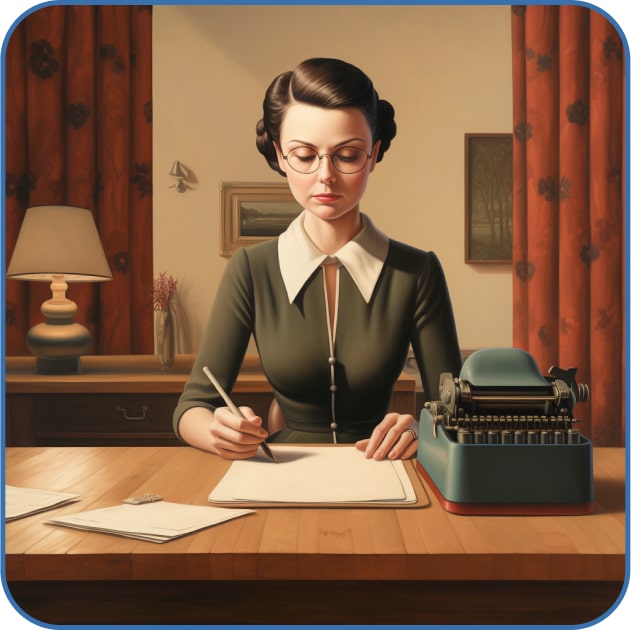
Merge
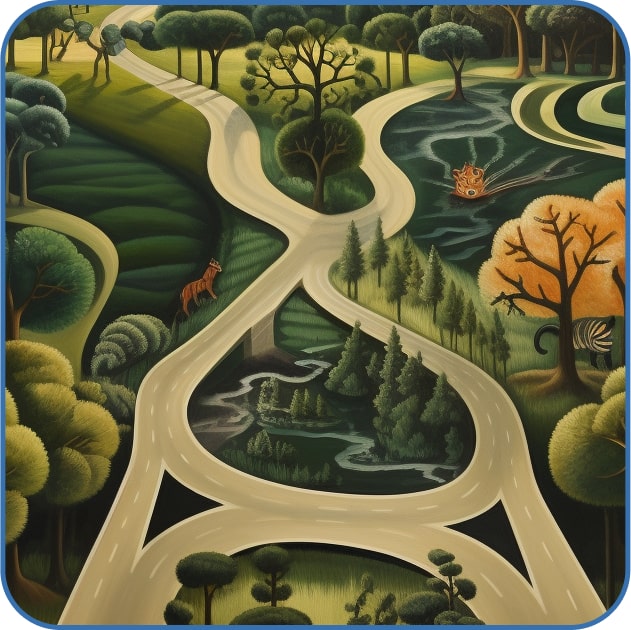
Model
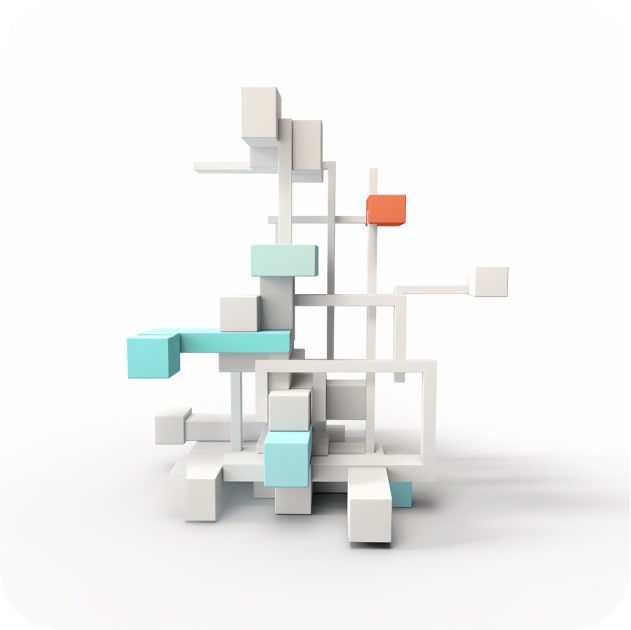
Monitor
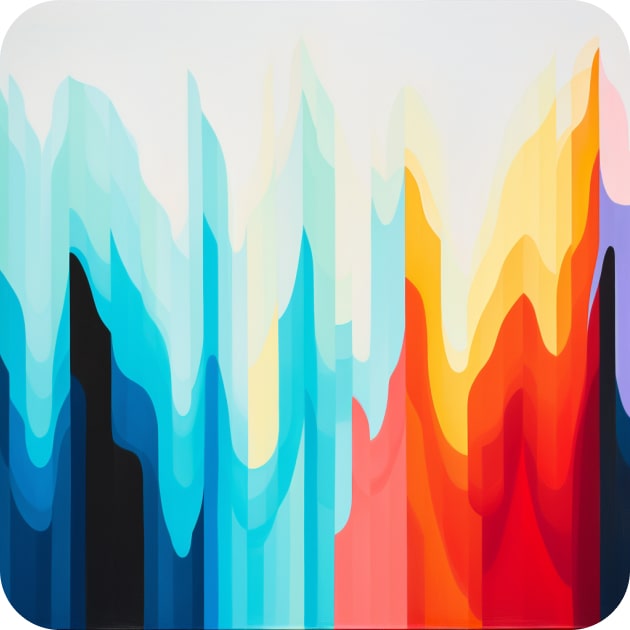
