Dagster Data Engineering Glossary:
Data Reduction
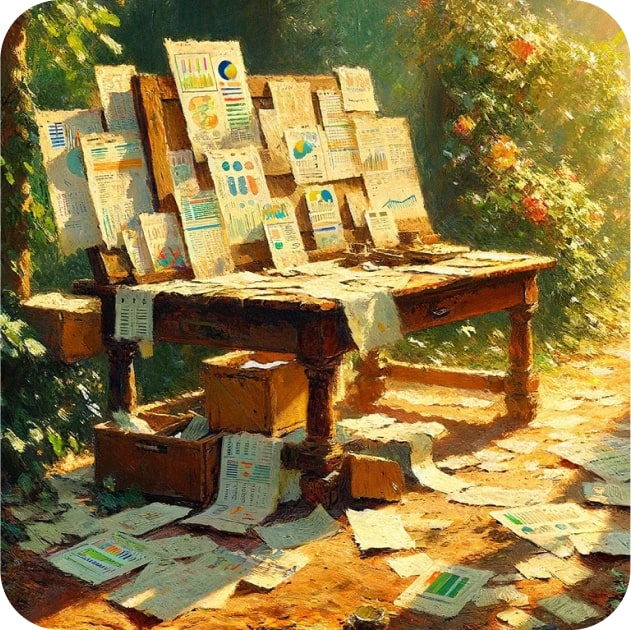
Data reduction definition:
Data Reduction in data engineering refers to the process of transforming, encoding, or otherwise converting a large set of data into a smaller, more manageable form without significant loss of information. This is usually done to increase efficiency, reduce data storage requirements, and improve the speed of subsequent data processing tasks.
Methods for data reduction can include dimensionality reduction techniques, data compression, data sampling, binning, feature selection, feature extraction, and aggregation, among others. The aim is to maintain the data's integrity and usefulness while making it more manageable.
Data reduction is especially crucial in big data applications, where the sheer volume of data can become a hindrance to data processing and analysis. By intelligently reducing data size, we can optimize storage, improve performance, and make the data more understandable.
Data reduction example in Python:
Please note that you need to have the necessary Python libraries installed in your Python environment to run this code.
Let's look at a simple data reduction method by applying a PCA (Principal Component Analysis) technique to a sample dataset. In a modern data pipeline, we often need to reduce the dimension of data, especially for high-dimensional data, to save storage and computational power.
We'll be using the famous Iris dataset from sklearn for the demonstration. This data set has four features, and we'll reduce it to two features using PCA.
Here's an example:
from sklearn.decomposition import PCA
from sklearn.datasets import load_iris
import pandas as pd
import seaborn as sns
import matplotlib.pyplot as plt
## Load the iris dataset
iris = load_iris()
X = iris.data
y = iris.target
## Original dataset dimensions
print(f"Original dataset shape: {X.shape}")
## Create the PCA object, specifying the number of components (or dimensions) to reduce to
pca = PCA(n_components=2)
## Fit and apply the PCA to the data (this is done in one step)
X_pca = pca.fit_transform(X)
## Reduced dataset dimensions
print(f"Reduced dataset shape: {X_pca.shape}")
## Convert numpy array to pandas dataframe
df = pd.DataFrame(data = X_pca, columns = ['Principal Component 1', 'Principal Component 2'])
df['target'] = y
## Visualize 2D Projection
plt.figure(figsize=(8,6))
sns.scatterplot(x='Principal Component 1', y='Principal Component 2', hue='target', data=df, palette='Set1')
plt.show()
In this script, we load the iris data set, which has 4 features. Then, we use PCA to reduce it to two features. We then plot these two features as a scatter plot to visualize the reduced data, which produces a graph as follows:
It will print out to the terminal:
Original dataset shape: (150, 4)
Reduced dataset shape: (150, 2)
This script demonstrates a fundamental data reduction technique that is commonly used in modern data pipelines, particularly when dealing with high-dimensional data.
