Data Shredding
Data shredding definition:
Data shredding, also known as data partitioning or data fragmentation, is the process of breaking down large datasets into smaller, more manageable pieces for easier processing and analysis. Shredding data can improve performance by allowing distributed processing of the smaller pieces, which can be performed in parallel, reducing the amount of time required to process the entire dataset. This approach is particularly useful for large datasets that cannot be processed on a single machine or within a single process.
As a side note, data shredding may, in a different context, refer to the process of securely erasing or destroying sensitive data that is no longer needed.
Data shredding example using Python:
In Python, data shredding can be achieved using various libraries, such as PySpark or Dask, which allow for distributed computing across multiple nodes or machines. For example, PySpark's repartition() function can be used to repartition a DataFrame into a specified number of partitions, enabling more efficient processing of large datasets. Please note that you need to have the necessary Python libraries installed in your Python environment to run this code.
Here's an example:
from pyspark.sql import SparkSession
# create a SparkSession object
spark = SparkSession.builder.appName("DataShreddingExample").getOrCreate()
# read in a large dataset
df = spark.read.format("csv").option("header", "true").load("large_dataset.csv")
# repartition the data into 10 partitions
df = df.repartition(10)
# perform some analysis on the data
result = df.groupBy("Col A","Col B").count().show()
# stop the SparkSession
spark.stop()
In this example, we start by creating a SparkSession object, then reading in a large dataset. We then repartition the data into 10 partitions using the repartition()