Named Entity Recognition
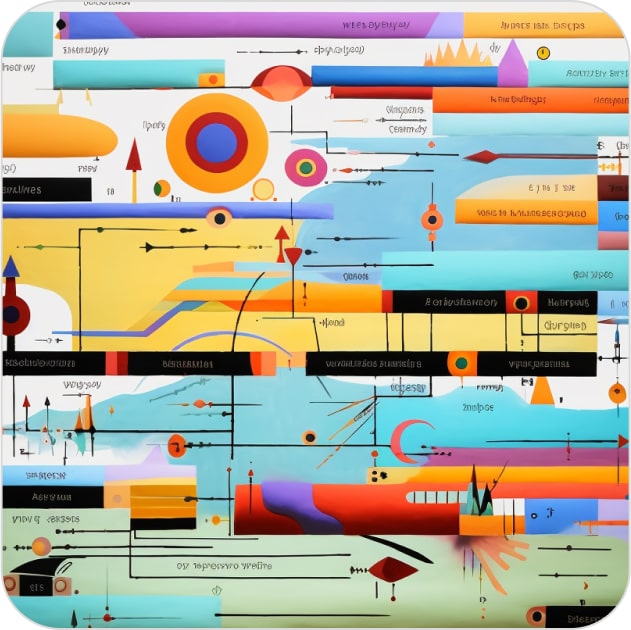
Parse
Partition
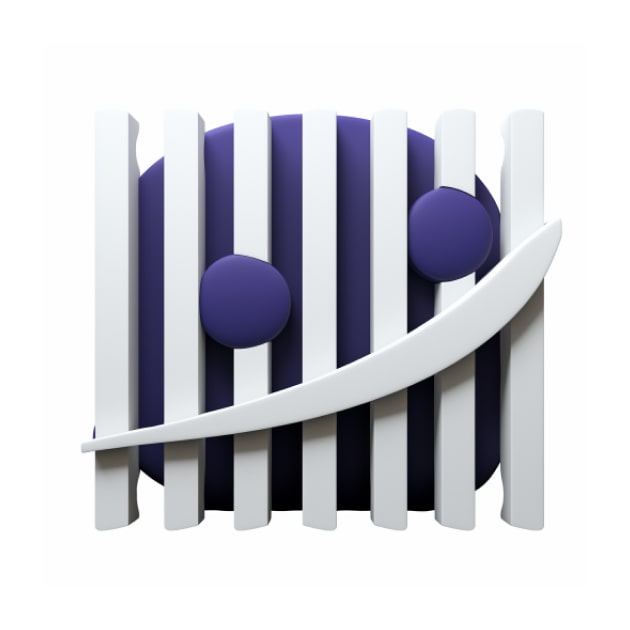
Prep
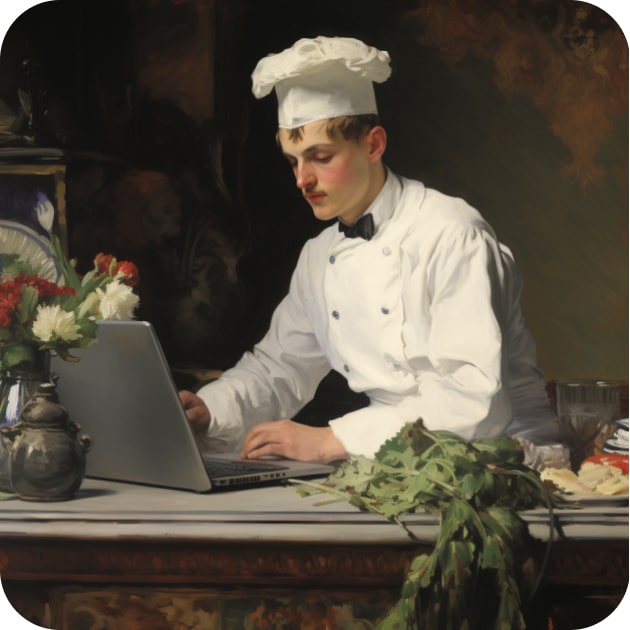
Preprocess
