February 23, 2024 • 9 minute read •
Balancing the Data Scales: Centralization vs. Decentralization
- Name
- TéJaun RiChard
- Handle
- @tejaun
- Name
- Fraser Marlow
- Handle
- @frasermarlow
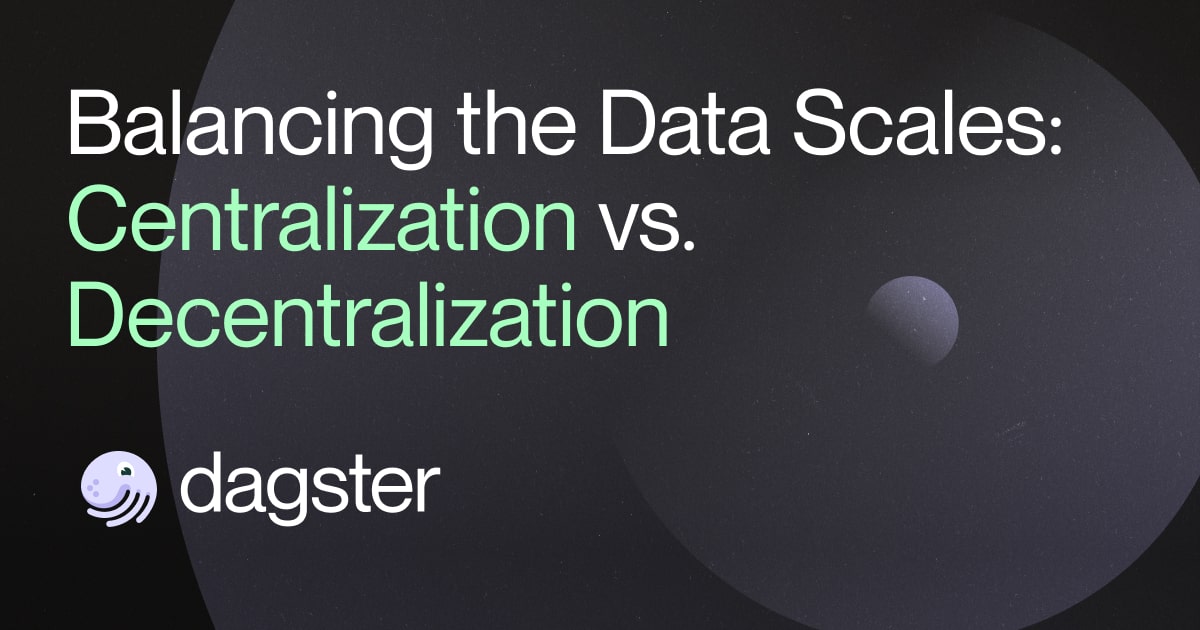
As organizations become more data-driven, they face a key challenge: to rapidly build out tools and teams to harness the value of the data without creating an unmanageable, messy collection of heterogeneous tools and technologies.
The risk of not having the correct data at the right time is real and costly. But the complexity and chaos that can emerge without well-thought-through systems is also real.
Organizations are under pressure to both move fast and remain disciplined in their building of data systems.
So, how should they address this challenge?
Some teams try to tame this problem by consolidating control of their data management within a single, centralized team or authority within the organization.
Others allow for the independent management of data through different departments and/or teams in the organization in a more decentralized approach.
While both methods offer situational benefits, it’s important to note that choosing between them isn’t a binary decision but rather a spectrum of approaches between full centralization and complete decentralization. Many organizations struggle not because they choose one method over the other, but because they lean too heavily towards one extreme, neglecting the advantages of a more balanced approach. Neither method is inherently wrong or bad. Instead, identifying the right blend that aligns with your organization's unique needs and circumstances can enable you to harness the strengths of both approaches to optimize your data management practices.
This post will explore the initial challenges of strictly centralized or decentralized data management approaches and propose a balanced solution: centralized oversight with decentralized execution.