function, which creates 10 smaller, more manageable pieces of the data. We can then perform some analysis on the data, such as grouping by a column and counting the occurrences, before stopping the SparkSession.
For example, if the input file large_dataset.csv
contained two columns of 1000 random integers between 0 and 10, your results might look like this:
|Col A|Col B|count|
+-----+-----+-----+
| 9| 4| 11|
| 10| 5| 2|
| 9| 5| 8|
| 8| 2| 8|
| 7| 1| 11|
| 4| 1| 11|
| 4| 2| 12|
| 0| 6| 6|
| 5| 4| 8|
| 1| 4| 14|
| 0| 4| 5|
| 3| 8| 11|
| 4| 6| 8|
| 5| 1| 12|
| 5| 5| 8|
| 2| 8| 13|
| 1| 1| 13|
| 0| 1| 6|
| 10| 0| 2|
| 0| 5| 4|
+-----+-----+-----+
only showing top 20 rows
Align
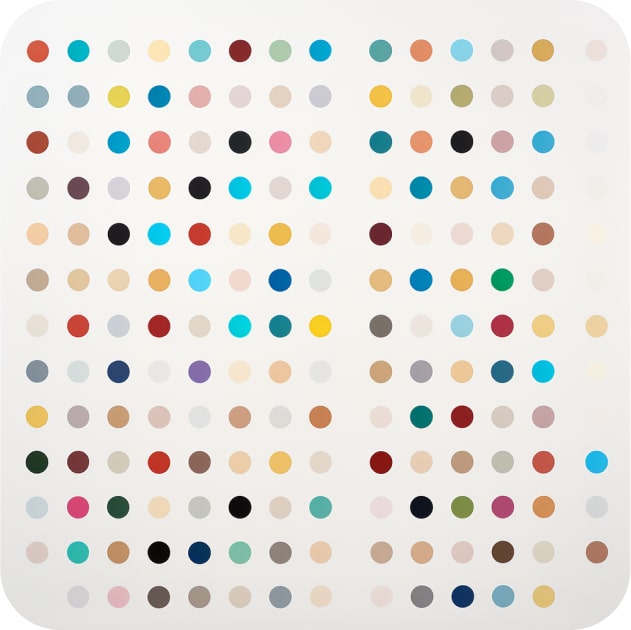
Clean or Cleanse
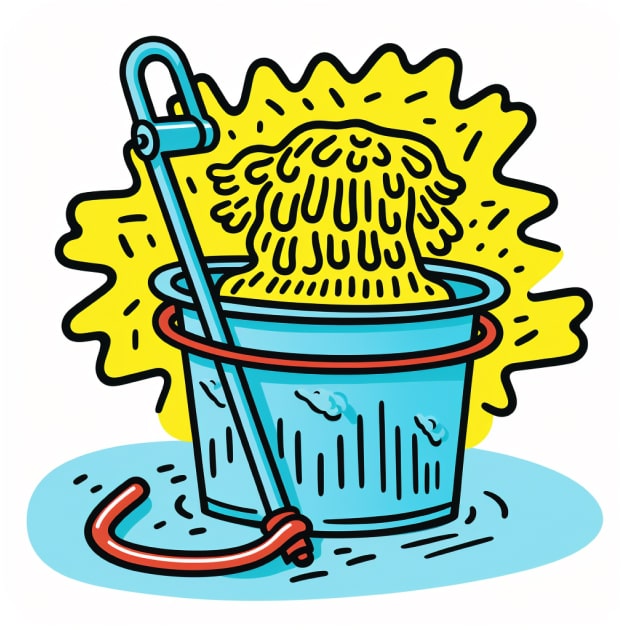
Cluster
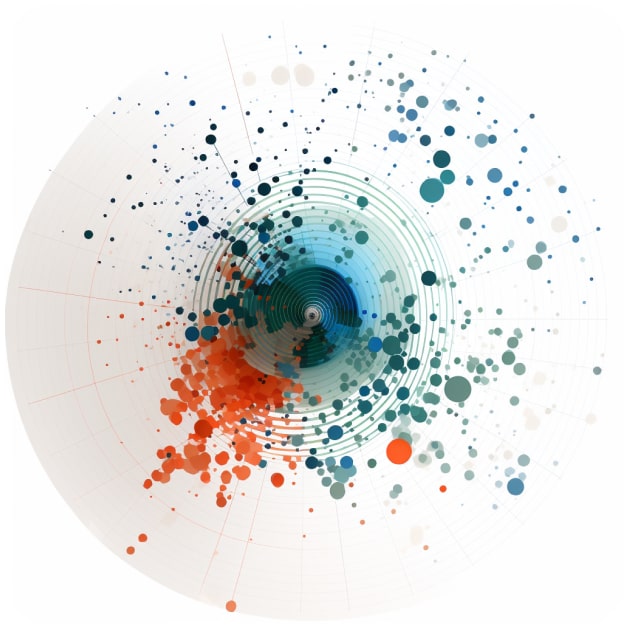
Curate
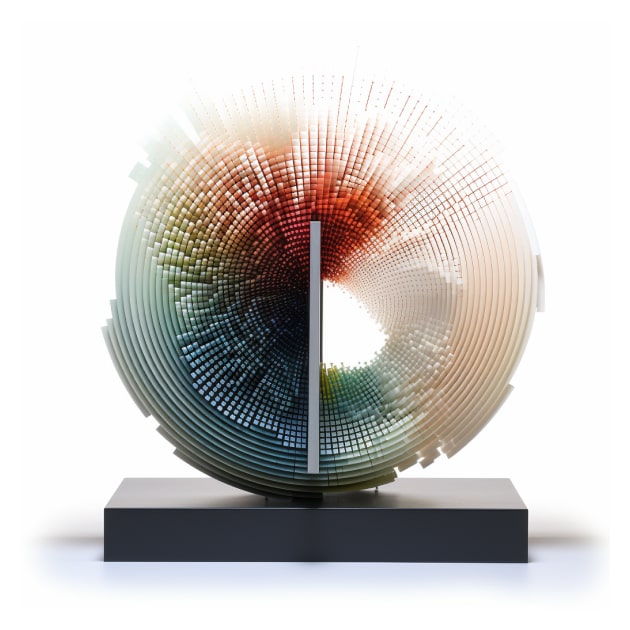
Denoise
