Dagster Data Engineering Glossary:
Data Dimensionality
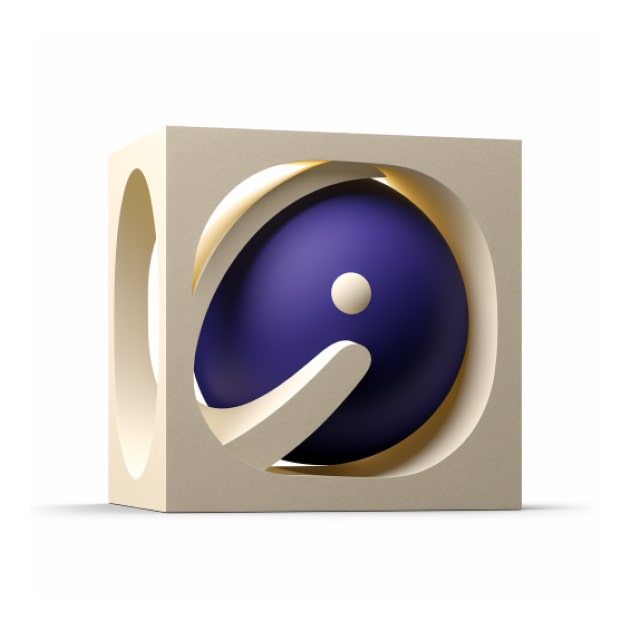
Data dimensionality definition:
Dimensionality in data engineering pipelines refers to the number of features or attributes that are present in the data as it moves through the pipeline. In data engineering, it is important to consider dimensionality as a key factor in designing and building data pipelines because high-dimensional data can have significant impacts on pipeline performance, storage requirements, and processing times.
For example, if you are working with a large dataset that contains thousands or millions of rows and hundreds or thousands of columns, it can be challenging to move this data efficiently through a pipeline. Large datasets with high dimensionality can also require more storage space and processing power, which can increase the cost and time required to manage the data.
To address the challenge of high-dimensional data in data engineering pipelines, it is common to use techniques such as feature selection, feature extraction, and dimensionality reduction. These techniques can reduce the number of features in the data, making it more manageable and easier to process.
Data dimensionality in Python:
Please note that you need to have the necessary Python libraries installed in your Python environment to run the code examples below.
Here is a very basic example Python code for evaluating dimensionality (in this case just number of rows and columns) in a dataset using the pandas library, assuming an input file dataset.csv
:
import pandas as pd
# Load dataset into a pandas DataFrame
df = pd.read_csv('dataset.csv')
# Get the number of rows and columns in the dataset
num_rows = df.shape[0]
num_cols = df.shape[1]
# Print the dimensionality of the dataset
print('Number of rows:', num_rows)
print('Number of columns:', num_cols)
Depending on your input file, this would output something like:
Number of rows: 16
Number of columns: 3
In this very basic example, we first load the dataset into a pandas DataFrame using the read_csv()
function. Then, we use the shape attribute of the DataFrame to get the number of rows and columns in the dataset. Finally, we print the dimensionality of the dataset by outputting the number of rows and columns using the print()
function.
This code can be used to quickly evaluate the dimensionality of a dataset and gain insight into its structure. If you need to perform further analysis on the data, you may want to use additional techniques such as visualization, statistical analysis, or machine learning algorithms to better understand the relationships between the features in the dataset.
A more advanced example of analyzing dimensionality of data using Python and sklearn:
Let’s look at a more advanced example using sklearn
.
By exploring multiple dimensions of the data using techniques like PCA and data visualization, we can gain a more complete understanding of the data and make better decisions about how to preprocess and analyze it.
from sklearn.datasets import load_iris
from sklearn.decomposition import PCA
# Load the iris dataset
iris = load_iris()
X = iris.data
# Perform Principal Component Analysis (PCA) to reduce the dimensionality of the dataset
pca = PCA(n_components=4)
X_pca = pca.fit_transform(X)
# Print the variance explained by each principal component
print('Explained variance:', pca.explained_variance_ratio_)
# Print the reduced dimensionality of the dataset
print('Number of rows:', X_pca.shape[0])
print('Number of columns:', X_pca.shape[1])
# Plot the first two principal components against each other
import matplotlib.pyplot as plt
plt.scatter(X_pca[:, 0], X_pca[:, 1], c=iris.target)
plt.title("My Dimensionality Example")
plt.xlabel('Principal Component 1')
plt.ylabel('Principal Component 2')
plt.show()
In this example, we still use the samel “iris dataset” from scikit-learn (literally a dataset of the characteristics of Iris flowers) and perform PCA to reduce the dimensionality of the dataset. We set n_components
to 4, which means we're keeping all four dimensions of the original data.
After computing the principal components, we print the explained variance ratio of each principal component to see how much of the variance in the original dataset is captured by each component. This gives us an idea of which dimensions are most important for describing the data.
Next, we plot the first two principal components against each other using matplotlib. This allows us to visualize the data in a two-dimensional space and see how well the different classes of flowers are separated from each other. This can help us gain a better understanding of the underlying structure of the data and identify any patterns or relationships that exist.
