Dagster Data Engineering Glossary:
Checkpointing
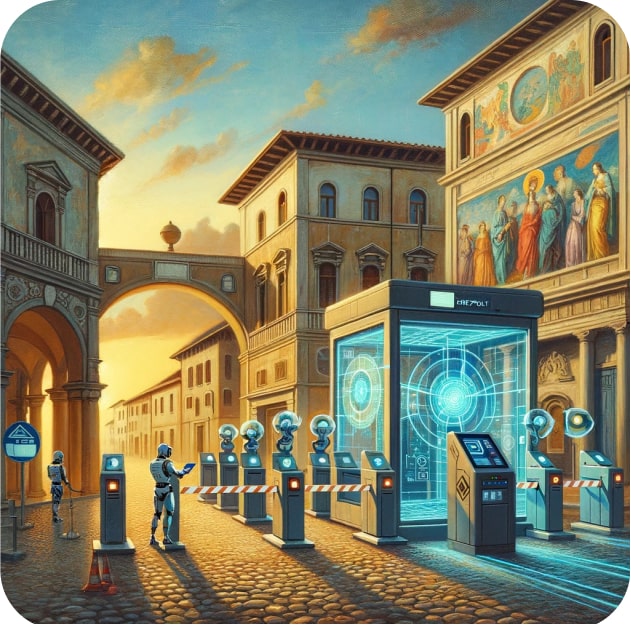
Definition of checkpointing:
Checkpointing, in the context of data processing and pipeline execution, refers to the practice of saving the state of a process at certain points so that it can be restarted from that point in case of failure, rather than from the beginning. This can be crucial for long-running processes and pipelines, ensuring that progress is not lost and that computation resources are not wasted.
Key Aspects of Checkpointing
Fault Tolerance: In distributed systems, failures are inevitable. Checkpointing allows a system to recover from failures by restarting the processing from the last saved state rather than reprocessing all the data from scratch. This minimizes data loss and processing time in case of hardware failures, software bugs, or network issues.
State Management: The "state" in data processing refers to the intermediate data and computations that a task depends on. Checkpointing involves saving this state periodically so that it can be reloaded and used to resume processing.
Performance Optimization: By reducing the amount of reprocessing needed after a failure, checkpointing helps optimize resource usage and overall performance of data pipelines. This is particularly important in large-scale data processing where reprocessing from the start can be very time-consuming and resource-intensive.
Consistency: Checkpointing ensures data consistency by maintaining a coherent state of data processing. If a failure occurs, the system can roll back to the last consistent checkpoint and continue processing, avoiding partial and inconsistent states.
Checkpointing Mechanisms
Checkpointing can be implemented in various ways, depending on the system and the specific requirements:
Event-Based Checkpointing: Checkpoints are taken based on certain events, such as the completion of a specific data batch or the processing of a certain number of records. This is typically more efficient than time-based checkpointing and more reliable than manual approaches.
Time-Based or Scheduled Checkpointing: The system takes checkpoints at regular time intervals. This is simple to implement but might not always be optimal in terms of resource usage. This is typically an older approach used in slow-moving legacy systems.
Manual Checkpointing: Here, developers have control over when checkpoints are created, allowing for more fine-grained control over the process. This is most suited for one-off system changes and upgrades.
Implementation Considerations
When implementing checkpointing, several factors need to be considered:
Storage: The choice of storage for checkpoints is crucial. It needs to be reliable and durable, often involving distributed storage systems like AWS S3, or similar. Frequency: Deciding the frequency of checkpoints involves a trade-off between fault tolerance and performance. Frequent checkpoints provide better fault tolerance but may incur higher overhead. State Size: The size of the state being saved can impact the efficiency of checkpointing. Efficient serialization and compression techniques may be needed to manage large state sizes.
Checkpointing in Dagster
Dagster implicitly supports a form of checkpointing through asset materializations. This allows for more efficient pipeline execution, as computations can be resumed based on the latest successful asset materializations rather than starting from scratch.
Dagster assets represent units of data that are the outputs of computations. When you define assets in Dagster, you create a graph of these assets, specifying how they depend on one another. Each time an asset is computed or updated ("materialized"), Dagster records this event, effectively creating a checkpoint in the asset's lifecycle. This metadata can serve a similar purpose to checkpoints, as it allows users to understand the state of their data assets at any given time and to restart computations from the last known good state if necessary.
Checkpointing is a technique used in data engineering to save the state of a process at specific intervals. This allows for recovery from failures without having to restart the entire process. Here's an example of checkpointing in a data processing pipeline using Python. We'll simulate a data processing task that reads data in chunks, processes it, and uses checkpointing to save the progress.
A Python example of Checkpointing
Let's demonstrate checkpointing in Python using a CSV file to simulate data chunks and a checkpoint file to store the progress. First, let's create a sample CSV file with dummy data:
import csv
# Sample data
data = [
['id', 'name', 'value'],
[1, 'Alice', 10],
[2, 'Bob', 20],
[3, 'Charlie', 30],
[4, 'David', 40],
[5, 'Eve', 50],
[6, 'Jacob', 60],
[7, 'Porshe', 70],
[8, 'Le', 80],
[9, 'Antoine', 90],
[10, 'Katarina', 100]
]
# Write sample data to CSV
with open('data.csv', 'w', newline='') as file:
writer = csv.writer(file)
writer.writerows(data)
Checkpointing Example
Now, let's write a Python script to process this data in chunks and use checkpointing to save the progress. We will build in a delay to give us the chance to interact with the program.
