Dagster Data Engineering Glossary:
Secondary Index
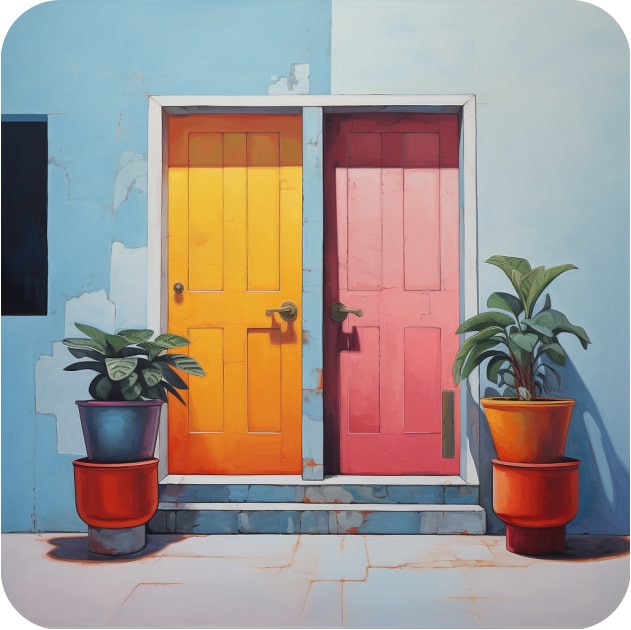
Secondary index definition:
In data engineering, a secondary index is an alternative way to access and search for data in addition to the primary index, which is typically based on the primary key of a table.
A secondary index can be a valuable data engineering technique that enhances the performance and flexibility of database queries. However, it should be used judiciously and selectively, considering the trade-offs involved in terms of maintenance and storage overhead.
Secondary index explained:
Here's a breakdown of the concept of a secondary index:
The primary index in a database is usually built on the primary key of a table. It ensures that rows in the table are physically stored in a way that allows for efficient retrieval based on the primary key values. Retrieving data using the primary key is typically fast and optimized, as the database engine knows exactly where to find the corresponding data.
A secondary index, on the other hand, is created on one or more columns other than the primary key columns of a table. Its purpose is to enable efficient searching and retrieval of data based on these secondary columns. In other words, it provides an additional path to access the data without having to perform a full table scan or a time-consuming query.
A table can have multiple "secondary" indexes. In fact, having multiple secondary indexes is a common practice in relational database systems to improve query performance.
Benefits of Secondary Indexes:
- Improved Query Performance: Secondary indexes speed up queries that involve filtering, sorting, or searching based on columns that are not part of the primary key.
- Reduced I/O Operations: Secondary indexes reduce the need for scanning the entire table, which can significantly reduce I/O operations and improve query response times.
- Flexibility: They allow for a more versatile querying capability, as you can search for data using various criteria that may not be part of the primary key.
- Supporting JOIN Operations: Secondary indexes can also be helpful when performing JOIN operations between tables, where the join condition involves columns other than the primary key.
The downsides of secondary indexing:
Secondary indexes require additional storage and maintenance, as they need to be updated whenever data in the indexed columns is inserted, updated, or deleted. This can lead to overhead in terms of storage and write operations. Consider carefully the columns to index as creating too many secondary indexes can lead to increased storage and maintenance costs.
Estimating the impact
The additional storage overhead introduced by secondary indexing in a database depends on various factors, including the database management system (DBMS) you are using, the number and size of indexed columns, and the number of rows in the table, etc.
This said, a common rule of thumb is that an index may require around 20-40% of the storage space of the data it indexes.
To get a more accurate estimate for your specific use case, you can use the following approach:
- Create a table with the data and columns you plan to index.
- Populate the table with representative data.
- Create the secondary index.
- Check the size of the index in your specific DBMS using built-in functions or tools provided by the DBMS itself.
So, when should we consider a secondary index? Let's look at some scenarios:
When to use a secondary index
Secondary indexes are most efficient in circumstances where they can significantly improve query performance and provide a valuable advantage in data retrieval. Here are some scenarios in which using a secondary index is highly beneficial:
- Frequent search queries on columns other than the primary key, such as filtering, sorting, or searching based on non-primary key attributes.
- Complex queries involving JOIN operations, WHERE clauses, or GROUP BY clauses on columns that are not part of the primary key.
- To improve read performance by avoiding full table scans.
- Ranges and range queries such as date ranges, numerical ranges, or alphabetical ranges.
- High selectivity i.e. the values in the column are relatively unique. High selectivity helps reduce the number of rows that need to be scanned for a specific value, making queries faster.
An example of secondary indexing in Python
To illustrate Secondary Indexing using Python, here is a simple example using PostgreSQL as our database, and using psycopg2, the most popular PostgreSQL database adapter for the Python programming language.
In this example, we'll create a table with a primary index on the "id" column and a secondary index on the "username" column for efficient username-based queries.
We achieve this with the PostgreSQL command CREATE INDEX <index_name> ON <table_name>
.
First, you'll need to install the psycopg2 library if you haven't already. You can install it using pip:
pip install psycopg2
Now, let's create a sample Python script to demonstrate the concept. Make sure to replace 'your_database_name'
, 'your_username'
, 'your_password'
, 'your_host'
, and 'your_port'
with the actual connection details for your PostgreSQL database.
If you have followed this guide, the example would use:
- 'dbname': 'dagsterdb',
- 'host': 'localhost',
- 'port': '5432',
- 'user': will be the one you retrieved with the command
whoami
- you can skip
'your_password'
if you have not set one.