Centralized vs. Decentralized Approach
Centralization
In a centralized approach, a small group manages all data-related activities. While centralized data management is praised for its streamlined decision-making and standardization, having all decisions go through this small group can lead to bottlenecks, as the central team becomes a gatekeeper for data requests and processing.
For instance, consider a sales team that needs new metrics. They submit a request to the central data organization, where a data engineer manager assigns the task to a data engineer. Not embedded within the sales team, this engineer must navigate a series of back-and-forth communications to understand and fulfill the request, leading to delays and potential misunderstandings.
The centralized approach also harbors additional challenges that might not be immediately apparent, like:
- Innovation Stifling: Centralized systems often stifle innovation due to their rigid control structures, making it difficult for teams to experiment and implement new ideas quickly. For example, a tech startup’s delay in approving a new data analysis tool due to centralized vetting processes, resulting in them missing crucial market opportunities.
- Knowledge Bottlenecks: Centralization can lead to knowledge bottlenecks where a few individuals hold the majority of expertise, creating a single point of failure and slowing down knowledge transfer. For example, a healthcare provider's IT department slowing critical patient care improvements when its few senior data architects are unavailable.
- Diminished Empowerment: A strictly centralized environment can inadvertently reduce team members’ sense of autonomy, as they must rely on a central authority for data requests. This can stifle their curiosity and innovation, leading to a less engaged and empowered workforce. For example, a digital marketing agency's creative team waiting on data for tailored campaigns because they feel demotivated to act without it, leading to less effective marketing overall.
- Scalability Issues: As the organization grows, the centralized team may struggle to scale its operations effectively, leading to longer turnaround times and decreased agility. For example, a rapidly expanding ecommerce platform, where the central data team is struggling to keep up with the demands for real-time customer behavior analytics, ultimately leading to missed opportunities for personalized marketing campaigns during peak shopping seasons.
- Overdependence on Central Units: Heavy reliance on a central unit for data management can create a risk of operational paralysis if the unit faces issues, impacting the entire organization’s data capabilities. For example, a multinational corporation's global operations are paralyzed due to its central data processing unit falling victim to a cyber-attack and its local branches’ inability to access critical business intelligence.
Decentralization
On the other hand, a decentralized approach allows people across different departments to manage their data independently. On the surface, decentralization promotes flexibility and rapid response to department-specific needs. However, this separation can result in varying levels of consistency and expertise across the organization.
Revisiting our hypothetical scenario, imagine a sales engineer (SE) who has been closely involved with the sales team, developing a deep and intimate understanding of their data requirements, sales cycles, and customer interactions. This SE, familiar with the nuances of sales data and the team’s objectives, could leverage their specialized knowledge to quickly identify and extract the most relevant data sets, apply appropriate analytics, and generate insights tailored to the sales team’s immediate needs. Utilizing advanced data tools and their knowledge of sales operations, this SE could efficiently fulfill requests by creating custom reports or dashboards that directly address the sales team’s specific questions, leading to faster decision-making and targeted strategies.
However, this SE might lack a broader understanding of company-wide data practices, leading to inconsistencies and potential data quality issues. For example, without a unified view of the organization’s data governance policies, the SE may end up creating reports that don’t align with company-wide data security standards or that fail to incorporate data from other departments that could provide additional insights. This siloed approach risks producing insights that, while valuable within the sales department, may not align with the strategic objectives of the org or overlook crucial cross-functional data interdependencies - ultimately affecting the accuracy and utility of the data analysis.
Additionally, decentralization can bring other hidden challenges that can undermine the organization’s cohesion and data integrity, such as:
- Inconsistent Data Governance: A decentralized approach can lead to inconsistent data governance policies and standards across different departments, complicating compliance and data quality management, resulting in higher operational costs due to the need for individualized solutions and the potential for regulatory fines.
- Duplicated Efforts: Without centralized oversight, teams might end up duplicating efforts, creating siloed solutions for similar problems, leading to inefficient use of resources and significantly increased costs due to the redundant investment in technology and manpower.