Data reduction vs. data compaction vs. data compression
While related, reduction, compaction, and compression are slightly different techniques:
Reduction | Compaction | Compression | |
Aim | Reduce the overall amount of data while retaining its critical information. | Minimize storage space while preserving data contents and structure. | Reduce data size by encoding it in a more space-efficient format, minimizing transfer or storage. |
Techniques | Aggregation, downsampling, encoding, filtering out less relevant data points, dimensionality reduction for feature selection. | Data compression, merging smaller data segments into larger ones, and optimizing data structures like indexes to reduce fragmentation. | Encoding data in a compressed forms to represent data in fewer bits. |
Key benefits | Improve data manageability, simplify analytics, and speed up query performance. | Improve storage efficiency and reduce costs. | Reduce data transfer times, saving storage space, and improving data transmission efficiency. |
Reduction, compression, and compaction are complimentary and often used together to achieve our data engineering objectives.
Align
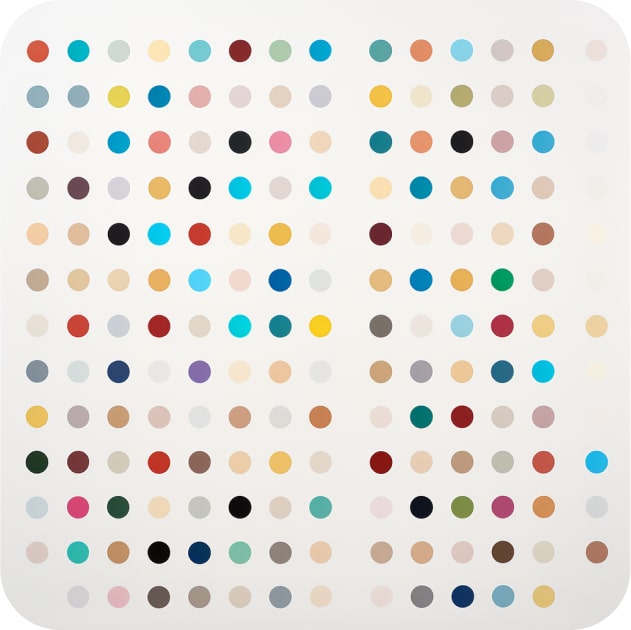
Clean or Cleanse
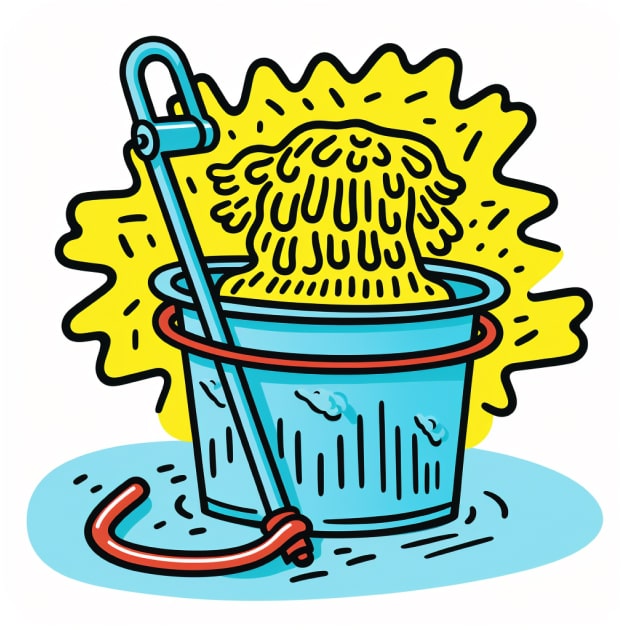
Cluster