import csv
import os
import time
# Function to read the last checkpoint
def read_checkpoint():
if os.path.exists('checkpoint.txt'):
with open('checkpoint.txt', 'r') as file:
return int(file.read().strip())
return 0
# Function to write the current checkpoint
def write_checkpoint(checkpoint):
with open('checkpoint.txt', 'w') as file:
file.write(str(checkpoint))
# Function to process data
def process_data(row):
# Simulate data processing
print(f"Processing row: {row}")
# Main function to read and process data with checkpointing
def main():
checkpoint = read_checkpoint()
print(f"Starting from checkpoint: {checkpoint}")
with open('data.csv', 'r') as file:
reader = csv.reader(file)
header = next(reader) # Skip header
current_position = 0
for row in reader:
if current_position >= checkpoint:
process_data(row)
checkpoint = current_position + 1
write_checkpoint(checkpoint)
# Delay for 5 seconds to allow interruption
time.sleep(5)
current_position += 1
if __name__ == "__main__":
main()
To break down the steps:
Checkpoint Read/Write Functions: read_checkpoint()
: Reads the last checkpoint from the file, and then write_checkpoint(checkpoint)
writes the current checkpoint to the file.
Data Processing: process_data(row)
: Simulates processing a data row.
Main Function: Here we read the last checkpoint, opens the CSV file and skips the header, process data rows starting from the last checkpoint, then update the checkpoint after processing each row.
Let's run the Script
If you now execute the two scripts above in sequence, the second one will start from the beginning and process all rows form the test file. You can stop the script mid-execution (e.g., using a keyboard interrupt). When you run the script again, it will resume processing from the last saved checkpoint.