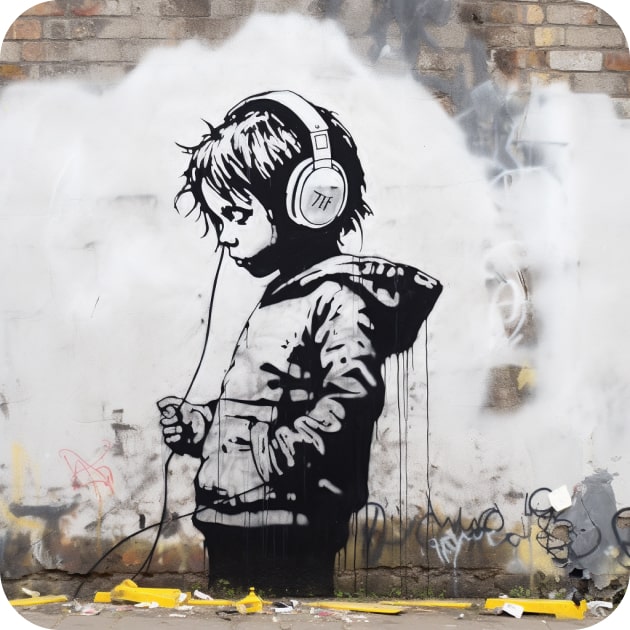
Denormalize
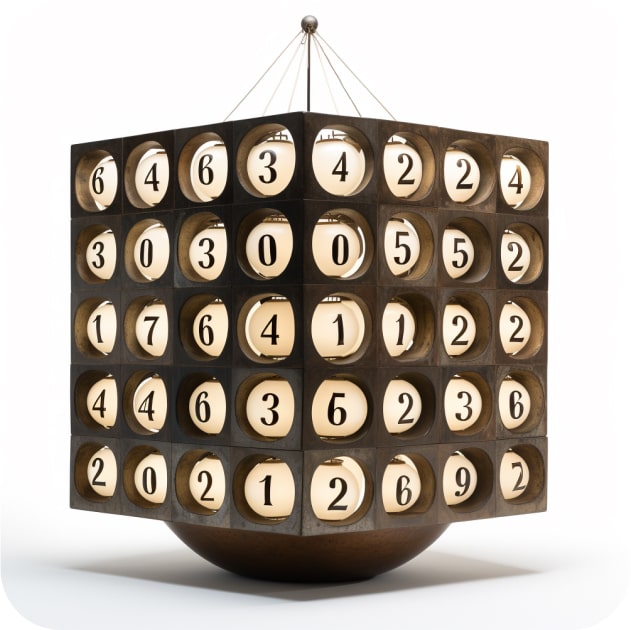
Derive
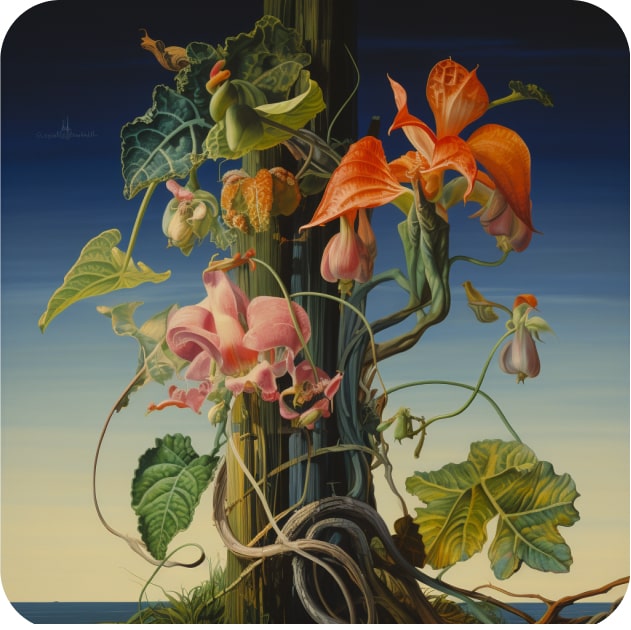
Discretize
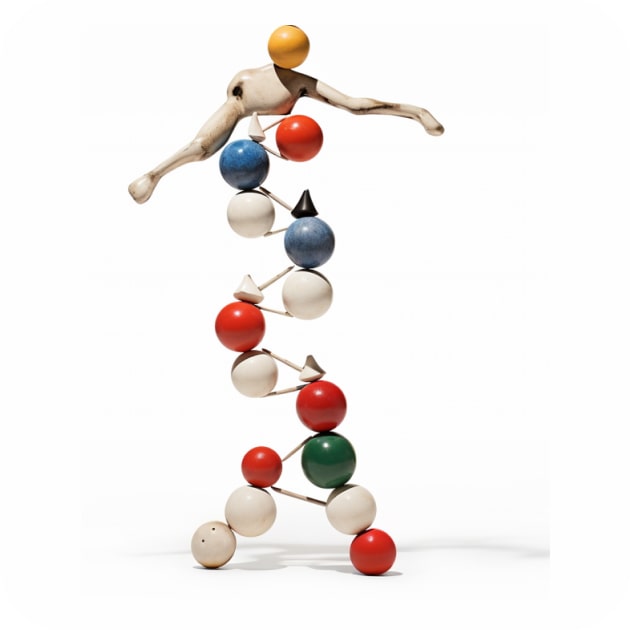
ETL
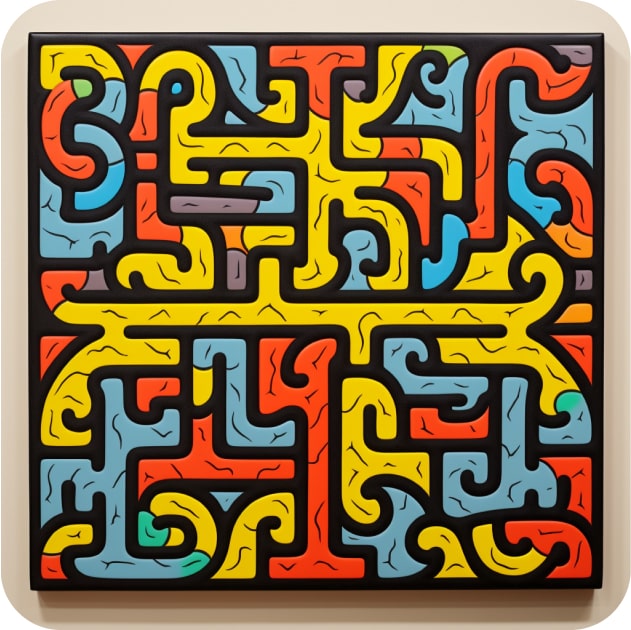
Encode
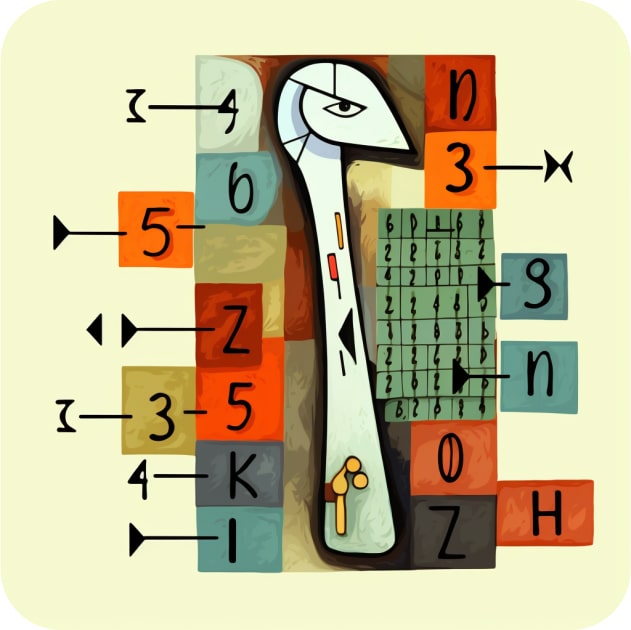
Filter
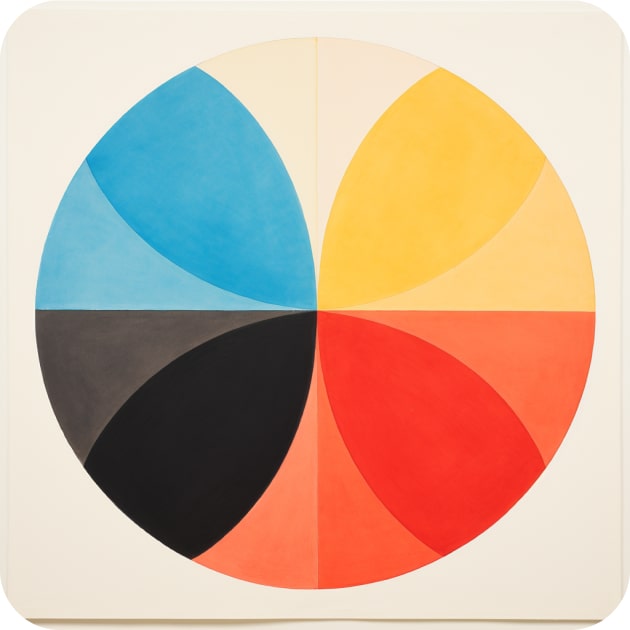
Fragment
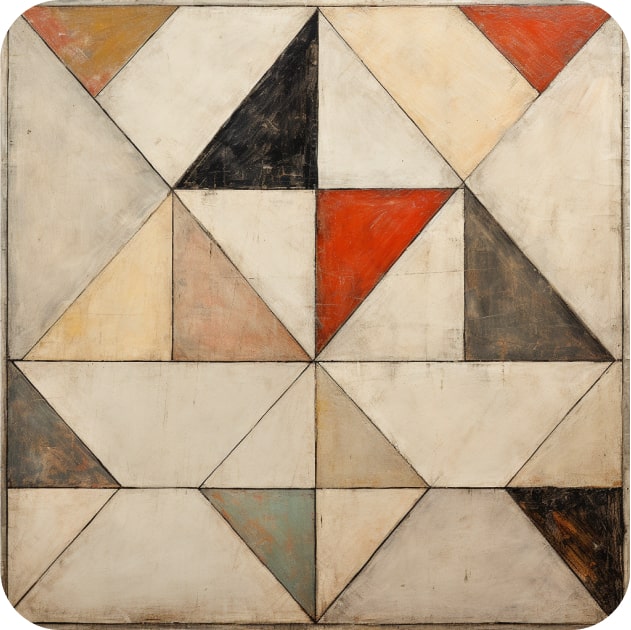
Homogenize
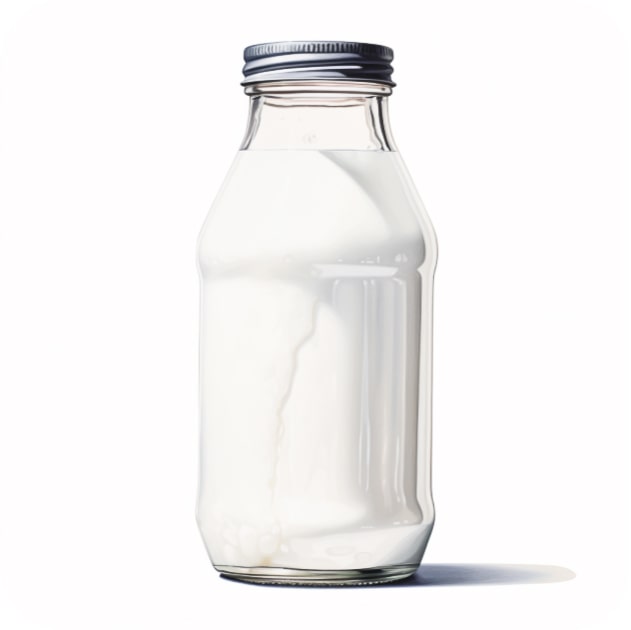
Impute