You will see the following output in the terminal:
Explained variance: [0.92461872 0.05306648 0.01710261 0.00521218]
Number of rows: 150
Number of columns: 4
Append
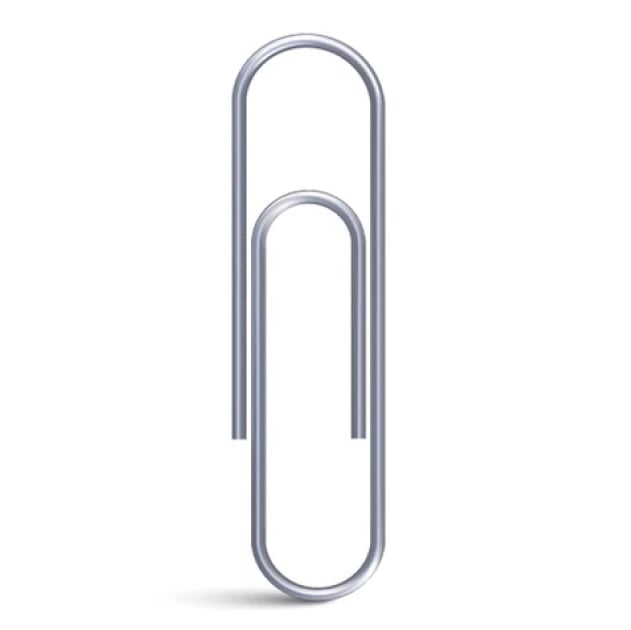
Archive
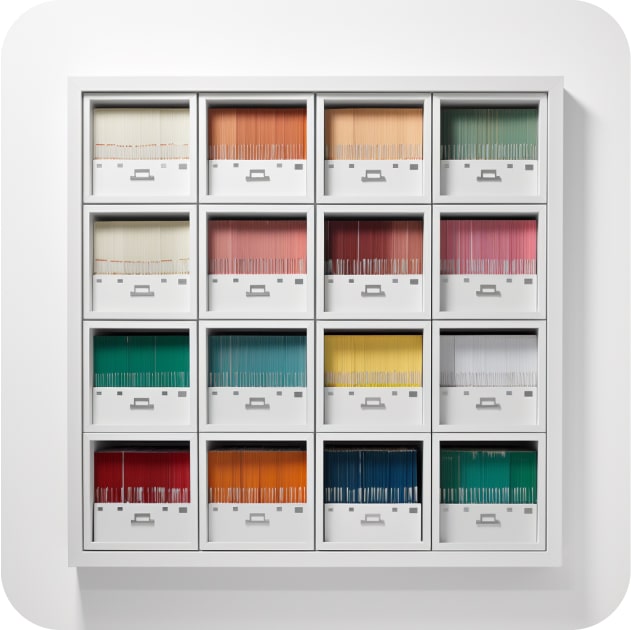
Augment
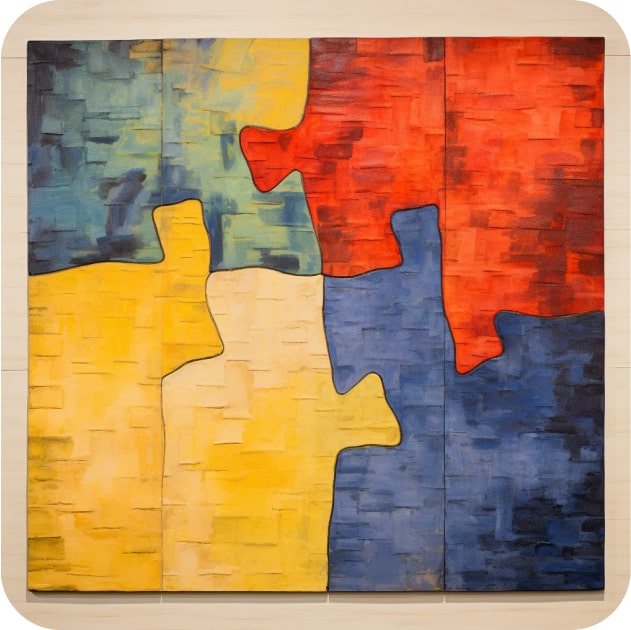