This example demonstrates a simple yet effective way to implement checkpointing in a data processing pipeline, allowing for resumption of work after interruptions without reprocessing already completed data.
Your output may look something like this:
% python checkpoint.py
Starting from checkpoint: 0
Processing row: ['1', 'Alice', '10']
Processing row: ['2', 'Bob', '20']
^C KeyboardInterrupt
% python checkpoint.py
Starting from checkpoint: 2
Processing row: ['3', 'Charlie', '30']
Processing row: ['4', 'David', '40']
Processing row: ['5', 'Eve', '50']
^C KeyboardInterrupt
% python checkpoint.py
Starting from checkpoint: 5
Processing row: ['6', 'Jacob', '60']
^C KeyboardInterrupt
% python checkpoint.py
Starting from checkpoint: 6
Processing row: ['7', 'Porshe', '70']
Processing row: ['8', 'Le', '80']
Processing row: ['9', 'Antoine', '90']
Processing row: ['10', 'Katarina', '100']
Append
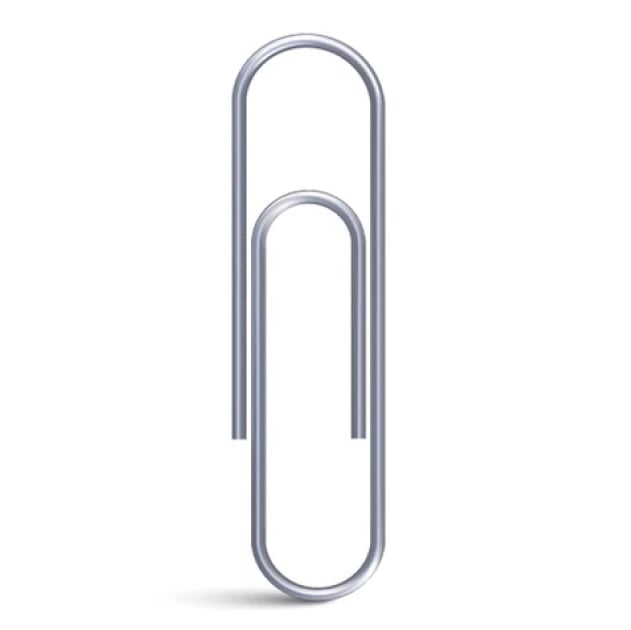
Archive
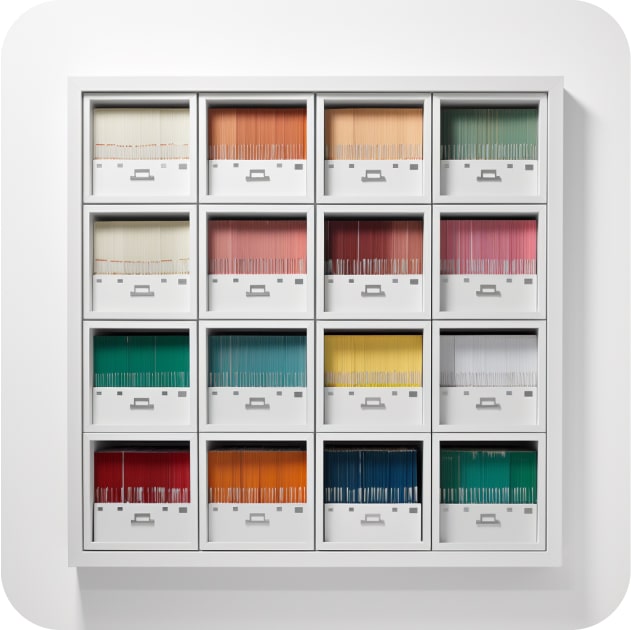
Augment
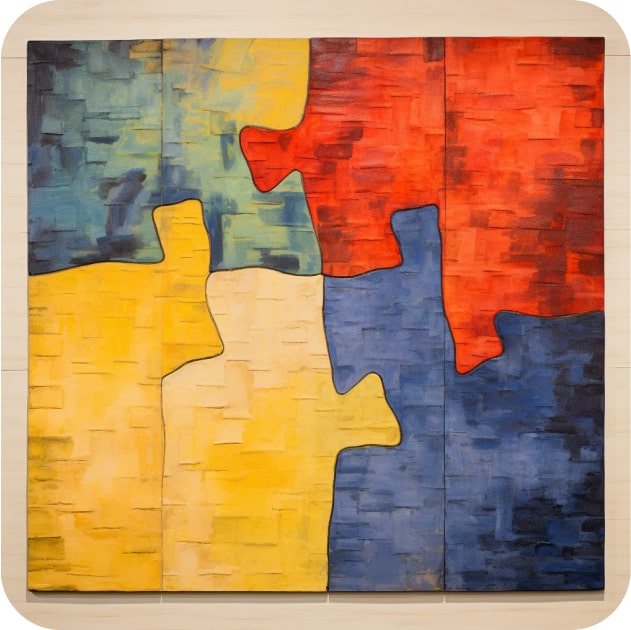
Auto-materialize
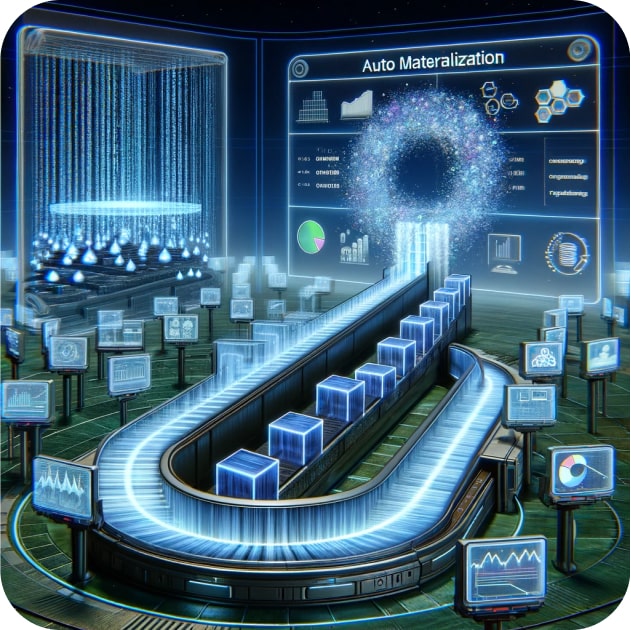
Backup
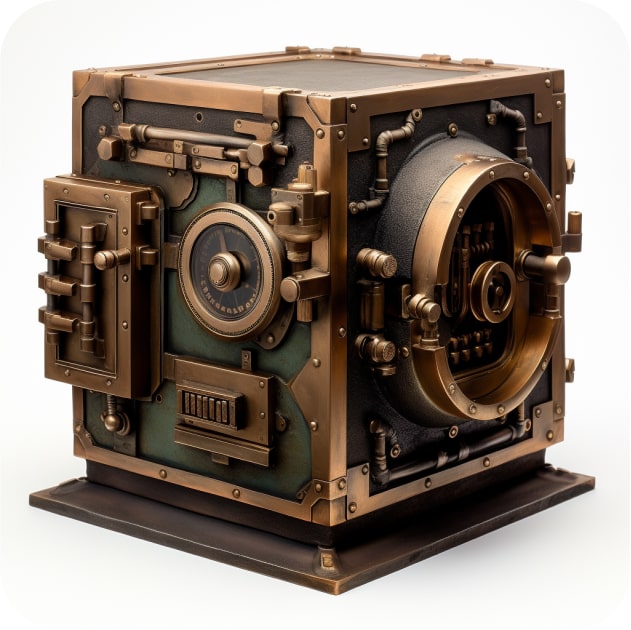