import psycopg2
# Establish a connection to the PostgreSQL database
conn = psycopg2.connect(
host="host",
port="port",
database="dbname",
user="your_username",
password="your_password"
)
# Create a cursor object to interact with the database
cursor = conn.cursor()
# Create a table with a primary key and a secondary index
create_table_query = '''
CREATE TABLE band_members (
name VARCHAR(255),
role VARCHAR(50),
created_date DATE
);
'''
# Execute the create table query
cursor.execute(create_table_query)
# Create a secondary index on the 'role' column
create_index_query = '''
CREATE INDEX role_index ON band_members(role);
'''
# Execute the create index query
cursor.execute(create_index_query)
# Insert some sample data into the table
insert_data_query = '''
INSERT INTO band_members (name, role, created_date) VALUES
('Thom Yorke', 'vocals', '1968-10-07'),
('Jonny Greenwood', 'guitar', '1971-10-05'),
('Ed OBrien', 'guitar', '1968-04-15'),
('Philip Selway', 'drums', '1967-05-23'),
('Colin Greenwood', 'bass', '1969-06-26');
'''
cursor.execute(insert_data_query)
# Query data using the secondary index
search_role = 'guitar'
select_query = '''
SELECT * FROM band_members WHERE role = %s
'''
cursor.execute(select_query, (search_role,))
users = cursor.fetchall()
if users:
print(f"Radiohead band members with a role of '{search_role}' found:")
for user in users:
print(f"Name: {user[0]}, Role: {user[1]}, Date of birth: {user[2]}")
else:
print(f"No users with username '{search_role}' found")
# Close the cursor and the database connection
cursor.close()