Auto-materialize
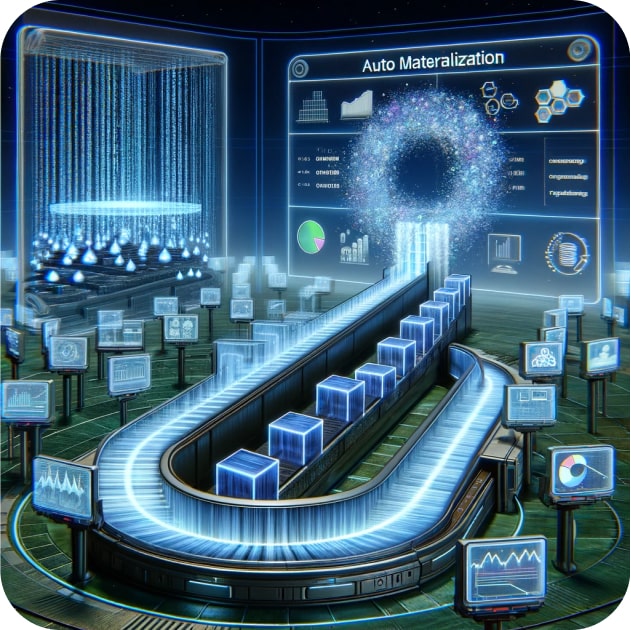
Backup
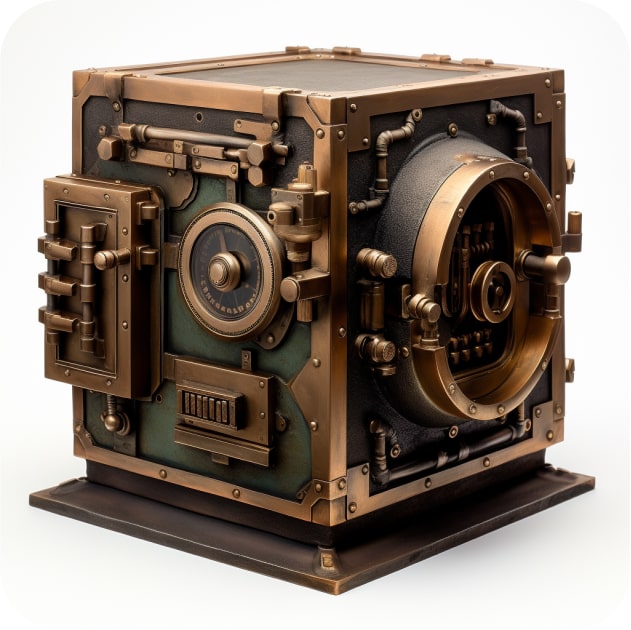
Batch Processing
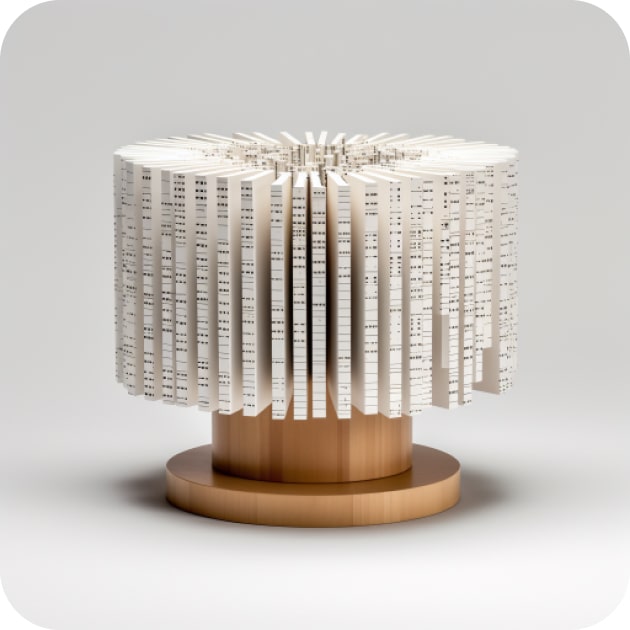
Cache
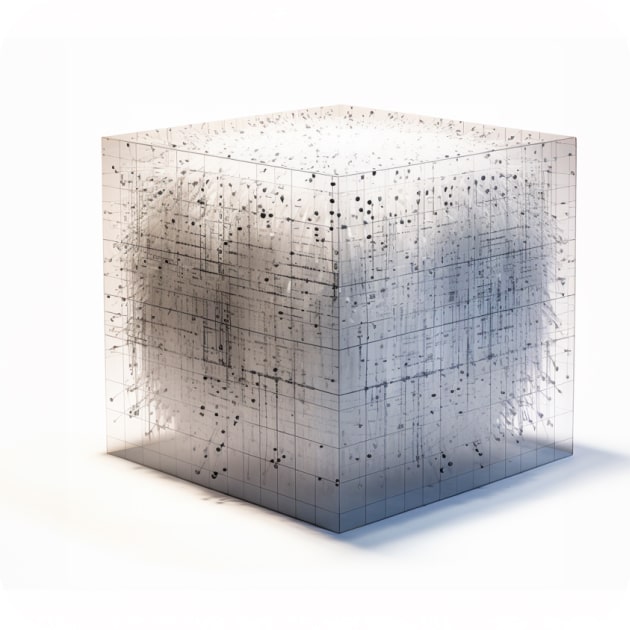
Categorize
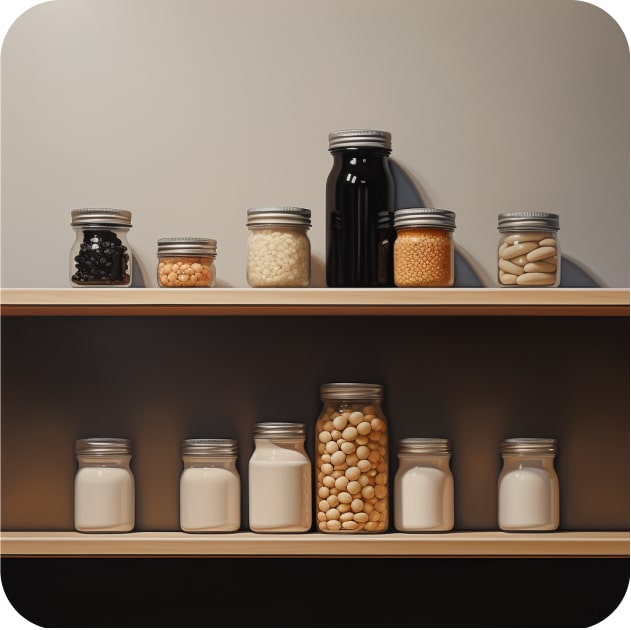