- Difficulty in Data Integration: Decentralization can make it challenging to integrate data across different sources and departments, hampering the organization's ability to generate holistic insights and adding substantial costs as each local team adopts its own tools and technologies and increases expenditures on things like licenses, training, and maintenance.
- Lack of Expertise Distribution: While decentralization empowers local teams, it might also concentrate specific expertise too narrowly or lack them entirely, preventing the broader distribution of skills and knowledge and leading to higher costs as organizations potentially outsource or hire new talent to fill these gaps.
- Security Risks: With data spread across multiple decentralized units, ensuring consistent and effective security measures becomes more complex, increasing the risk of data breaches, which can be extremely costly in terms of financial loss, regulatory fines, and damage to reputation.
As you can see, too much of anything can be a bad thing. However, there are benefits to both, assuming you can use them both in moderation.
Establishing the Balance Between The Two
The key is finding a sweet spot that leverages the strengths of centralized and decentralized models. This balance ensures operational efficiency, risk reduction, and alignment with strategic business goals. These elements are paramount when trying to maximize the value and impact of data management within an organization.
The way that progressive organizations have achieved the benefits of both a centralized and the decentralized model is by building a data platform that establishes standards and allows for a shared resource while still facilitating local autonomy.
At this intersection though, it's also important to understand the role of data orchestration in realizing this balanced approach. Surpassing the traditional confines of just scheduling and debugging, data orchestration acts as the strategic command center for all data operations, seamlessly integrating the centralized and decentralized elements of data management. This integration and combination serves as a framework that powers the entire data ecosystem. By automating the flow of data across systems and processes, data orchestration ensures that information is not only accessible and reliable but also harmonized and standardized across the organization. This advanced and more strategic orchestration fosters a deep understanding of all data resources, turning data into actionable insights that empower decision-makers at every level of the organization.
A unified data platform is the pivotal element for achieving both the meticulous oversight needed for data standardization and the agility required for fostering innovation and responding swiftly to data-driven needs.
The Role of a Data Platform in Balancing Centralization and Decentralization
In order to understand the role of a data platform here, we first need to understand what a data platform is. A data platform is a comprehensive framework and set of technologies that enable data operations within an organization. These operations can include but aren’t limited to: collection, storage, analysis, management, governance, and processing of data. It integrates with existing data systems and can scale to accommodate organizational growth and future technological advancements.
A robust data platform serves as a foundation and levels the playing field for all teams. It is much more than the tools used to build data pipelines. It is a foundation that removes the common obstacles you face, allowing you to concentrate on innovation rather than routine tasks. It elevates the quality, speed, and ease of managing data pipelines, setting a new standard for your work.
Additionally, a data platform isn't confined to a purely centralized or decentralized approach. Instead, it offers you the flexibility to position it anywhere along that spectrum based on your needs.
In short, a data platform lays the groundwork for both centralized oversight and decentralized execution in your organization. It helps you leverage data as a strategic asset, supporting a balanced approach to data management that empowers your teams, aligns with your strategic goals, and drives innovation and growth.
Build and Run Data Pipelines
A data platform should simplify the building and running of data pipelines. It should be accessible enough that an analyst who may not fully understand the platform's intricacies can still quickly write and deploy data workflows. Just like how you wouldn't expect everyone who drives to know what happens under the hood of a car, a data platform lets people build pipelines and data assets without needing to know how to implement data quality, lineage, and other features; they just benefit from it coming out-of-the-box.
Establish Data Quality Standards
Establishing data quality standards is another critical aspect. A data platform can provide a unified way to monitor and ensure that all data tables meet the organization's format, partitioning, and optimization standards. It can automate best practices and provide a predefined way to alert the relevant stakeholders when something needs attention.
Automation and Alerts
Automation is a crucial feature of a data platform that supports both a centralized and decentralized approach, taking care of routine tasks and ensuring that processes are consistent and error-free. Alerts keep everyone informed, providing notifications when data events occur or if issues arise, ensuring that the right people can take action promptly.
Supporting the Local Teams
So, we have discussed the centralized capabilities of such a platform, but how does it support local autonomy?