conn.close()
This example will return:
Band members with a role of 'guitar' found:
Name: Jonny Greenwood, Role: guitar, Date of birth: 1971-10-05
Name: Ed OBrien, Role: guitar, Date of birth: 1968-04-15
Append
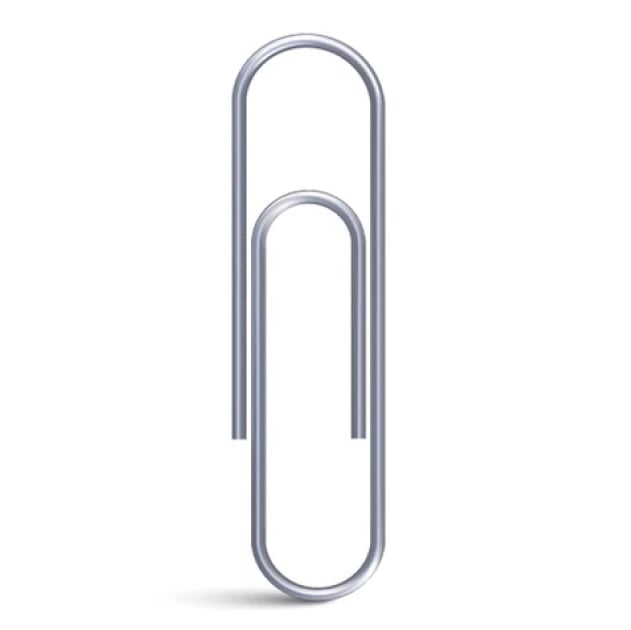
Archive
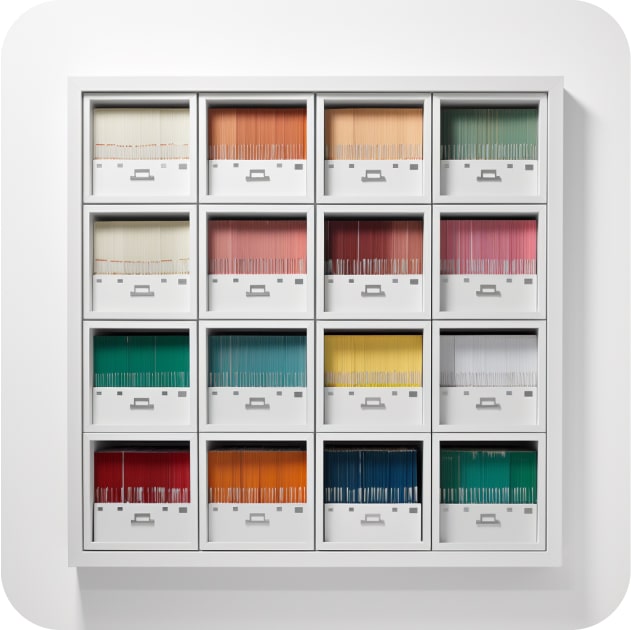
Augment
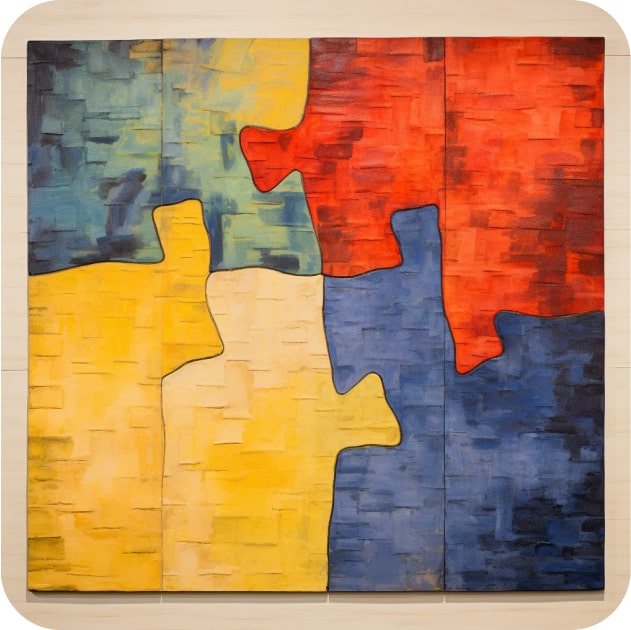
Auto-materialize
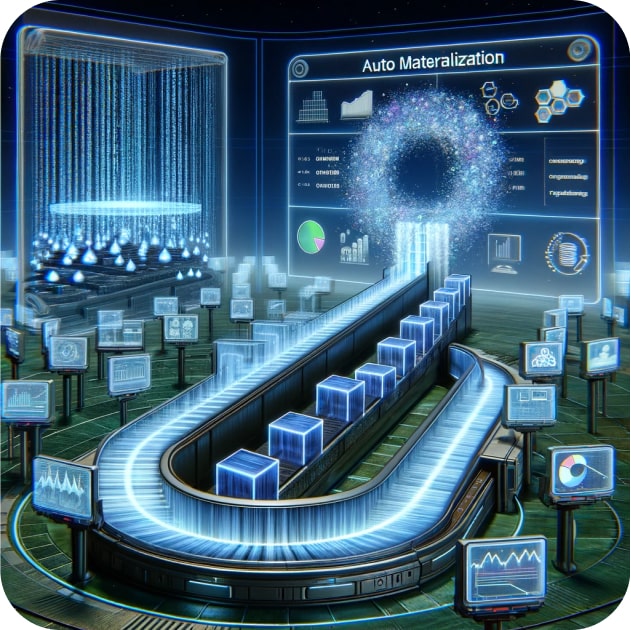
Backup
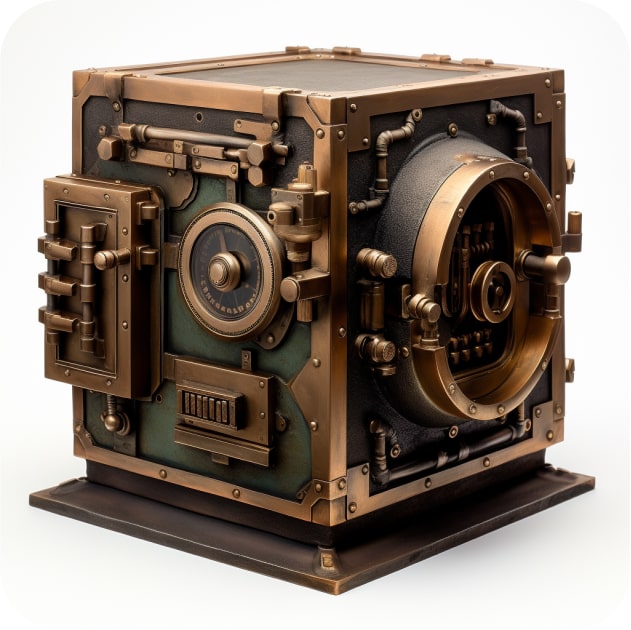
Batch Processing