Checkpointing
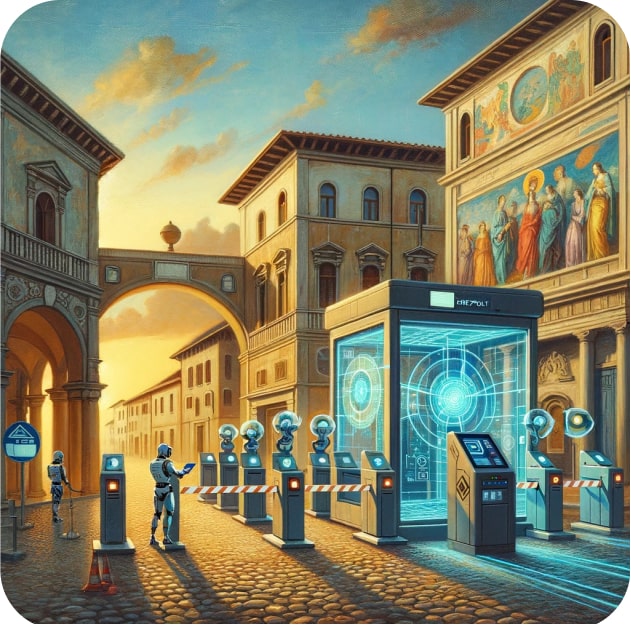
Deduplicate
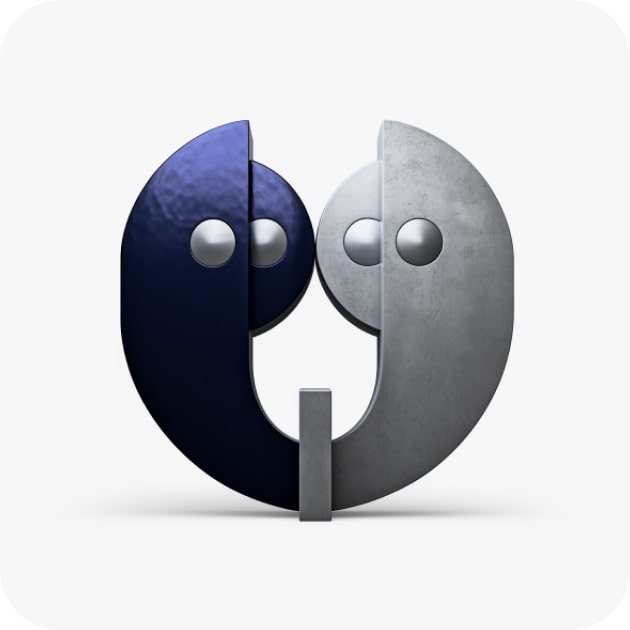
Deserialize
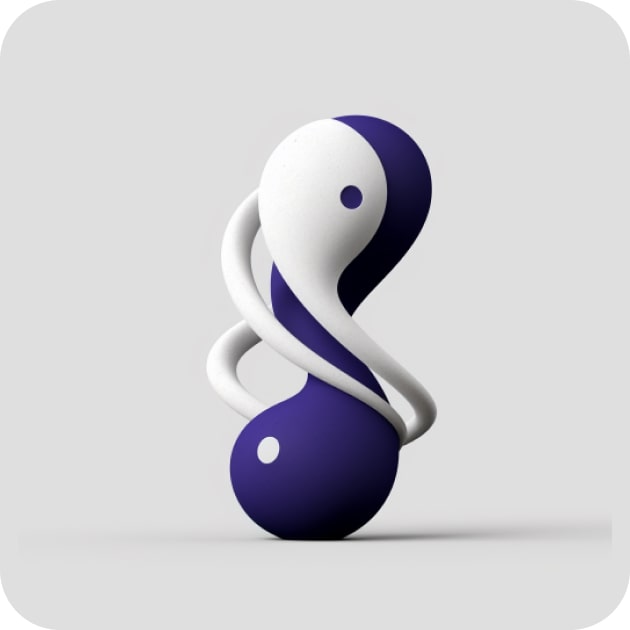
Encapsulate
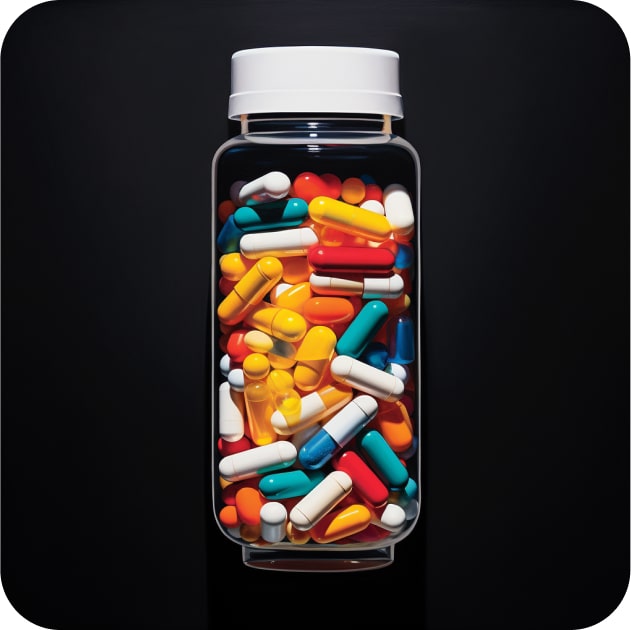
Enrich
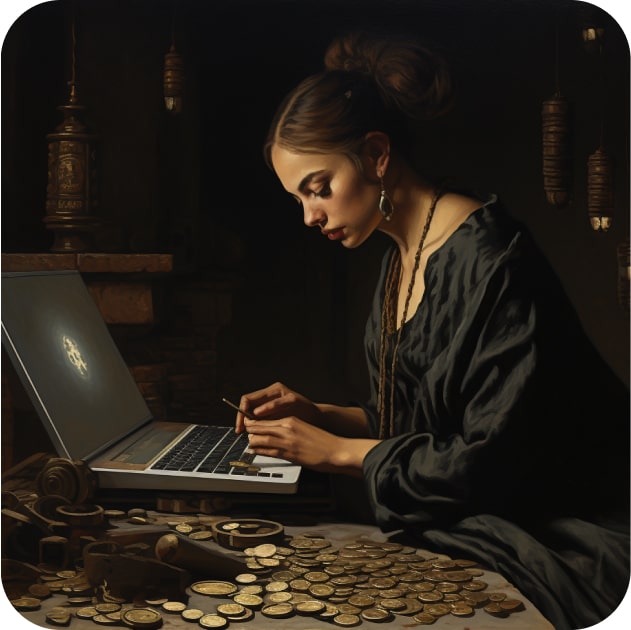
Export