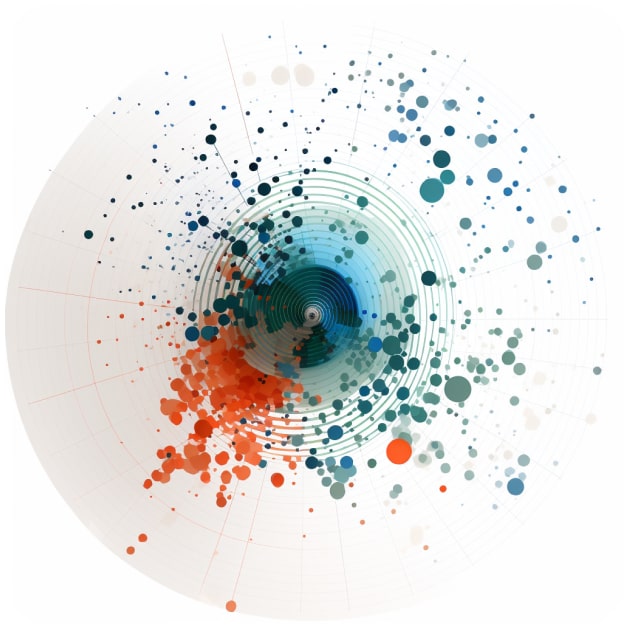
Curate
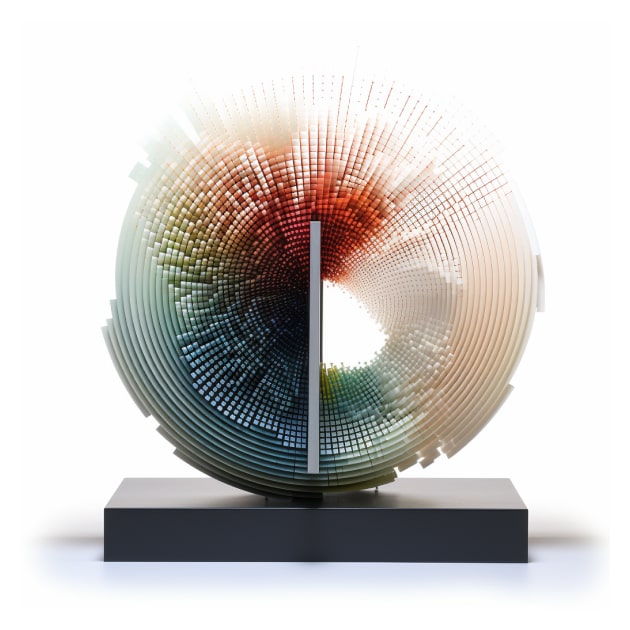
Denoise
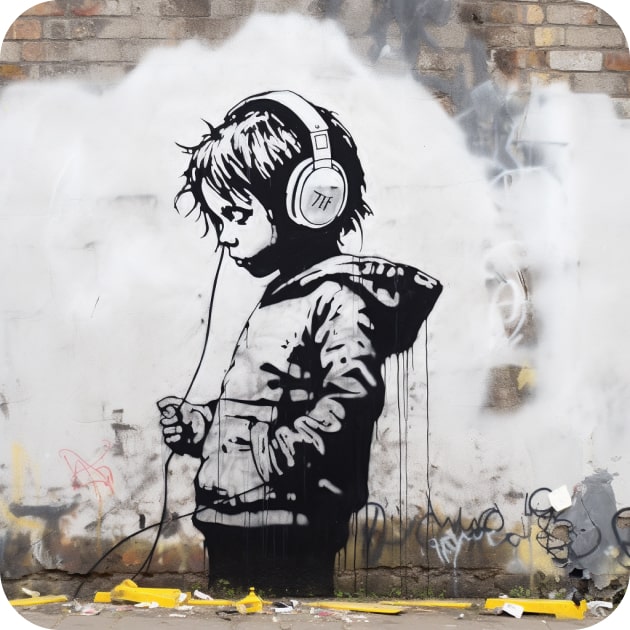
Denormalize
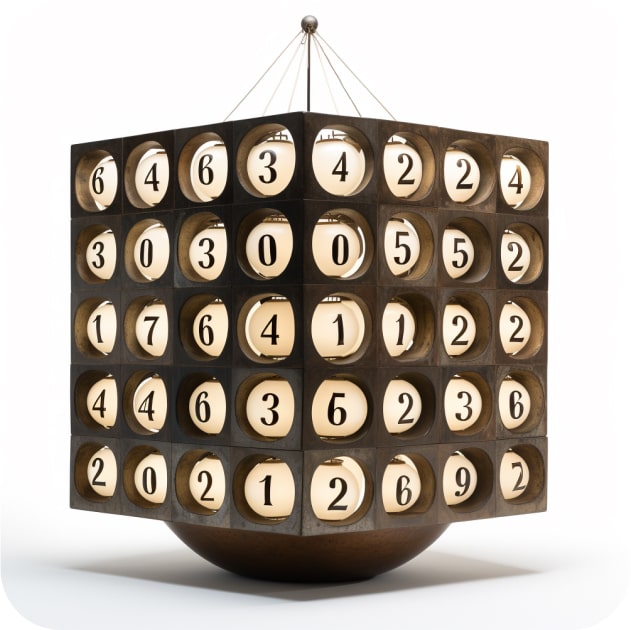
Derive
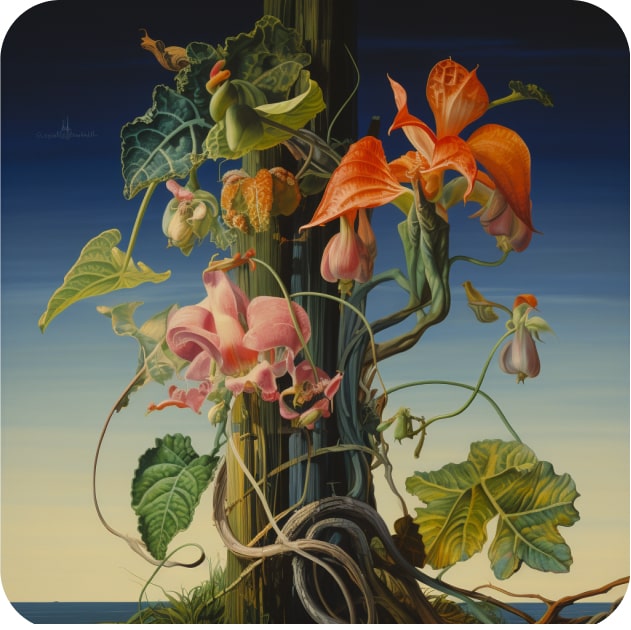
Discretize
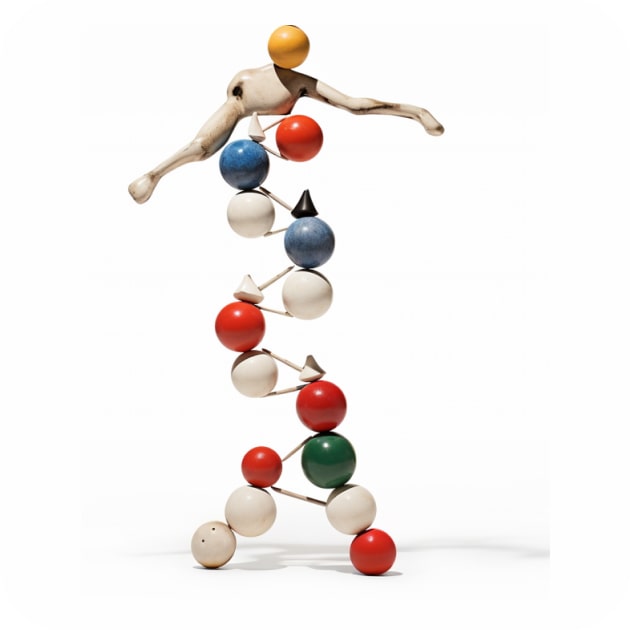
ETL
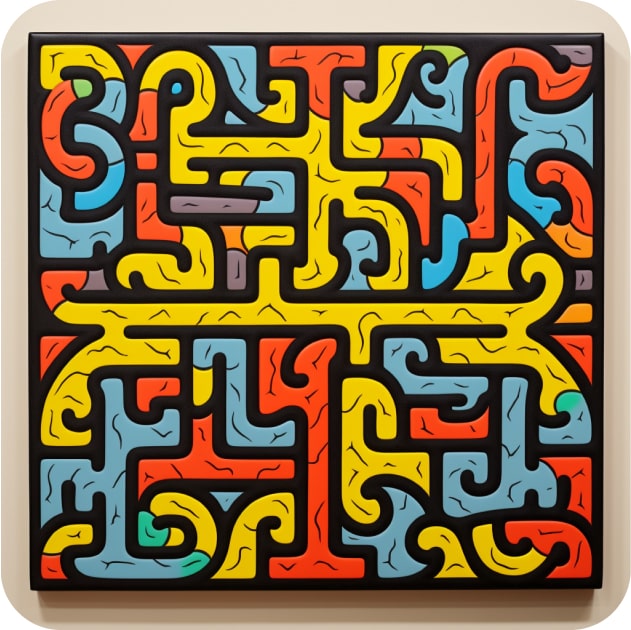