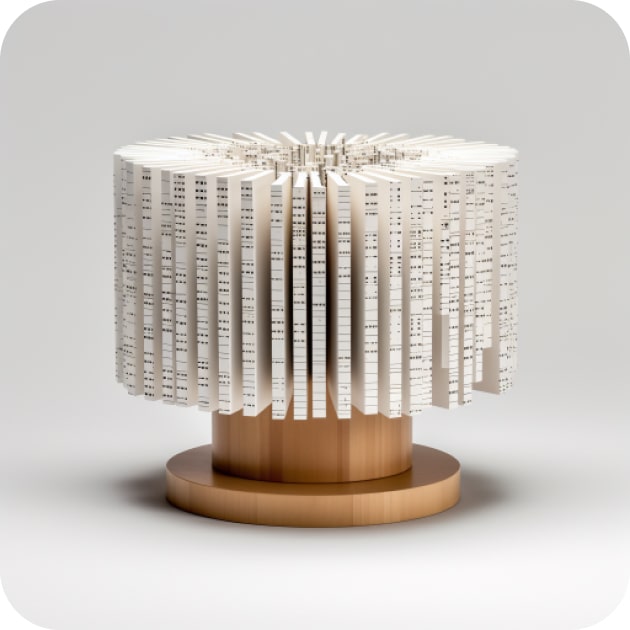
Cache
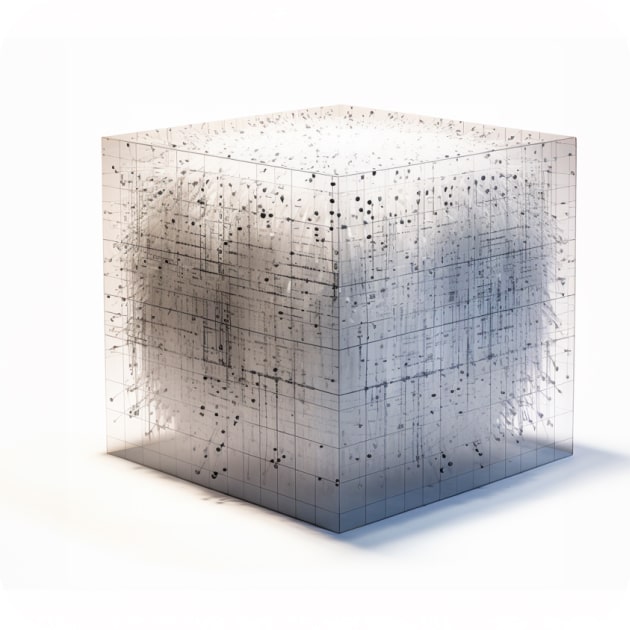
Categorize
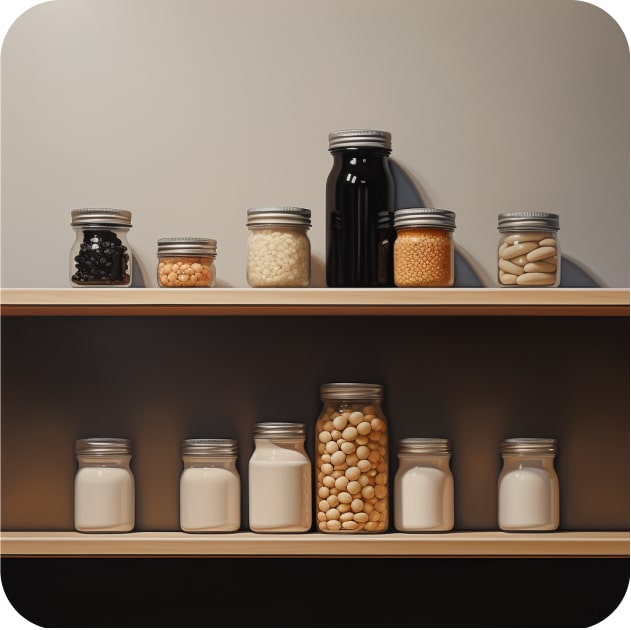
Checkpointing
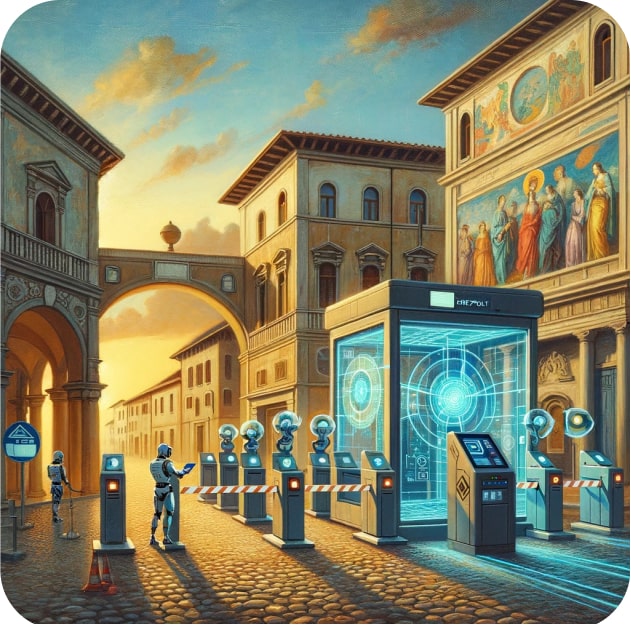
Deduplicate
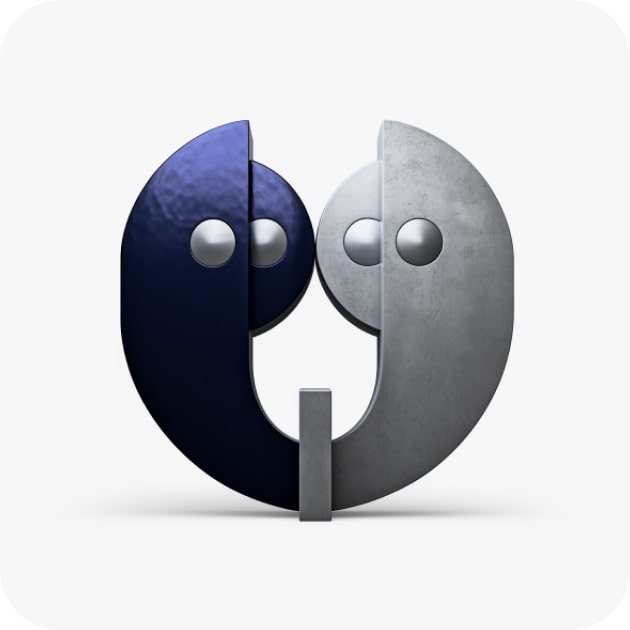
Deserialize
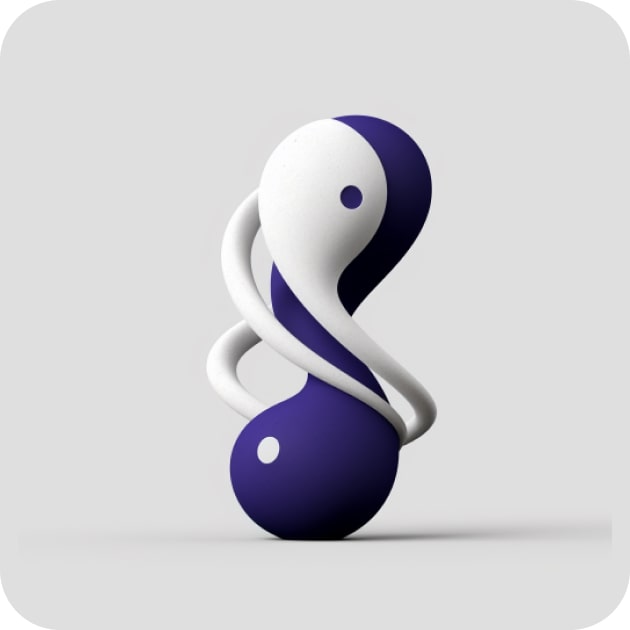
Dimensionality
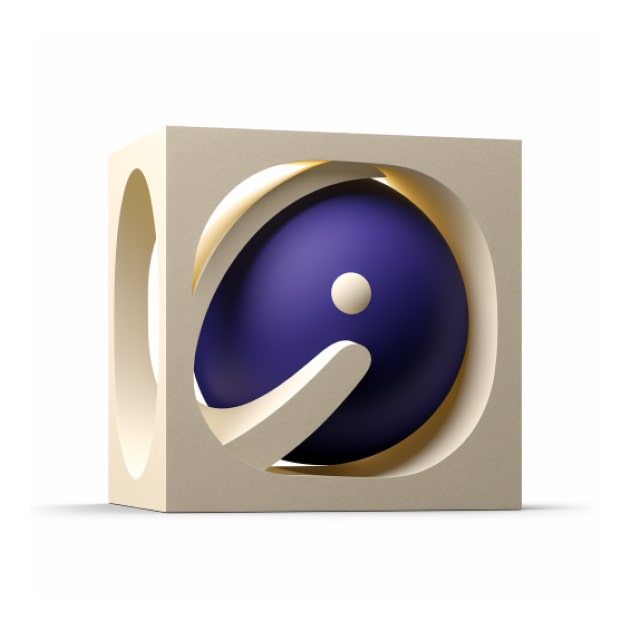
Encapsulate
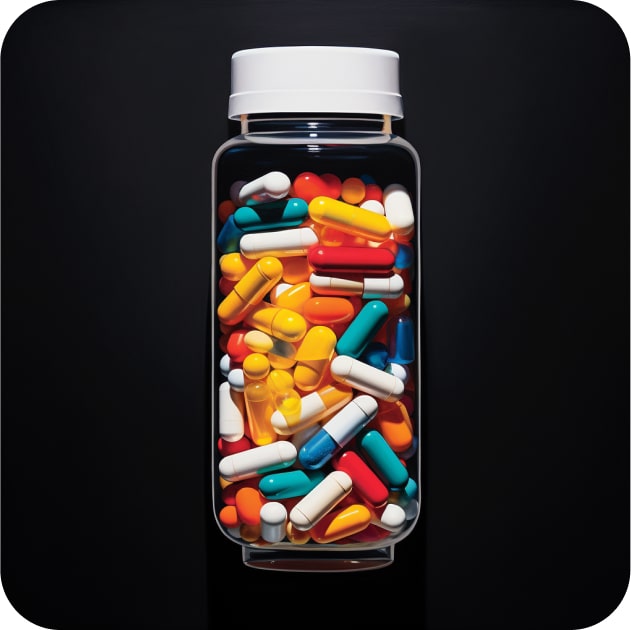
Enrich
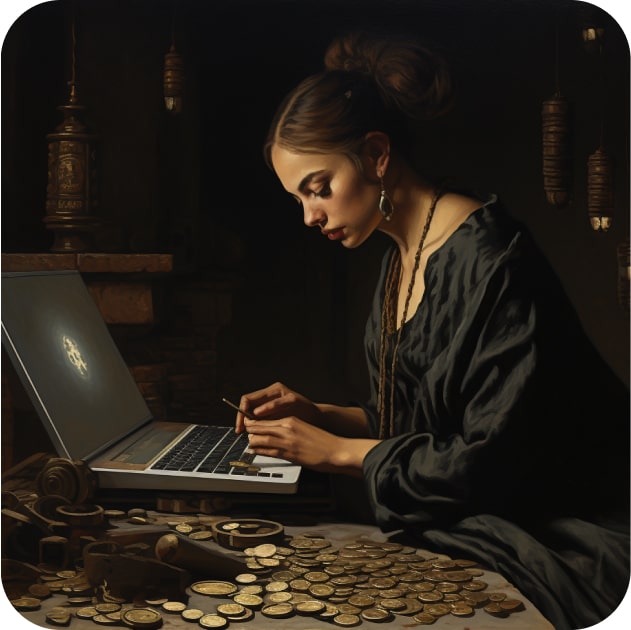
Export
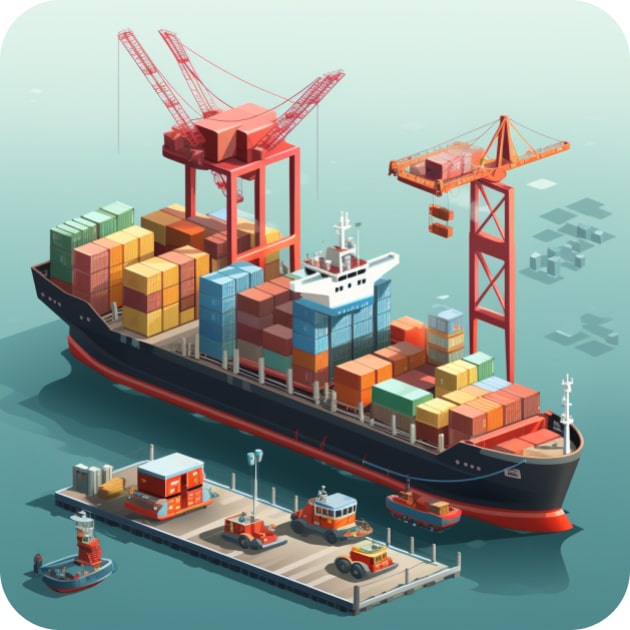
Graph Theory