A well-designed platform centralizes the common tasks of data management, especially the observability, scheduling, and common standards. But it also provides the flexibility for local teams to manage their own work autonomously, self-serve for tasks such as pipeline creation/editing, and access to shared data cataloging.
Importantly the platform has to be extensible and composable to the extent that a local team—say a data science team — can build and train their models or generate their data assets using the toolset of their choice, and still execute, observe, and share the critical data assets that other teams may need.
A Tailored Data Operations Experience
Embracing a balanced approach to data management is essential in today's complex and decentralized data environment. By finding the middle ground between centralized oversight and decentralized execution, organizations can enjoy the benefits of both worlds. This approach fosters a culture of collaboration and empowerment, where data is not just a resource but a catalyst for innovation and growth.
As you reflect on your organization's data management practices, consider where you stand in the centralization-decentralization spectrum. Are you leaning too heavily on one side, and could a unified data platform help you achieve the balance necessary for success?
The principles outlined here are universal, and you can apply them to any modern data orchestration solution that prioritizes flexibility, scalability, and robustness. It's about setting a standard that lifts the entire organization, ensuring that data is managed and orchestrated to drive value and insight.
You should note, though, that balance is not a one-size-fits-all solution. Each organization will have its unique needs and challenges. However, the principles of a balanced approach remain the same: clear standards, autonomy where it counts, and a platform that supports both.
Reflecting on Your Data Strategy
That said, maybe you could improve your operations with some balance.
Take a moment to consider your current data strategy:
- Do you find that a centralized team within your organization often bottlenecks requests for data and analytics?
- Due to a highly decentralized approach, are there data quality and process inconsistencies in your organization?
- Does everyone in your organization understand and follow clear standards and practices?
- Do local data teams appear productive, but then things fall apart when it comes to sharing and collaborating around data?
If you're encountering issues related to these things, it may be time to explore a data platform that can help you strike the right balance.
Tip: Our platform, Dagster, offers features that facilitate centralized oversight and decentralized execution, providing the flexibility and control needed to manage your data effectively. Learn more about how our platform can empower your data strategy by visiting our platform page.
The Next Steps
As you move forward, consider these next steps:
- Assessment: Conduct an audit of your current data management practices. Identify areas where centralization is causing delays and where decentralization leads to inconsistency.
- Research: While reviewing the capabilities necessary for a balanced data management approach, consider exploring our platform. Discover how it can facilitate centralized oversight and decentralized execution, and see if it aligns with your organization's needs.
- Engagement: Engage with your teams to understand their needs and pain points. A solution that works for one team may not work for another, so gathering diverse perspectives is essential.
- Piloting: Consider running a free trial of the platform. Measure the impact on speed, quality, and collaboration within your data teams.
- Feedback Loop: Create a feedback loop where data platform users can share their experiences and suggest improvements. The feedback will help refine your approach and ensure the platform evolves with your organization's needs.
Conclusion
Embracing a balanced approach to data management is essential in today's complex and decentralized data environment. By finding the middle ground between centralized oversight and decentralized execution, organizations can enjoy the benefits of both worlds and create a robust framework for managing data assets. Having the structure of centralization and the agility of decentralization helps foster a culture of collaboration and empowerment, where data is not just a resource but a catalyst for innovation and growth.
The correct data platform can help you streamline operations, foster innovation, and unlock the full potential of your data. It's about empowering your teams to work smarter, not harder, and ensuring that data is a powerful driver for decision-making and growth.
We're always happy to hear your feedback, so please reach out to us! If you have any questions, ask them in the Dagster community Slack (join here!) or start a Github discussion. If you run into any bugs, let us know with a Github issue. And if you're interested in working with us, check out our open roles!
Follow us:
Routing LLM prompts with Dagster and Not Diamond
- Name
- Colton Padden
- Handle
- @colton
AI Reference Architectures
- Name
- Dennis Hume
- Handle
- @Dennis_Hume
Data Platform Week 2024
- Name
- Alex Noonan
- Handle
- @noonan