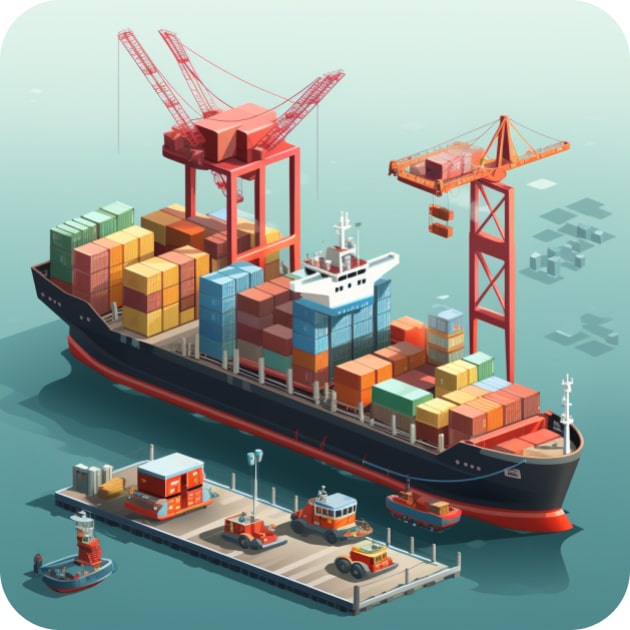
Graph Theory
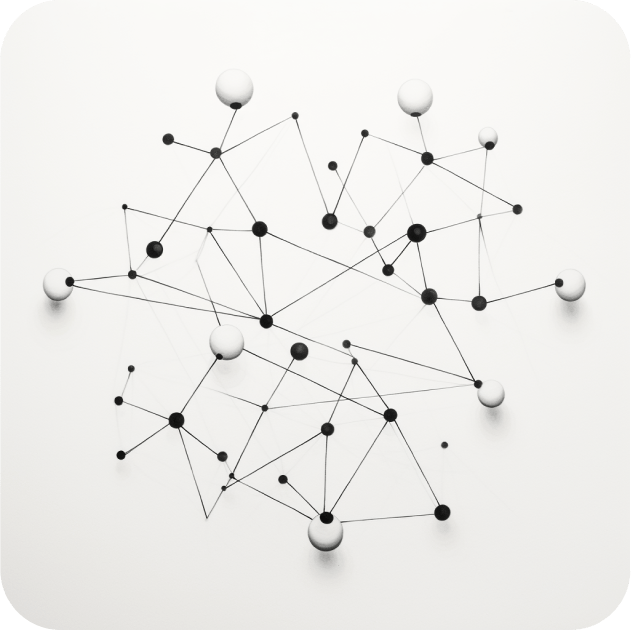
Idempotent
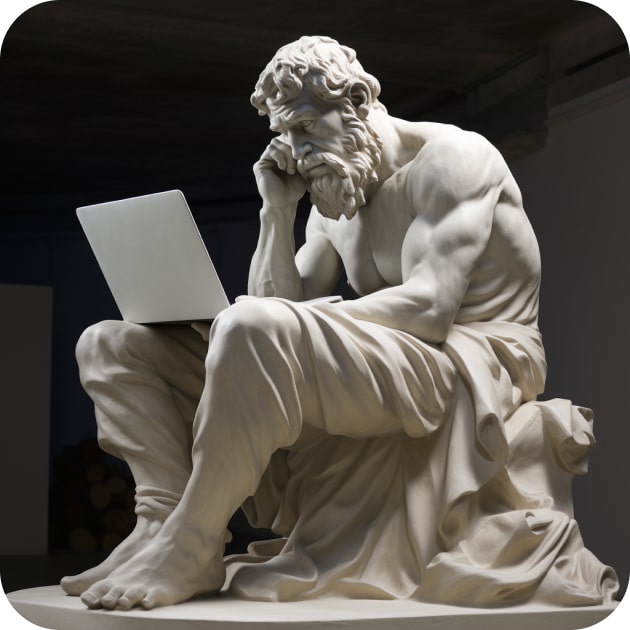
Index
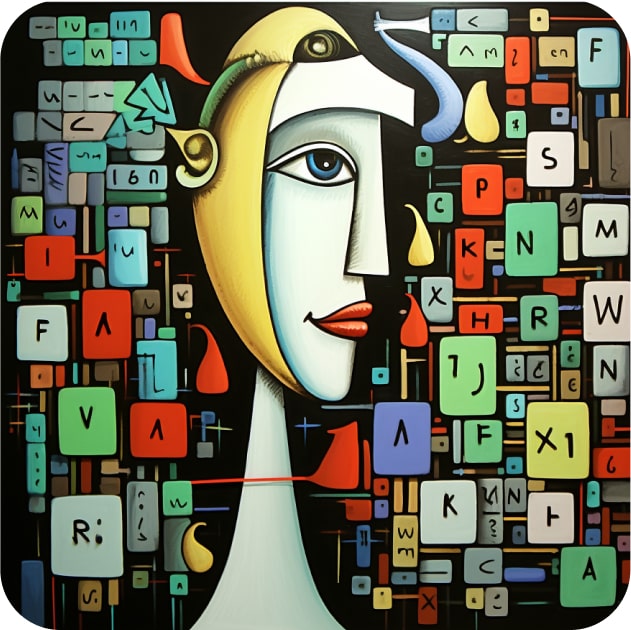
Integrate
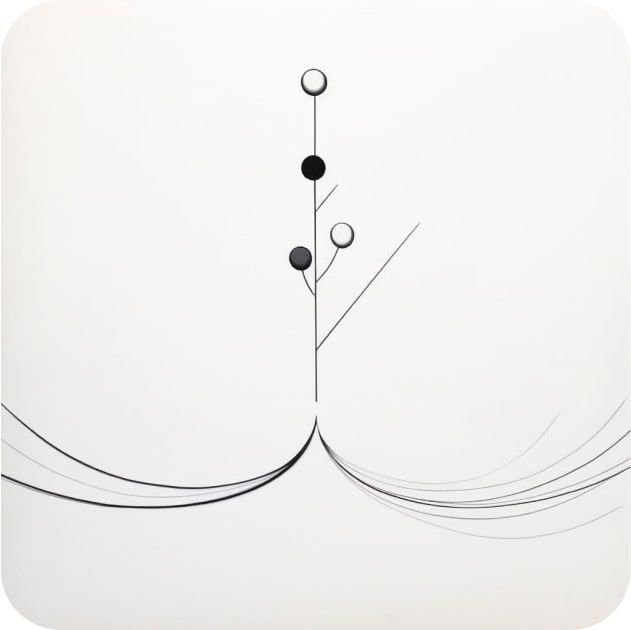
Lineage
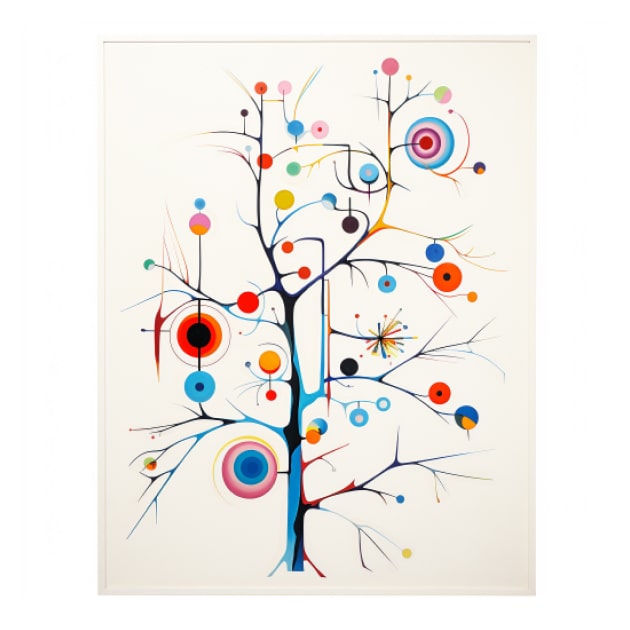