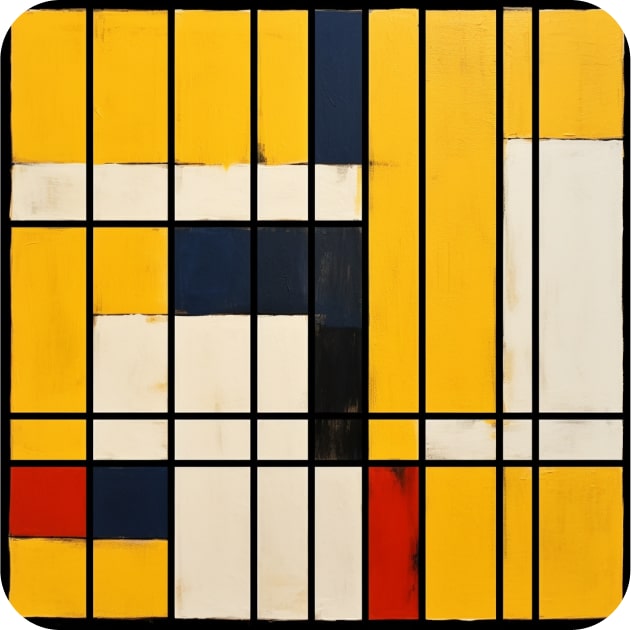
Linearize
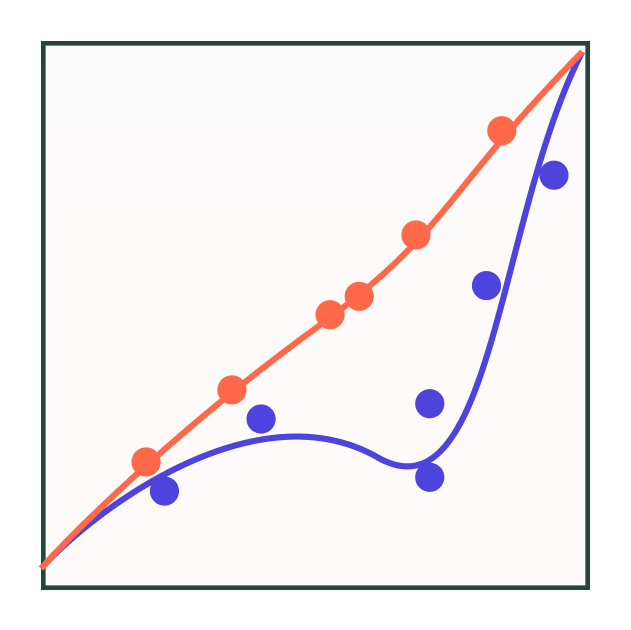
Munge
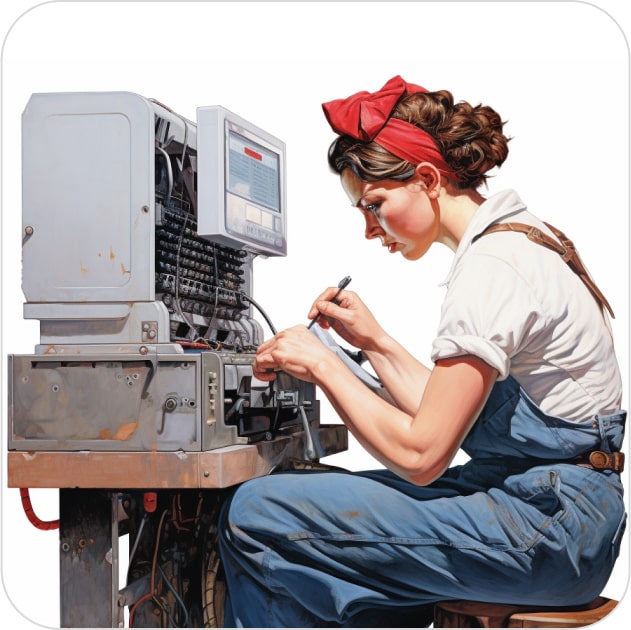
Normalize
Reduce
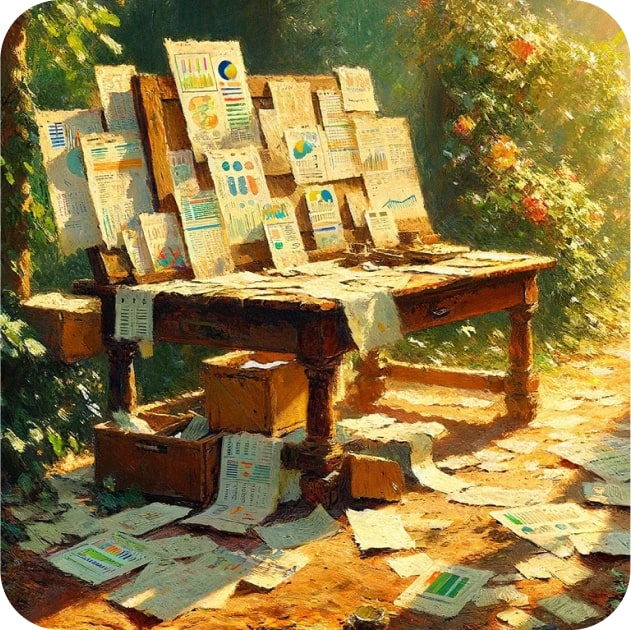
Reshape
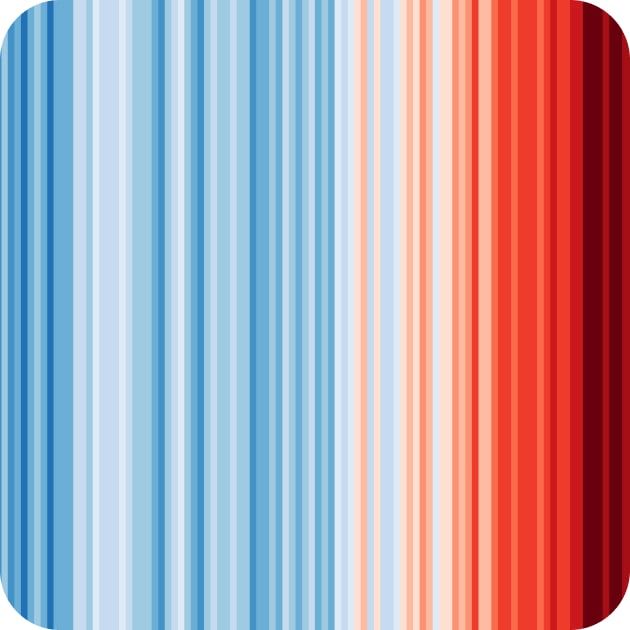
Serialize
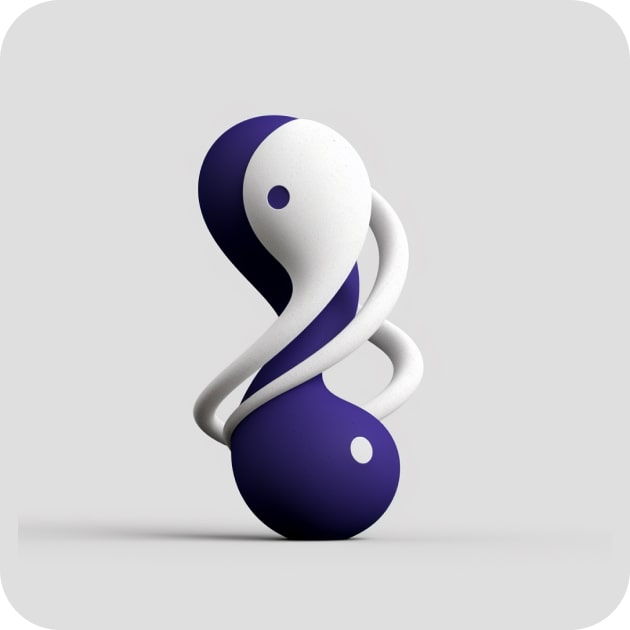
Skew
Split
Standardize
Tokenize
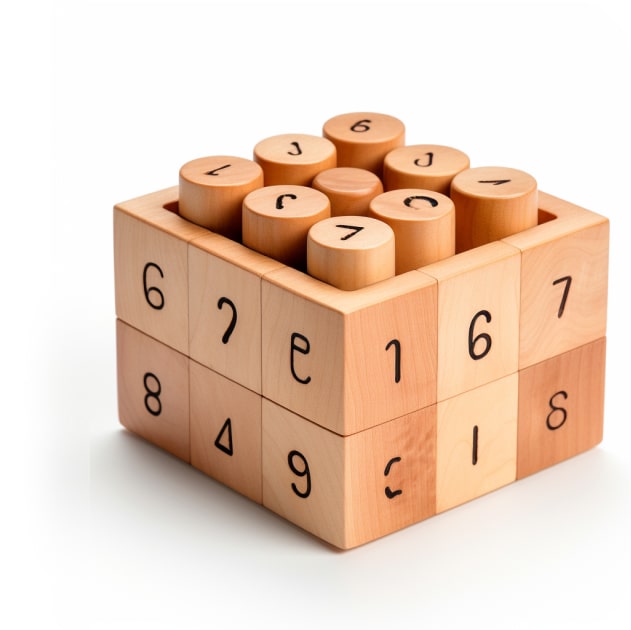
Transform
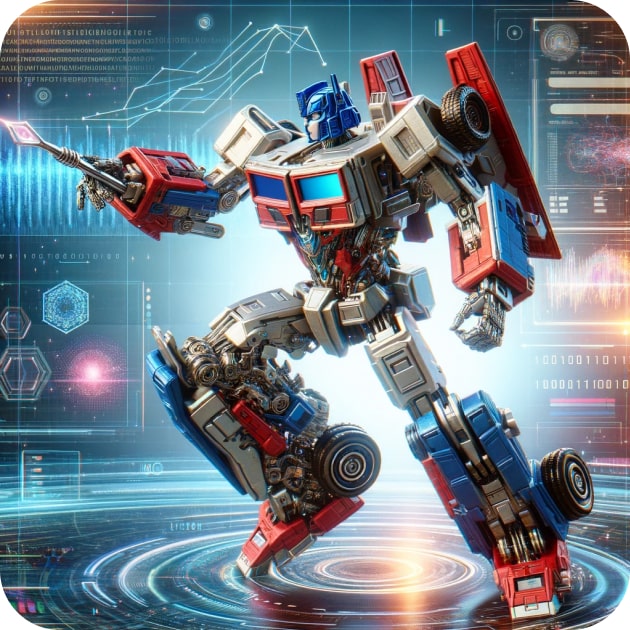
Wrangle
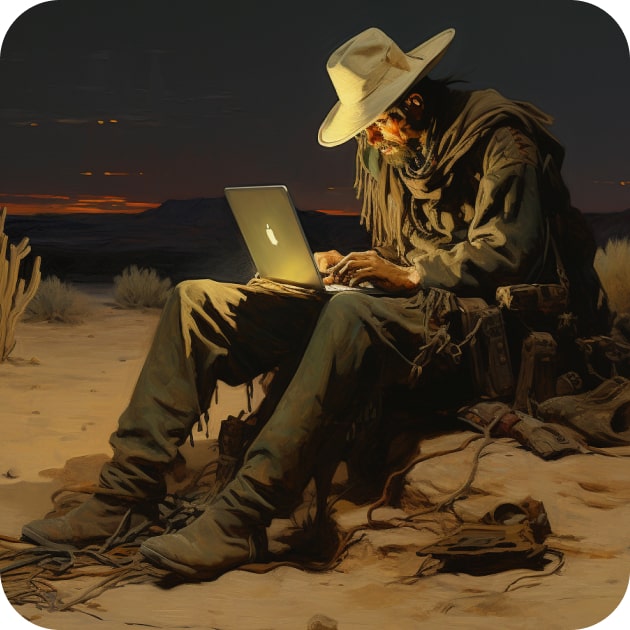