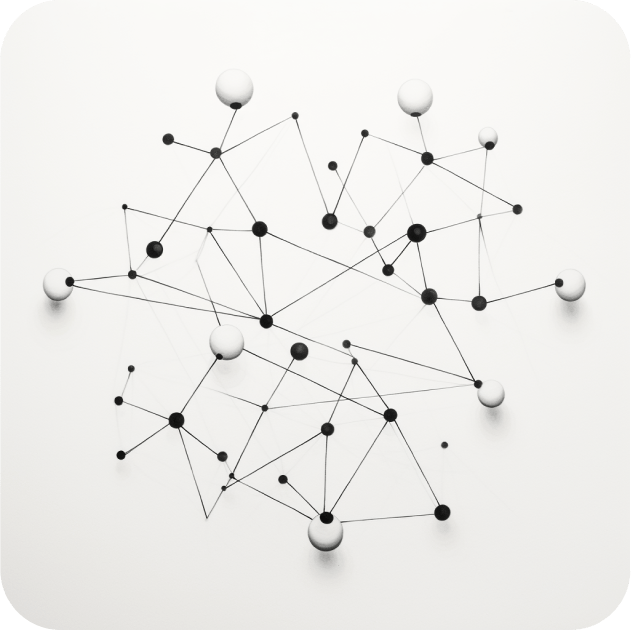
Idempotent
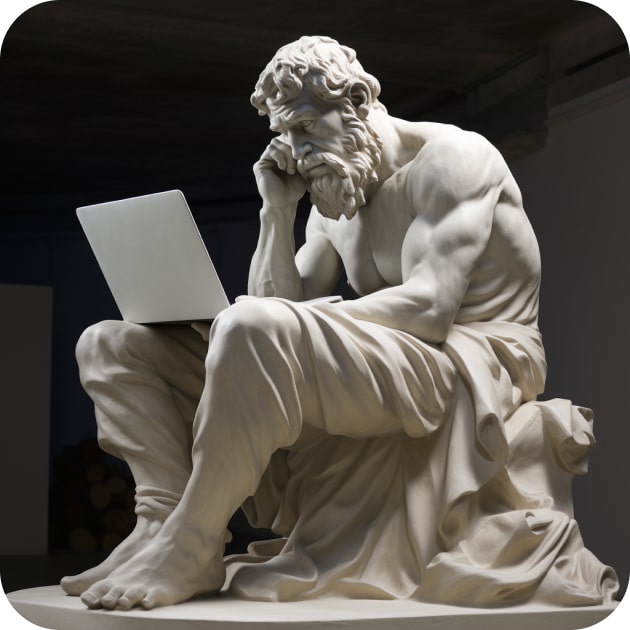
Index
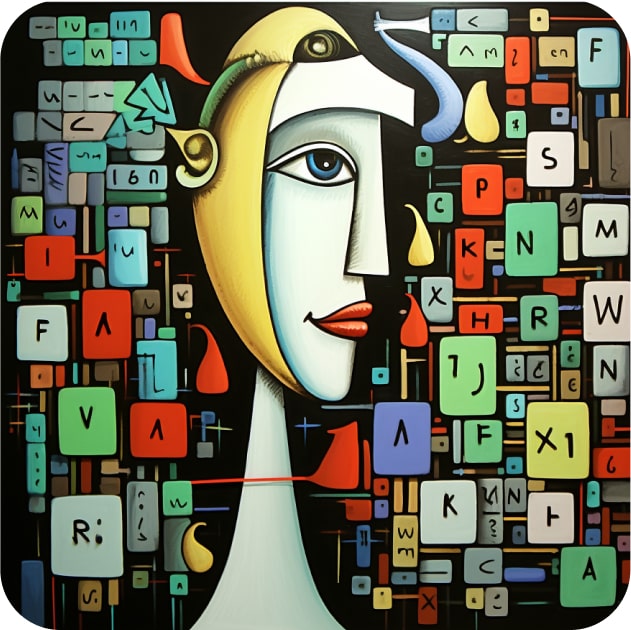
Integrate
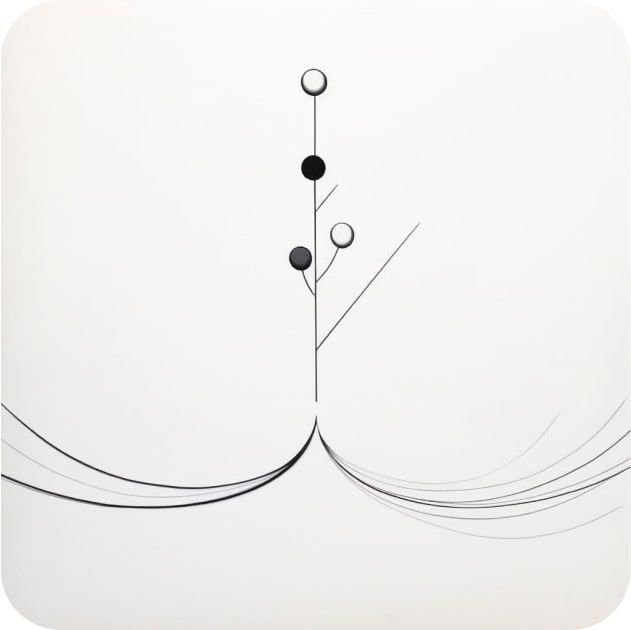
Lineage
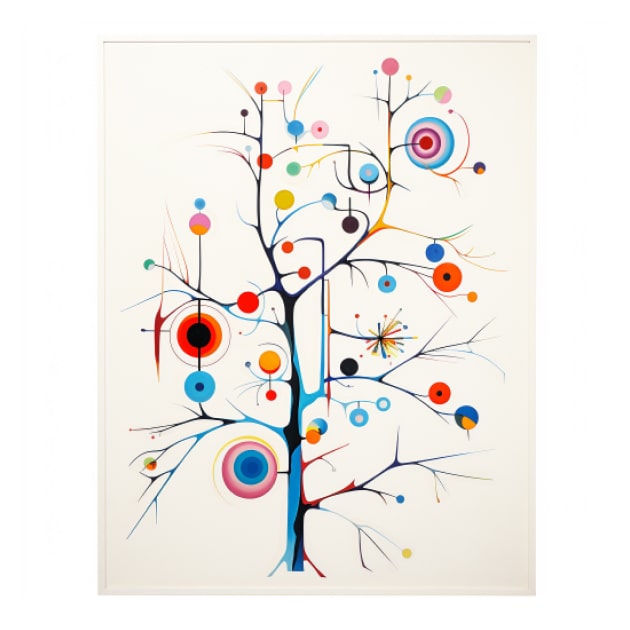
Linearizability
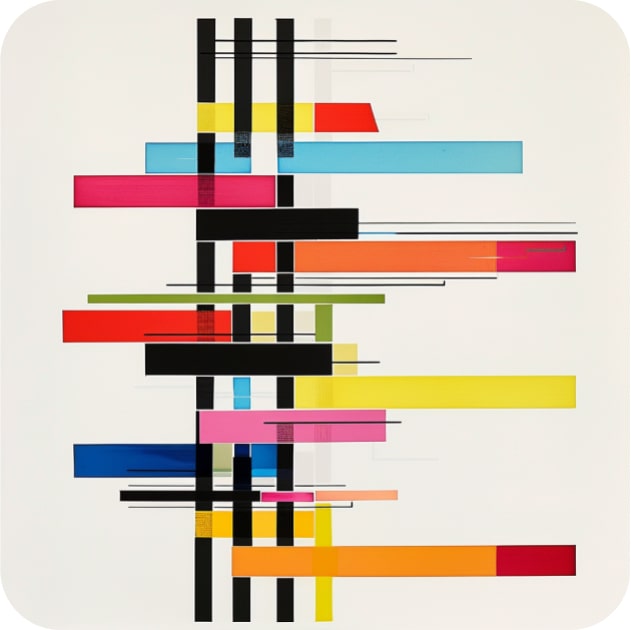
Materialize
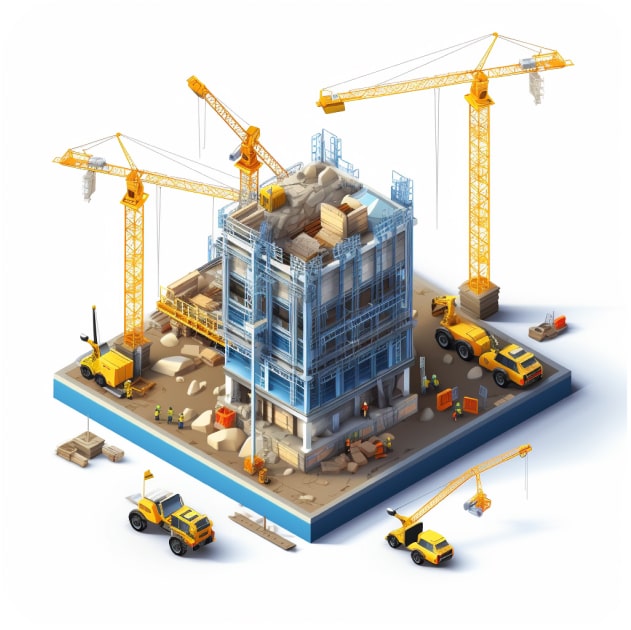
Memoize
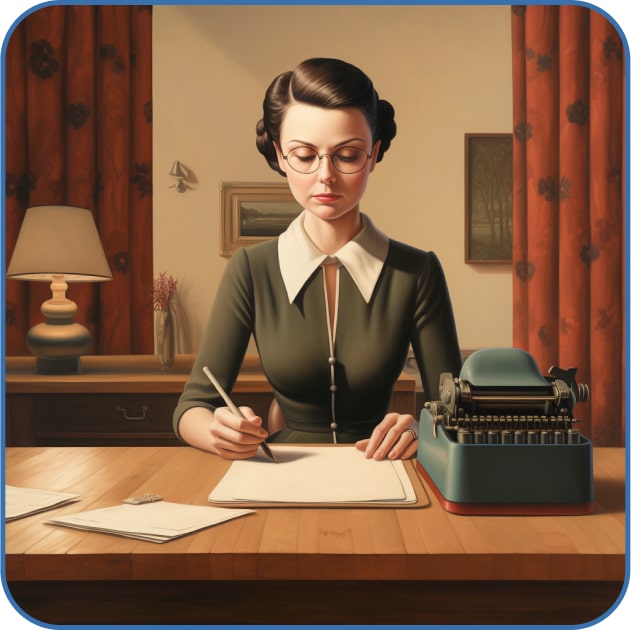
Merge
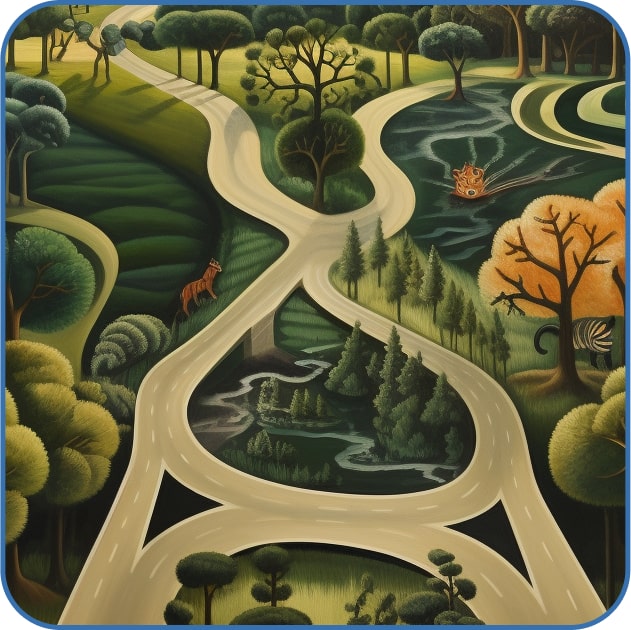
Model
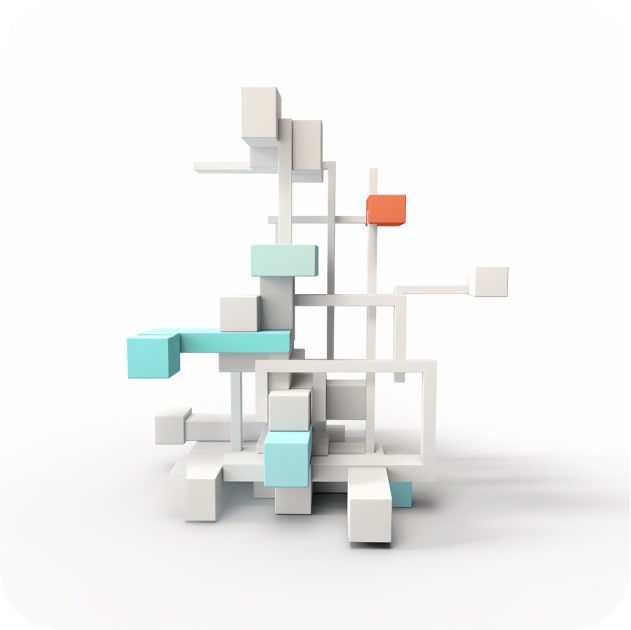
Monitor
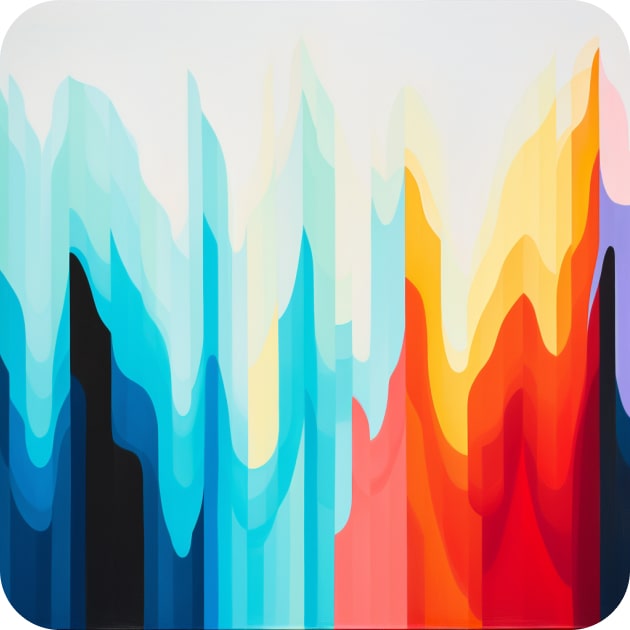