Linearizability
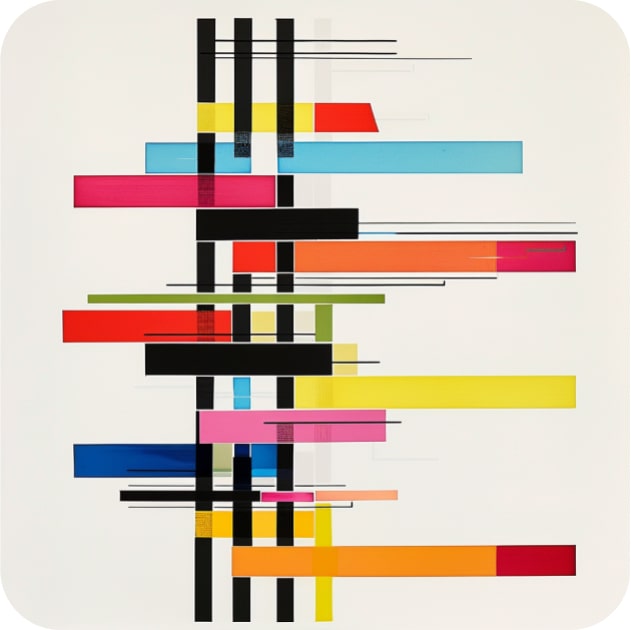
Materialize
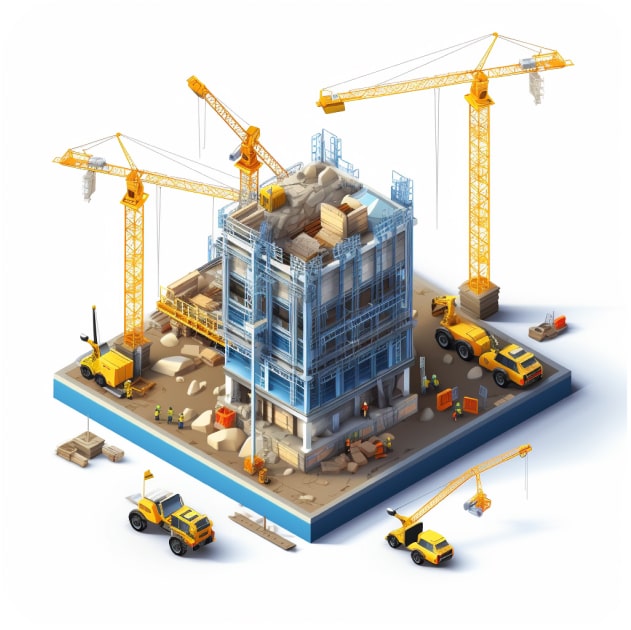
Memoize
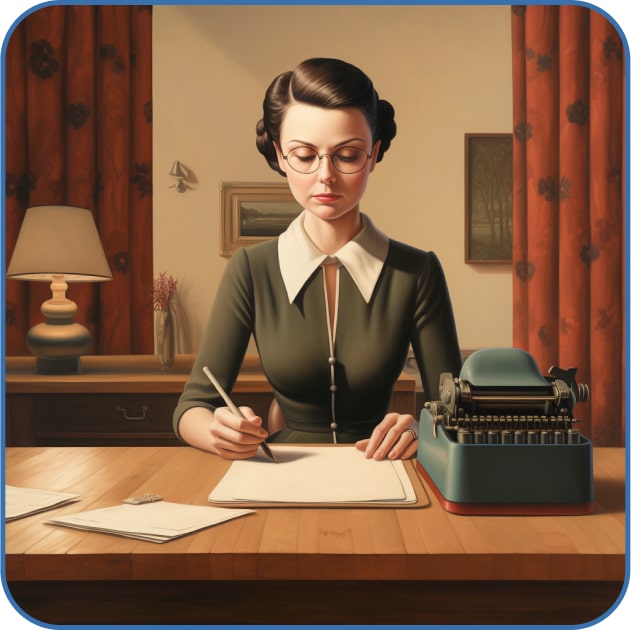
Merge
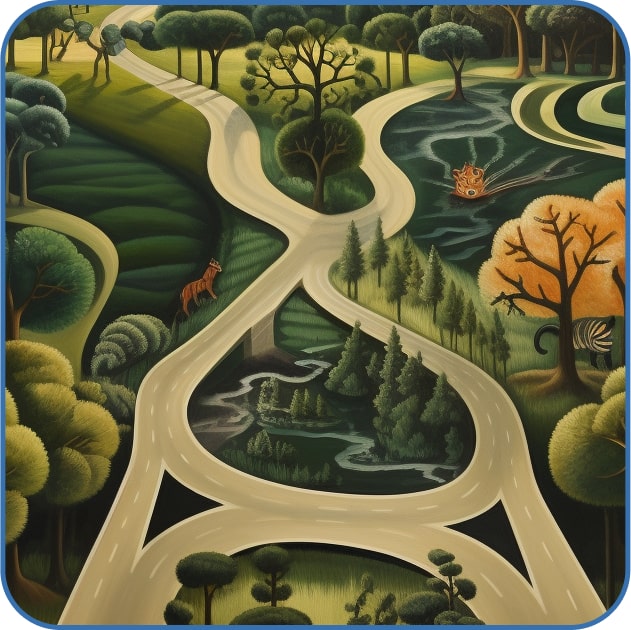
Model
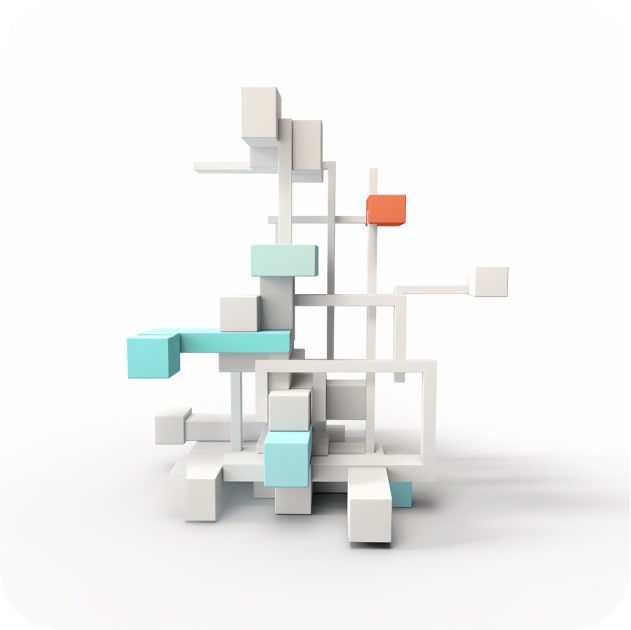
Monitor
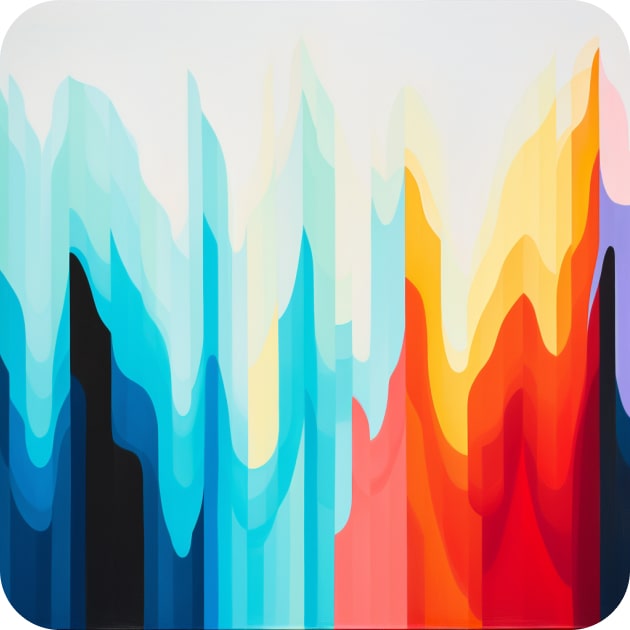