Encode
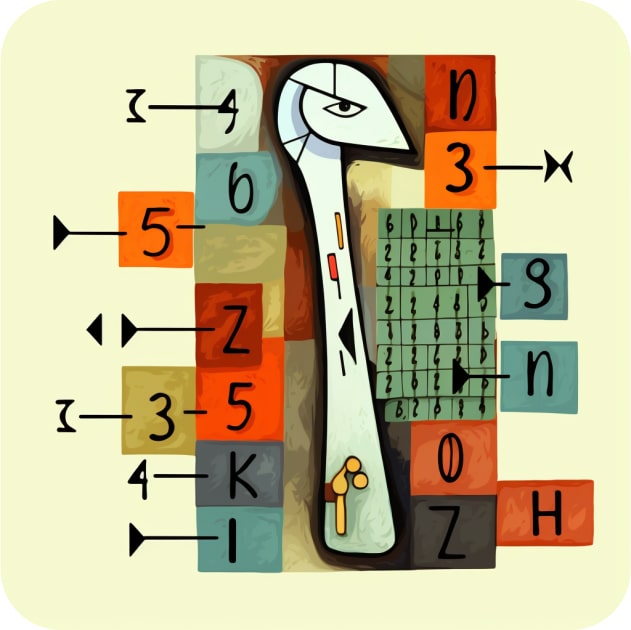
Filter
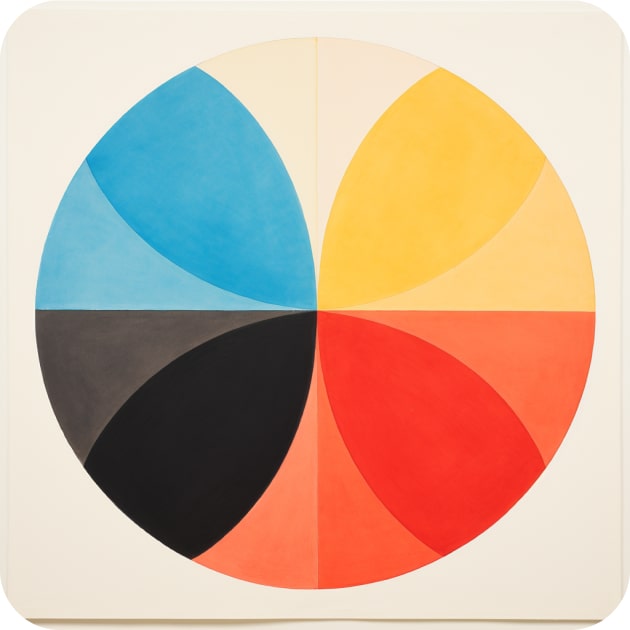
Fragment
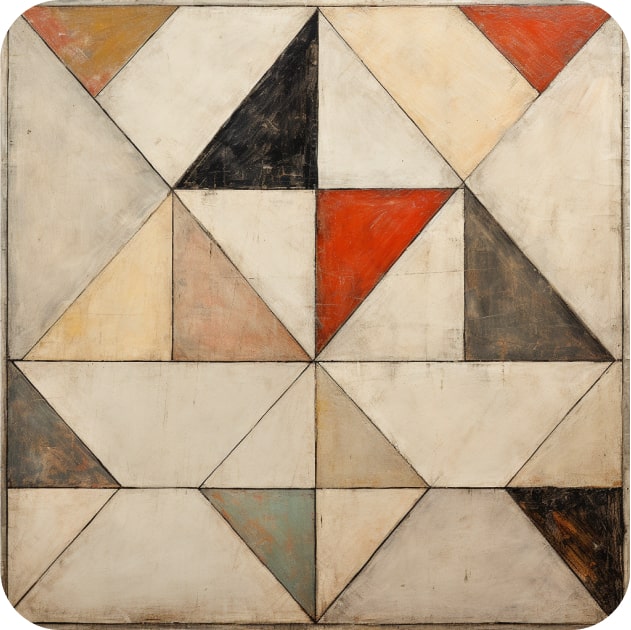
Homogenize
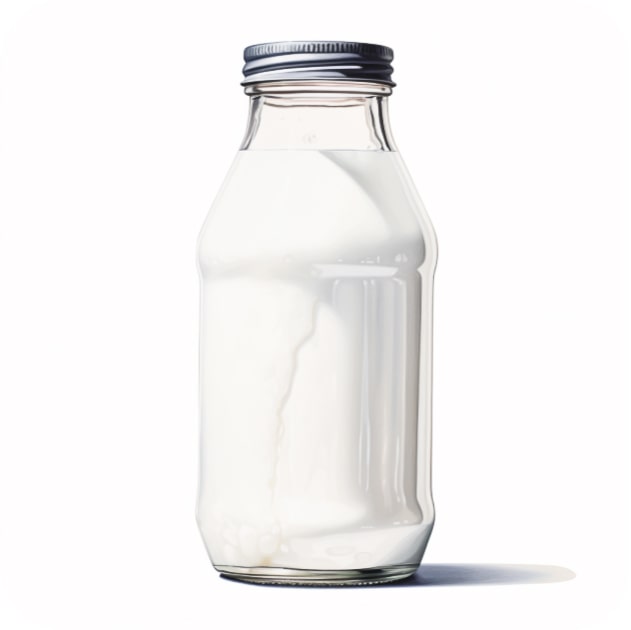
Impute
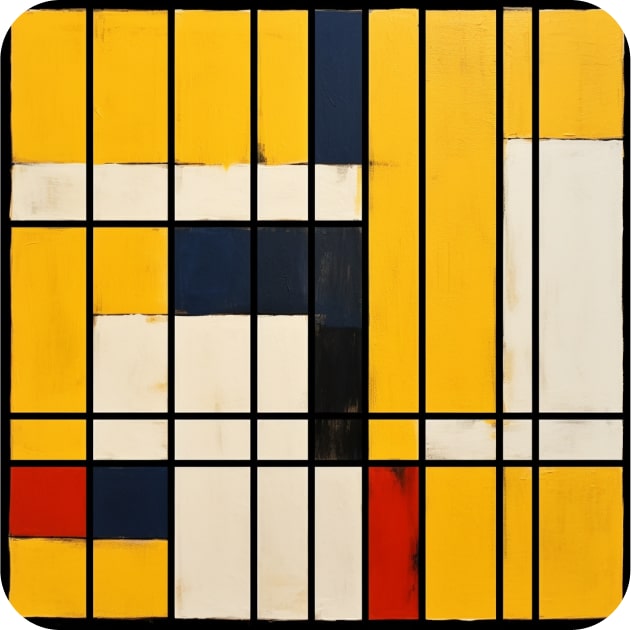
Linearize
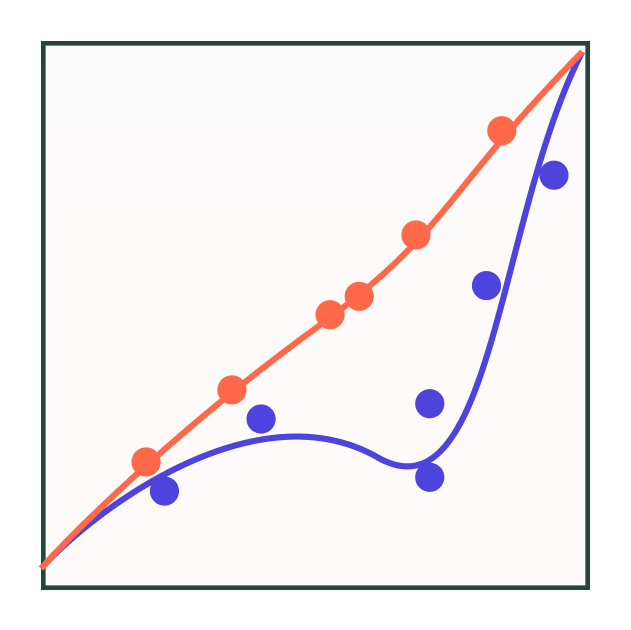
Munge