Named Entity Recognition
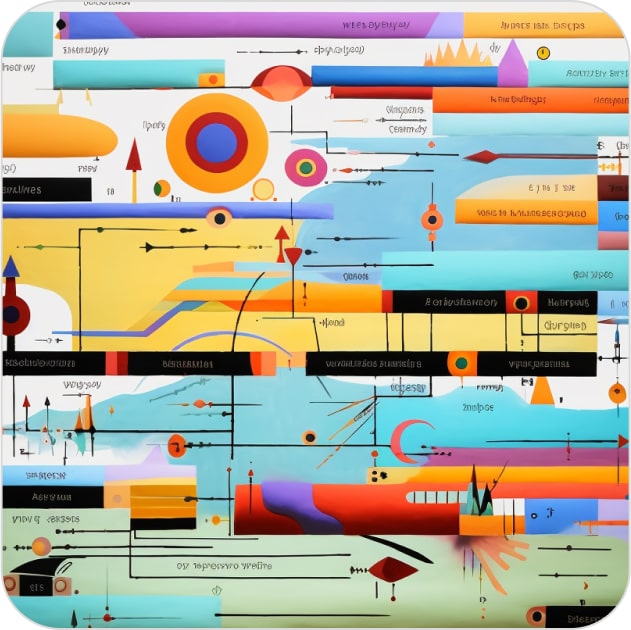
Parse
Partition
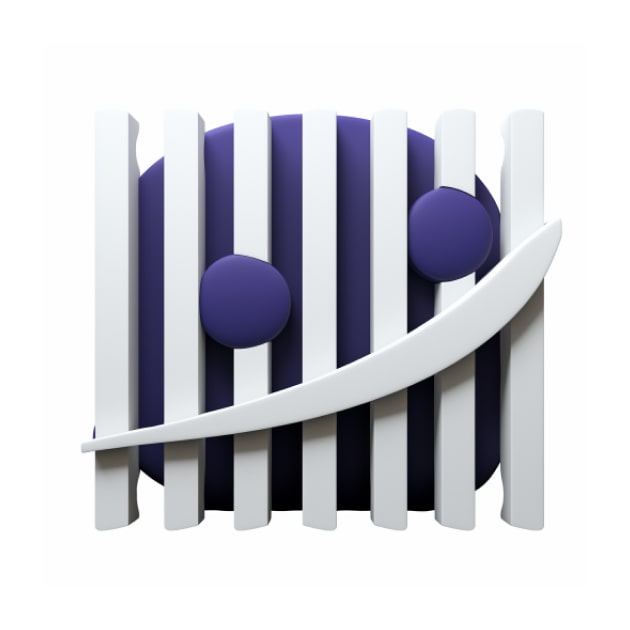
Prep
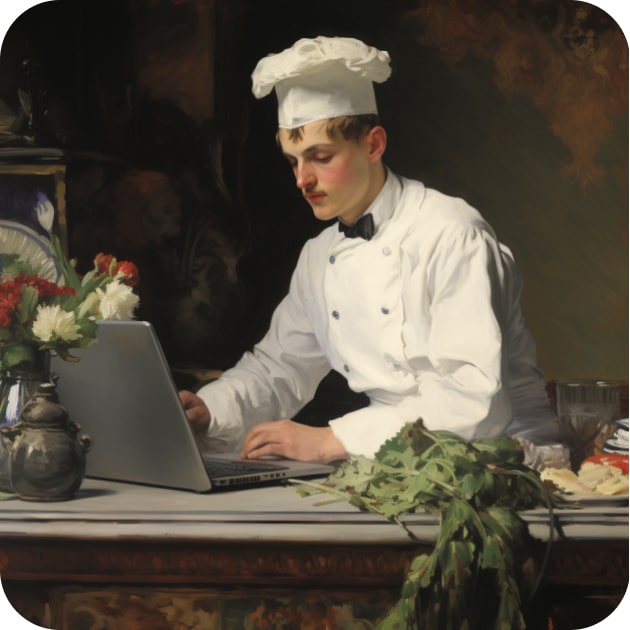
Preprocess
Primary Key
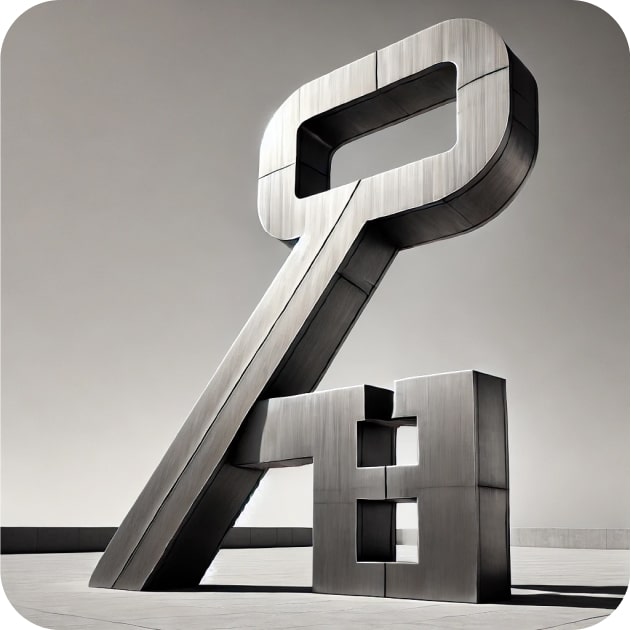
Replicate
Scaling
Schema Inference
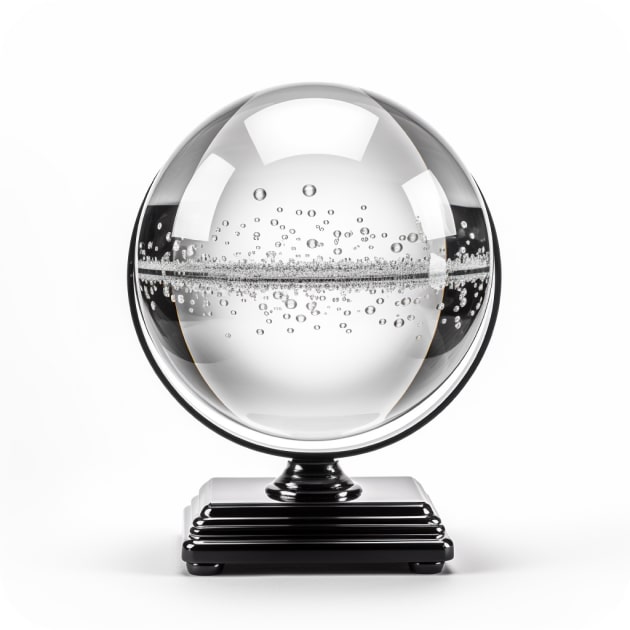
Schema Mapping
Software-defined Asset
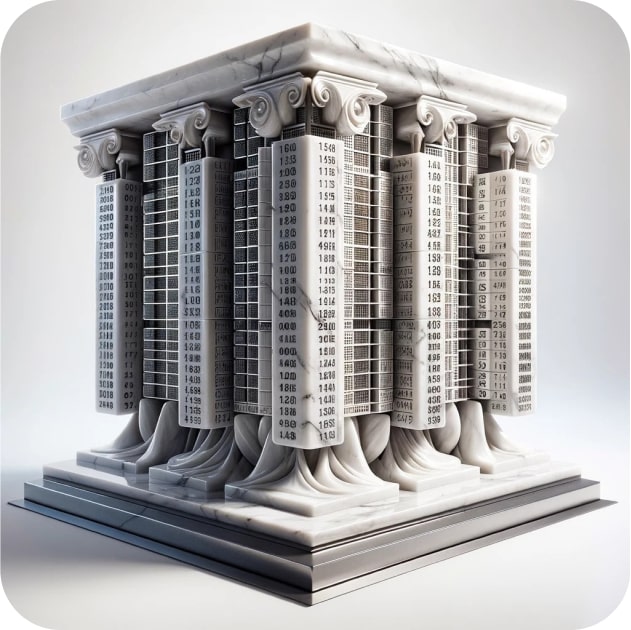
Synchronize
Validate
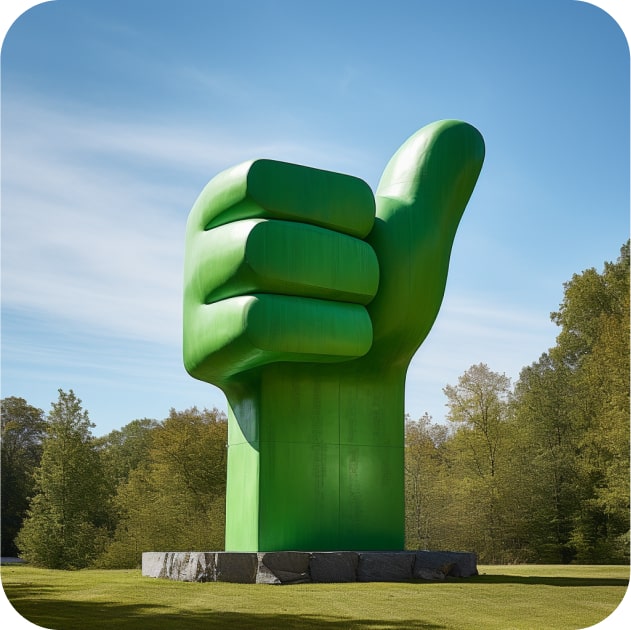
Version
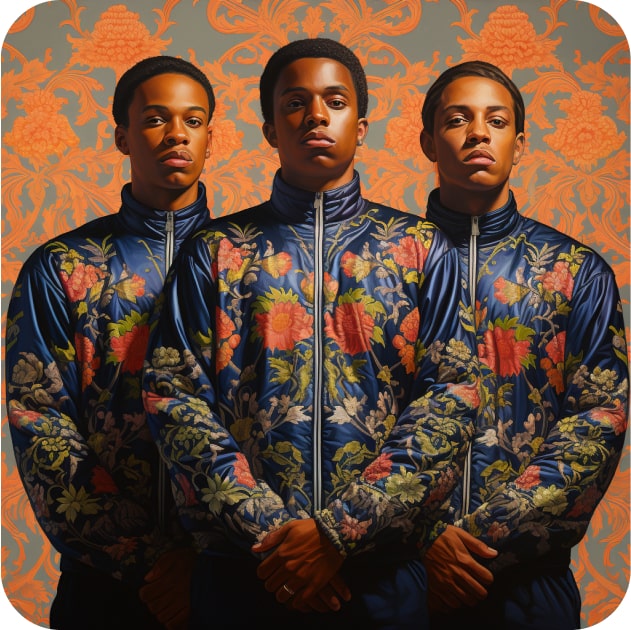