Named Entity Recognition
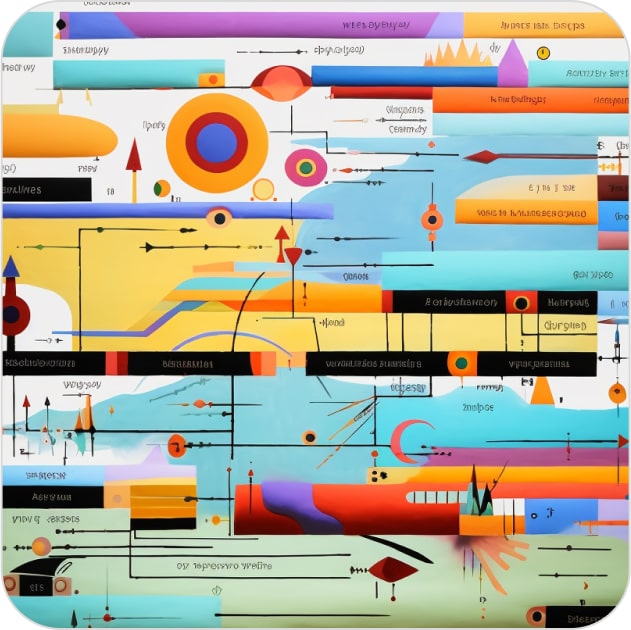
Parse
Partition
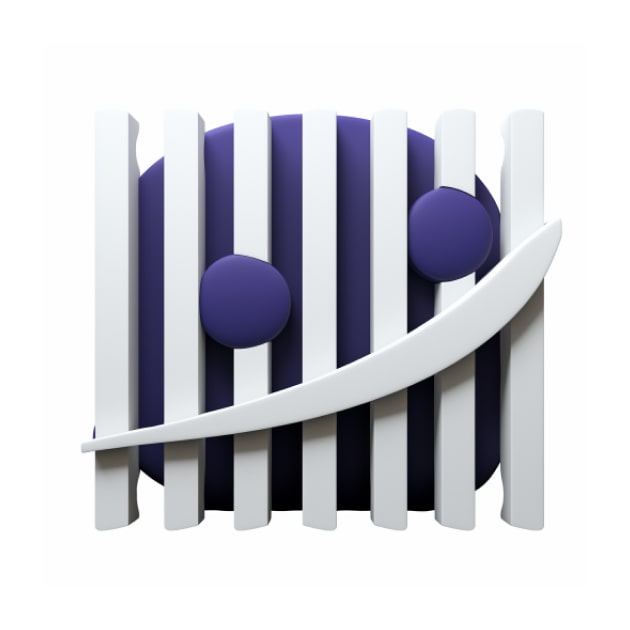
Prep
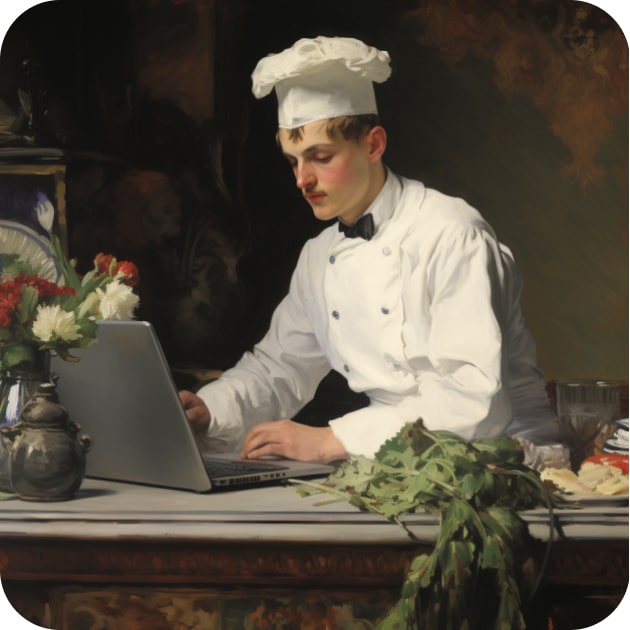
Preprocess
Primary Key
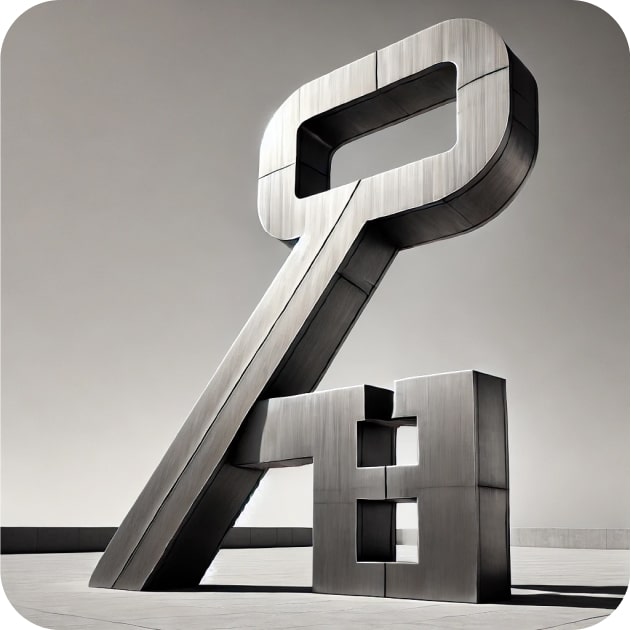
Replicate
Scaling
Schema Inference