Primary Key
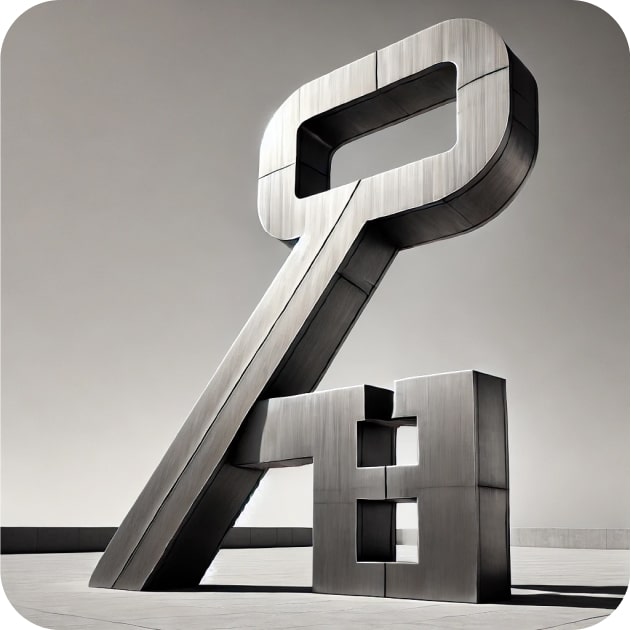
Replicate
Scaling
Schema Inference
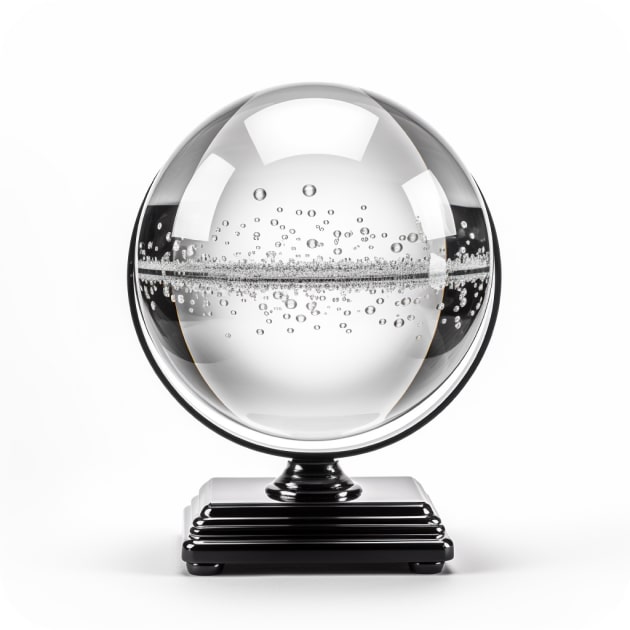
Schema Mapping
Secondary Index
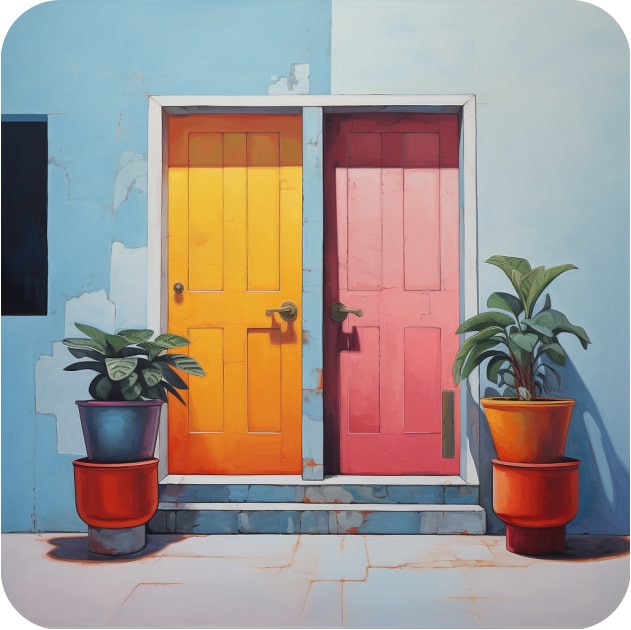