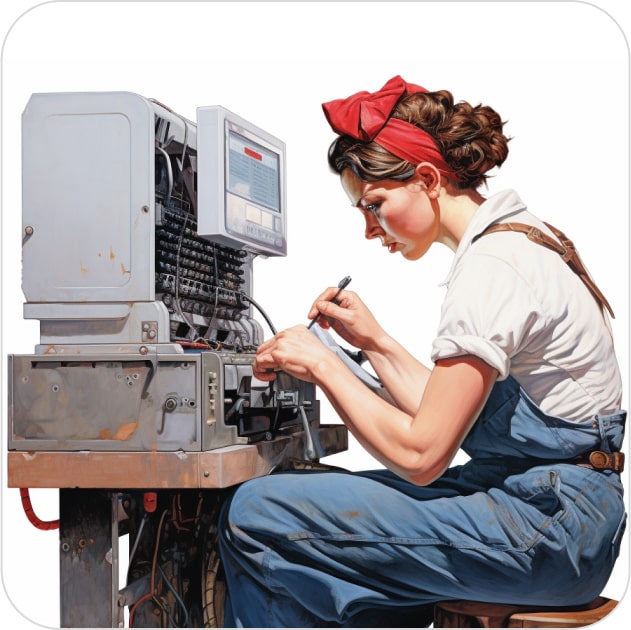
Normalize
Reshape
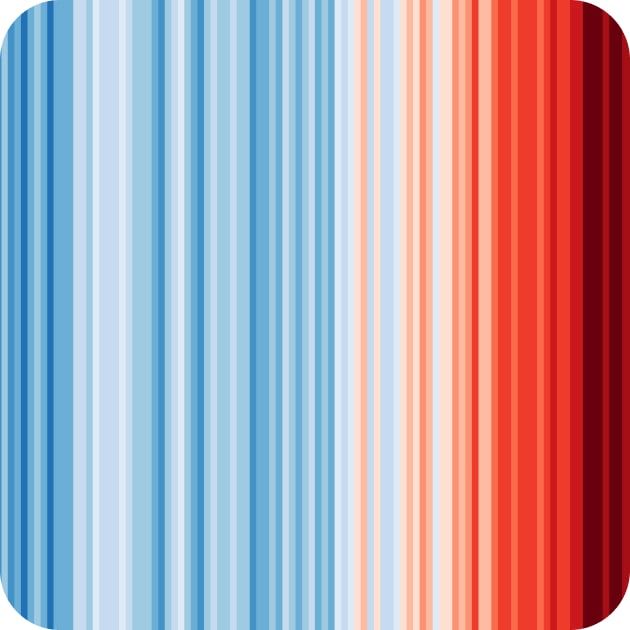
Serialize
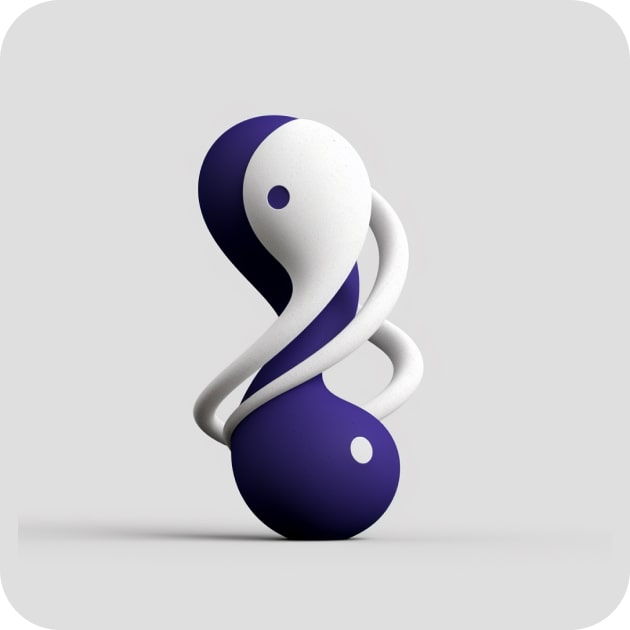
Shred
Skew
Split
Standardize
Tokenize
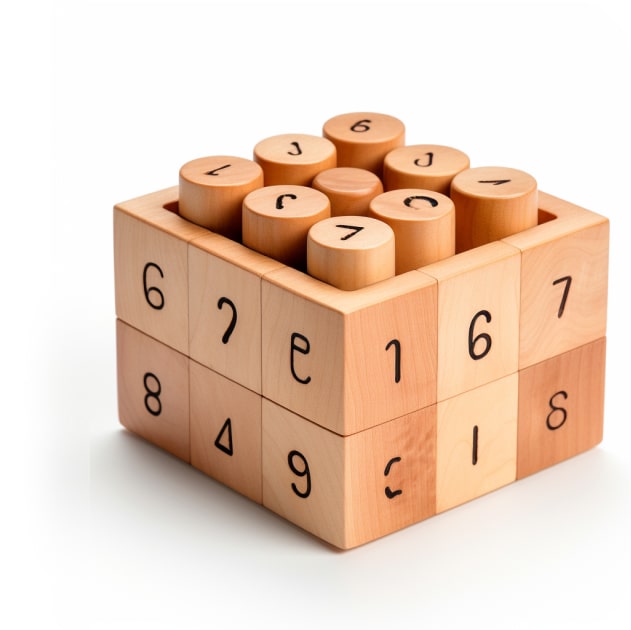
Transform
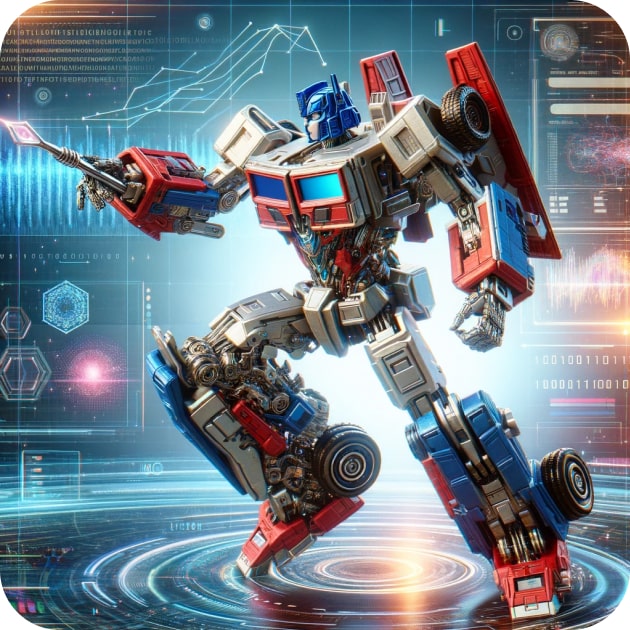
Wrangle
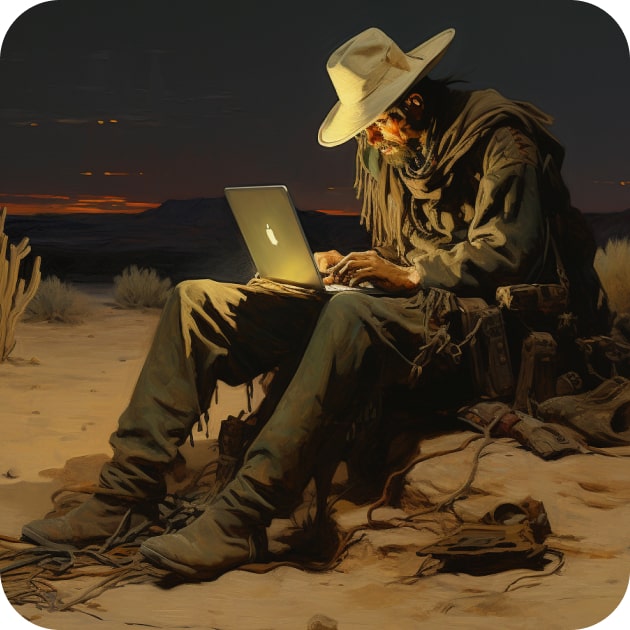