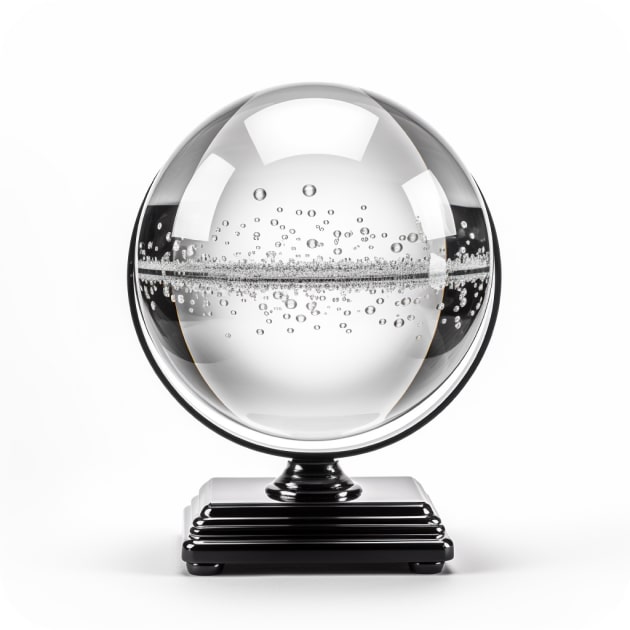
Schema Mapping
Secondary Index
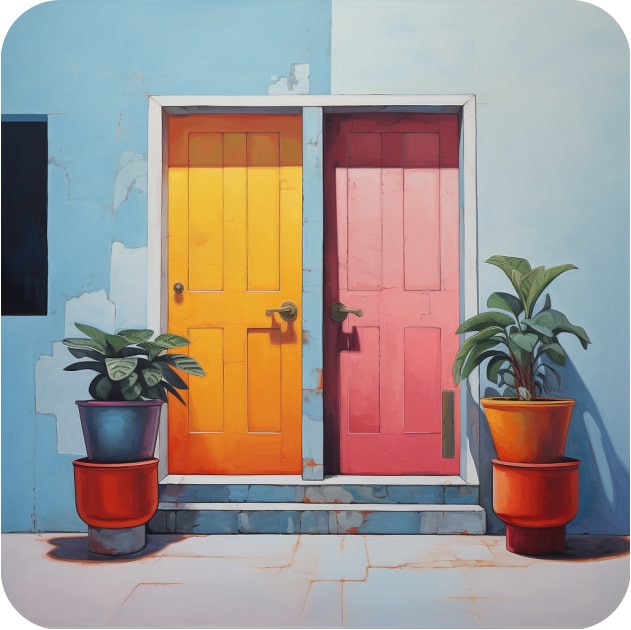
Software-defined Asset
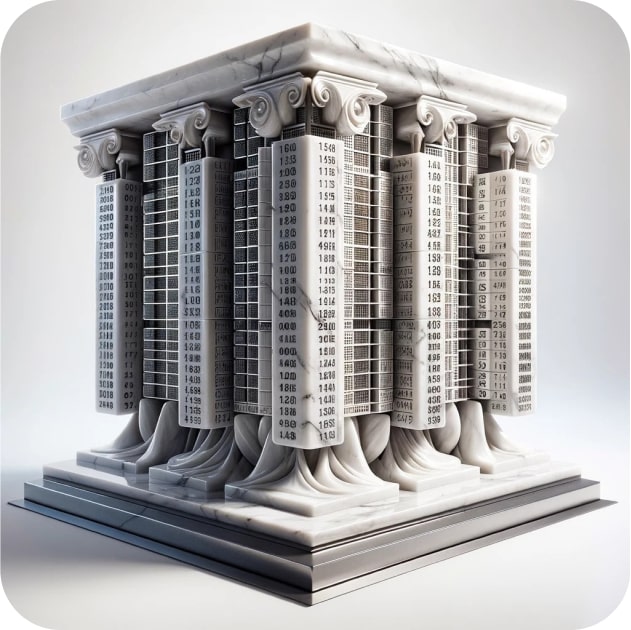
Synchronize
Validate
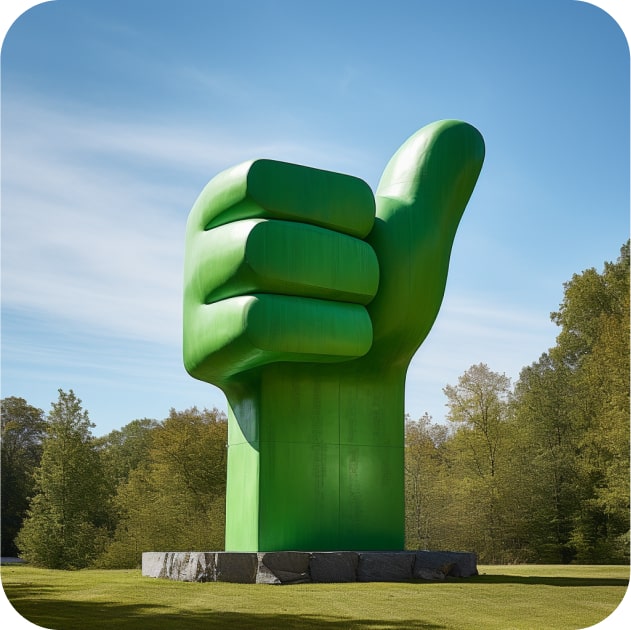
Version
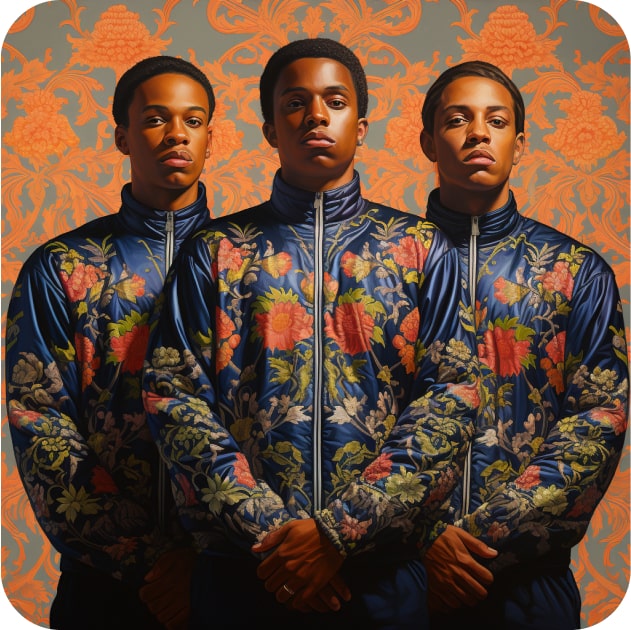