Software-defined Asset
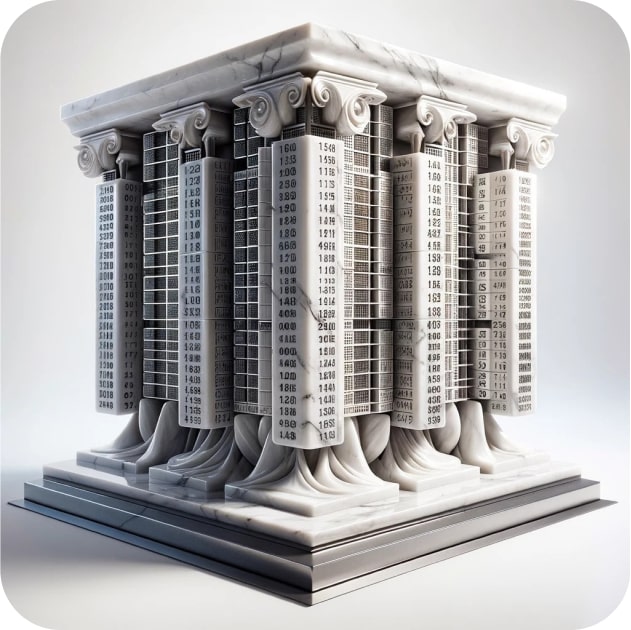
Synchronize
Validate
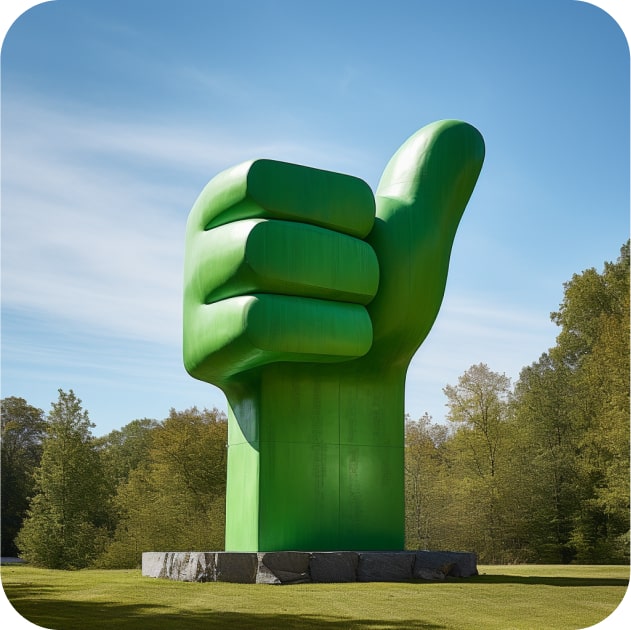
Version
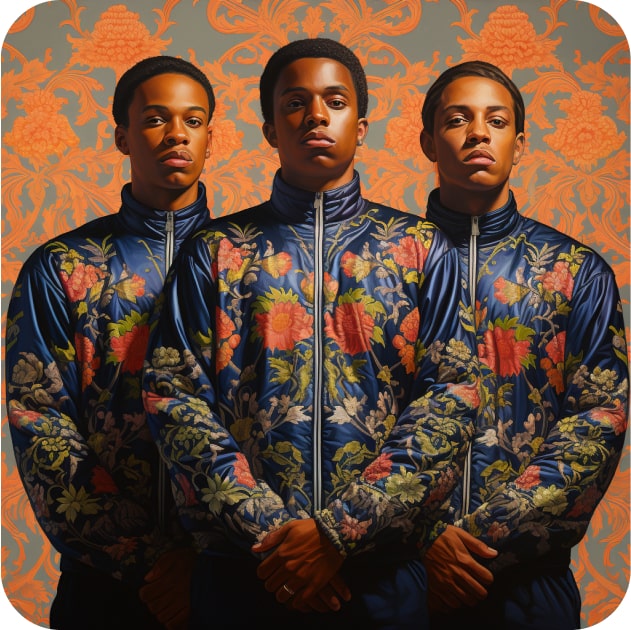