Batch Processing
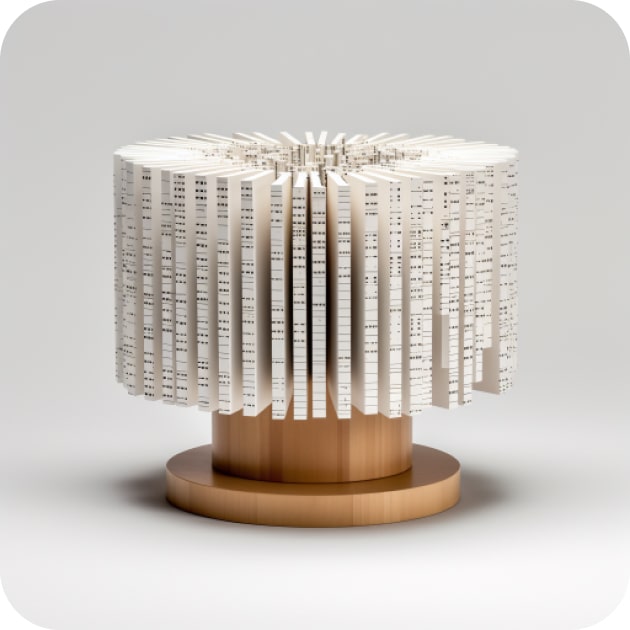
Cache
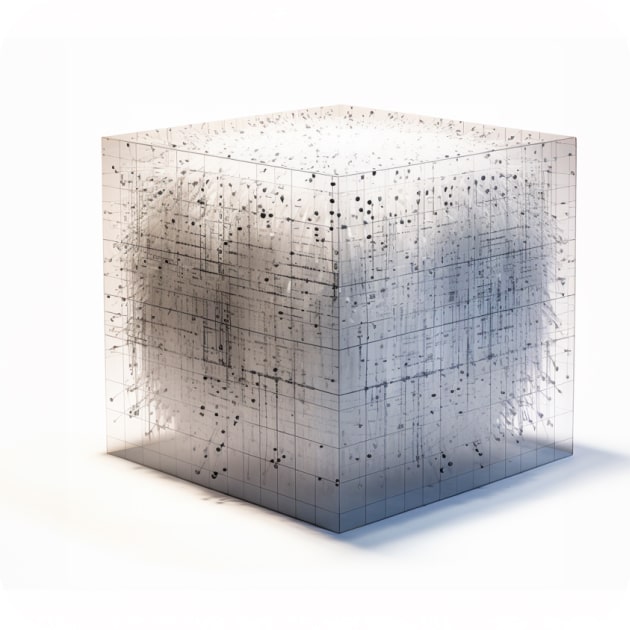
Categorize
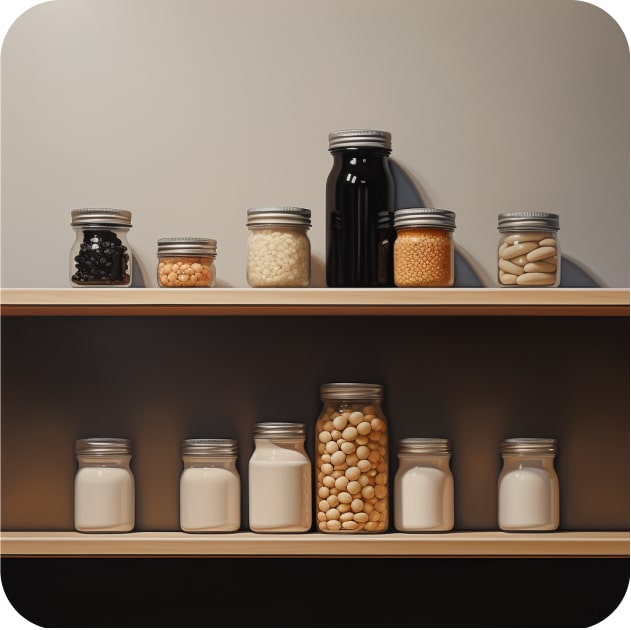
Deduplicate
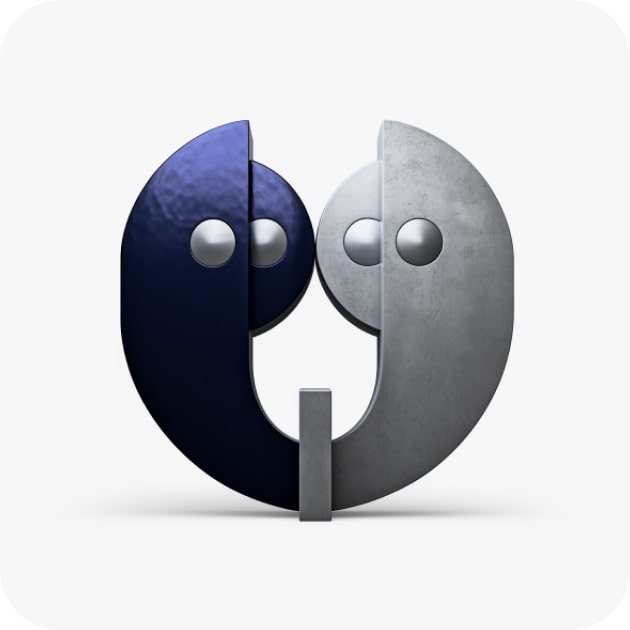
Deserialize
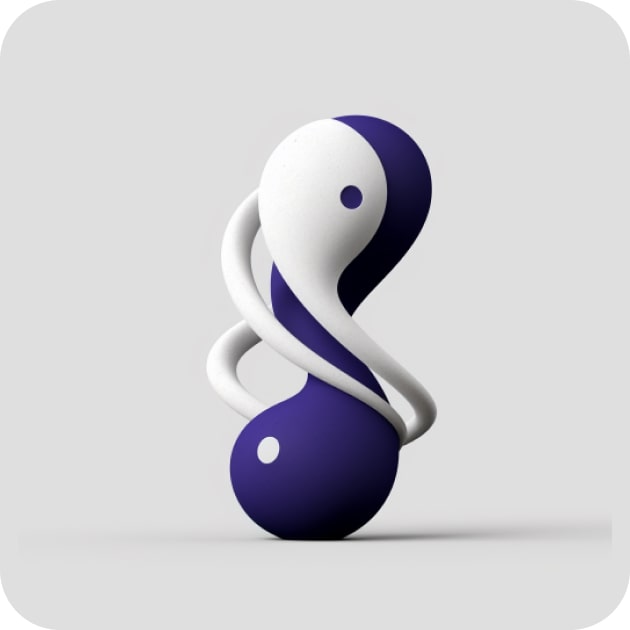
Dimensionality
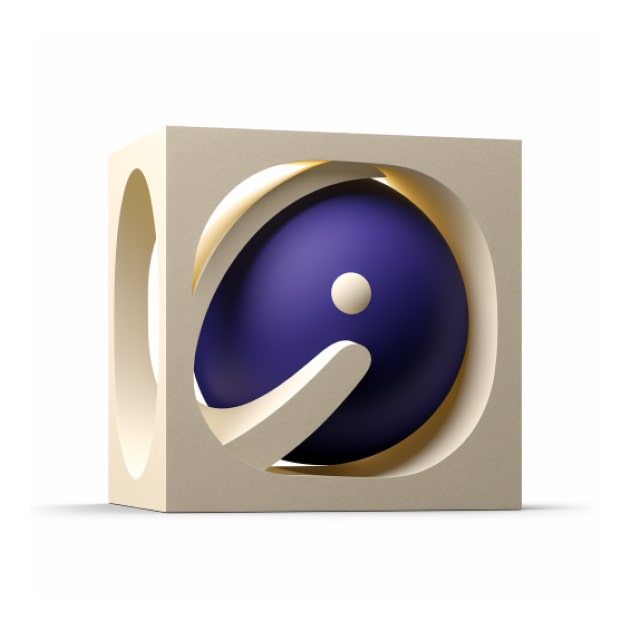
Encapsulate
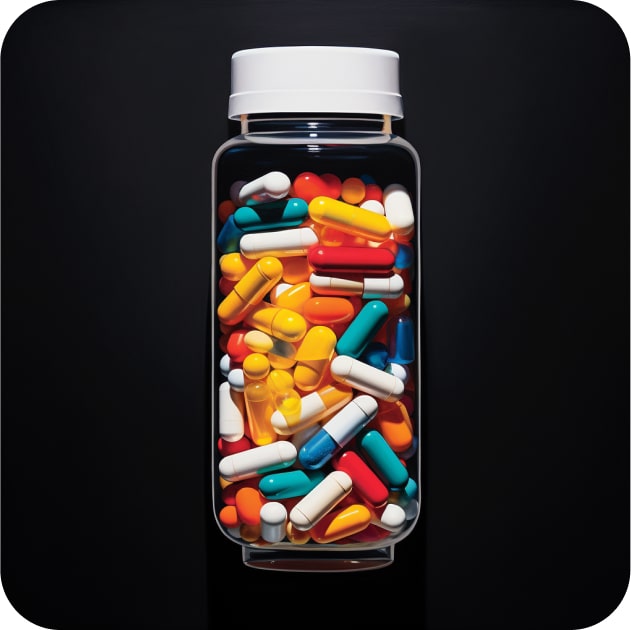
Enrich
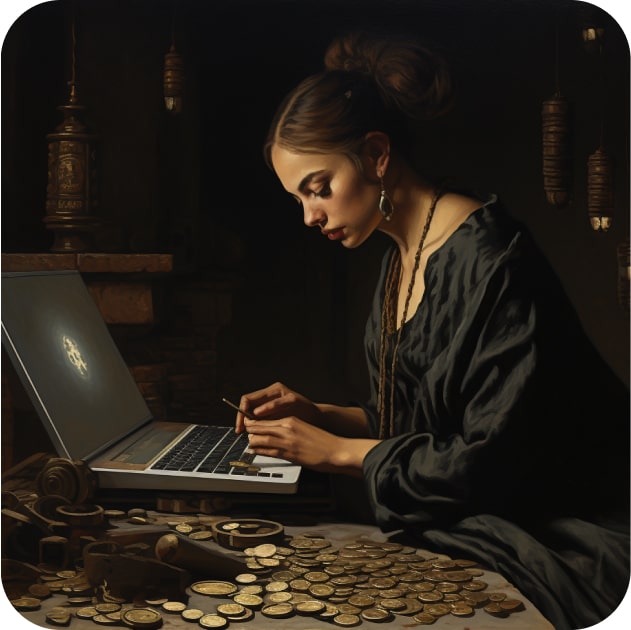
Export
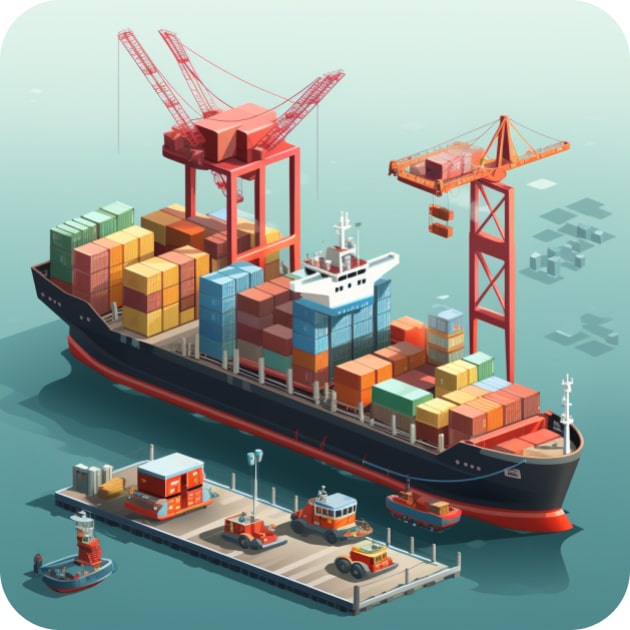
Graph Theory
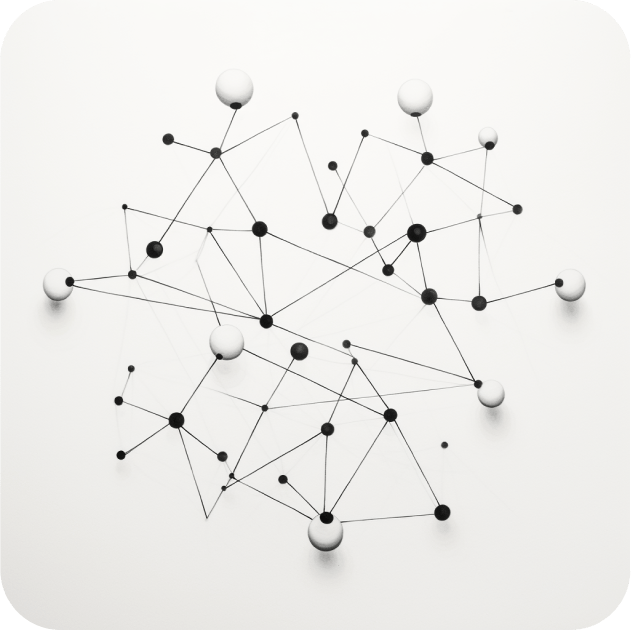
Idempotent
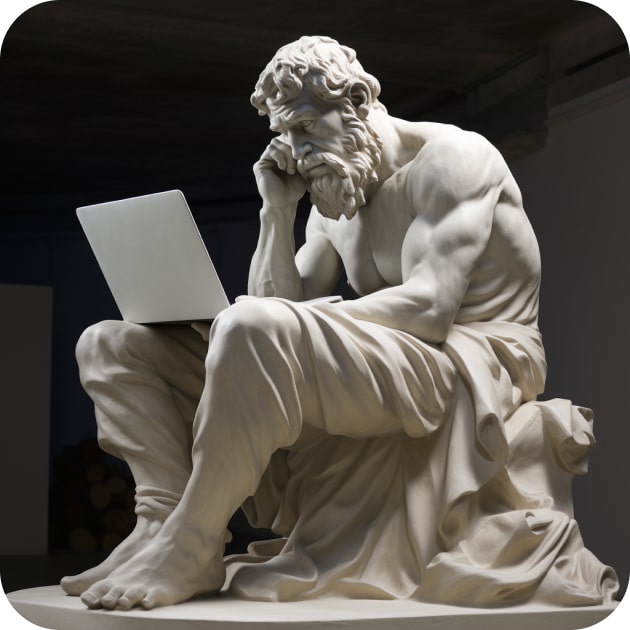
Index
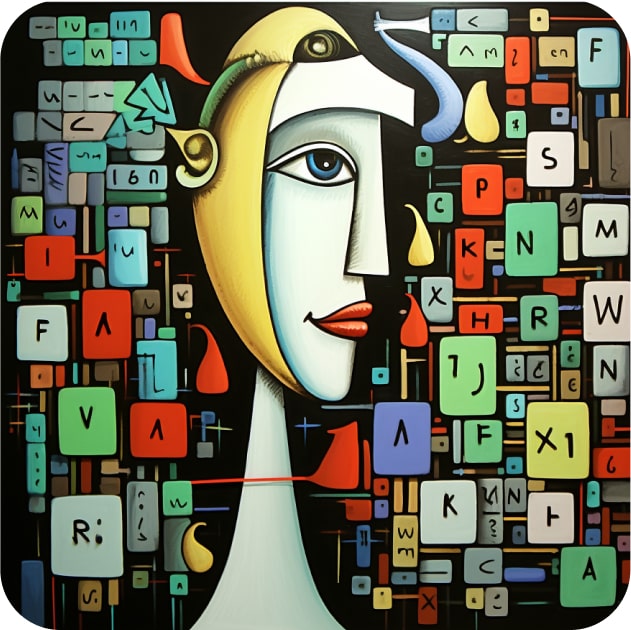
Integrate
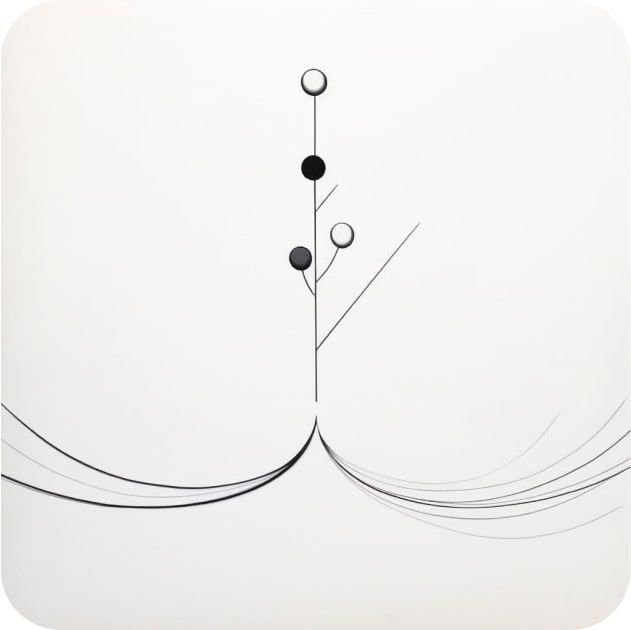
Lineage
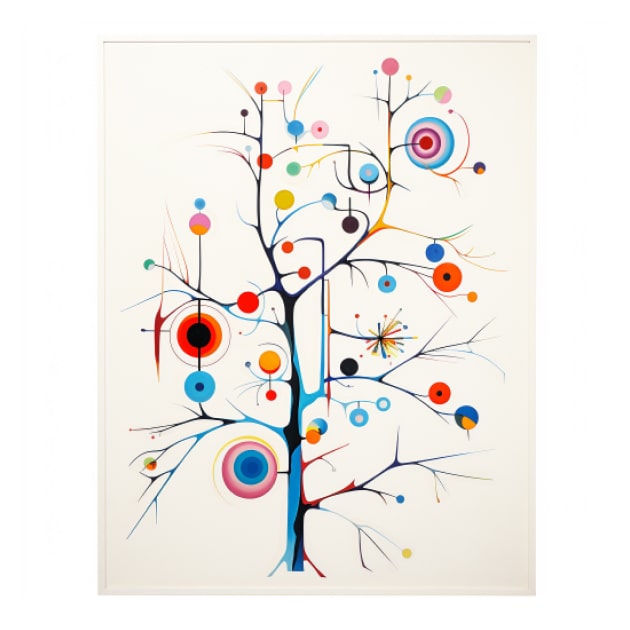
Linearizability
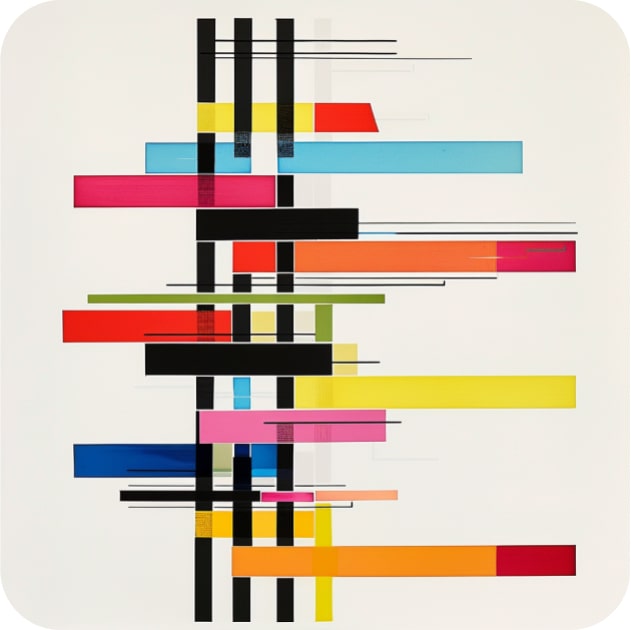
Materialize
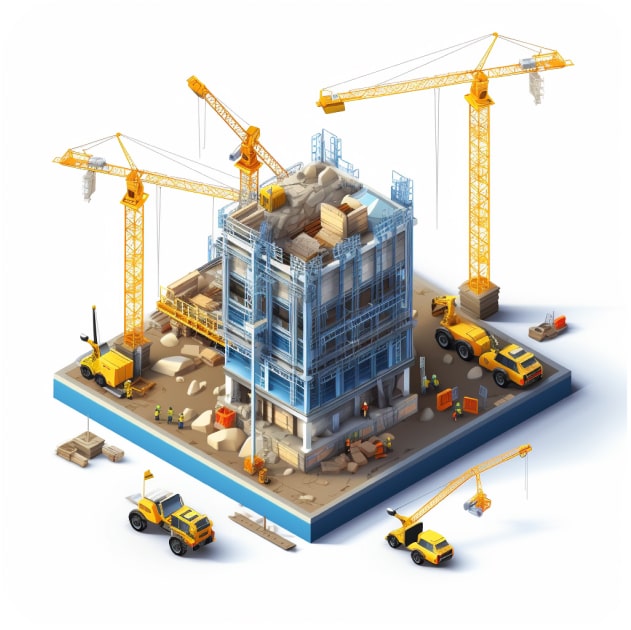
Memoize
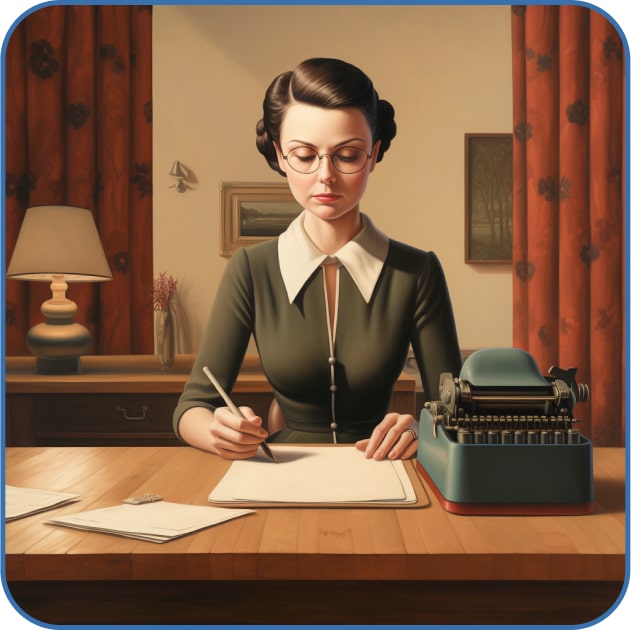
Merge
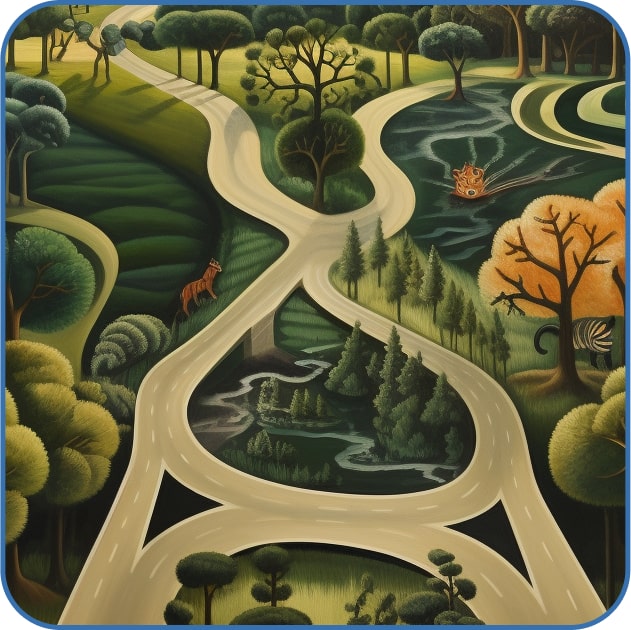
Model
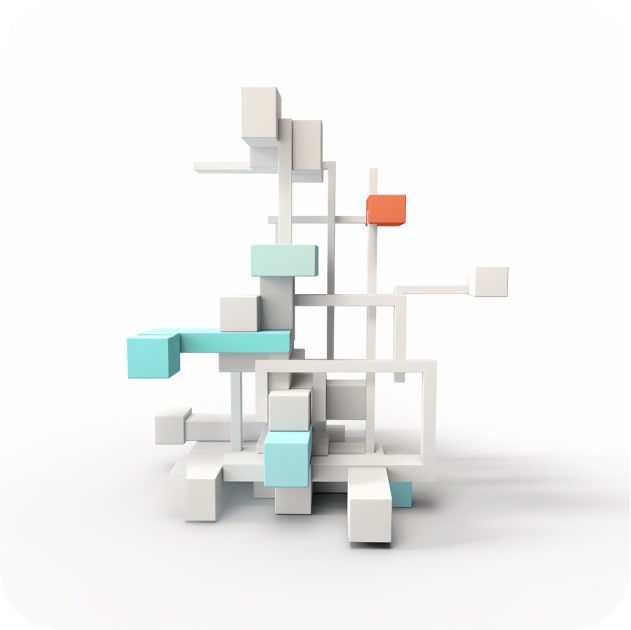
Monitor
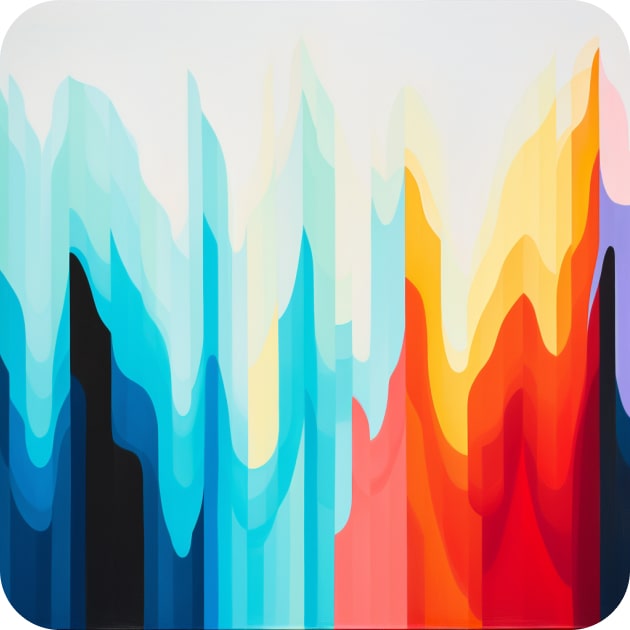
Named Entity Recognition
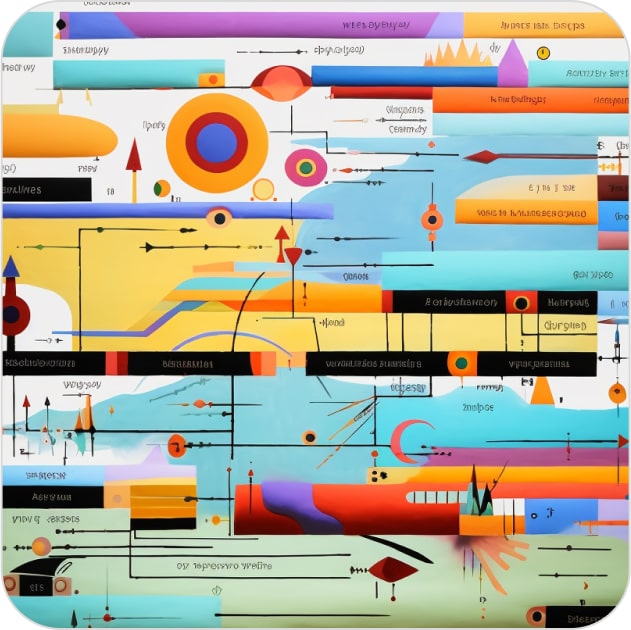
Parse
Partition
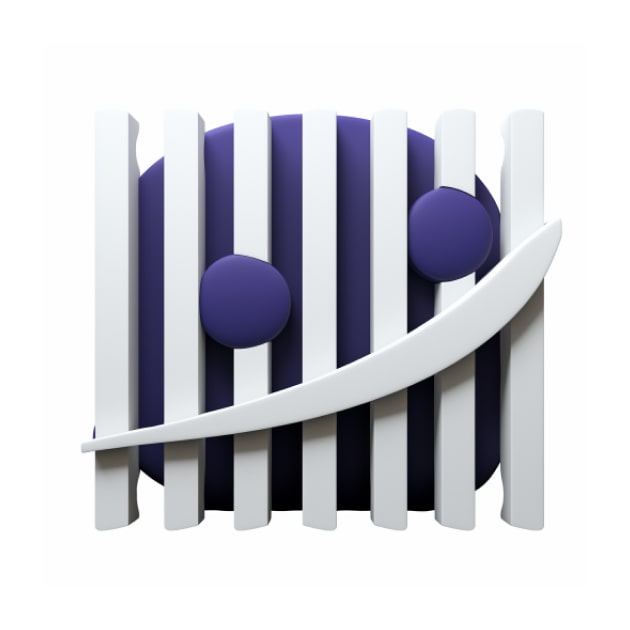
Prep
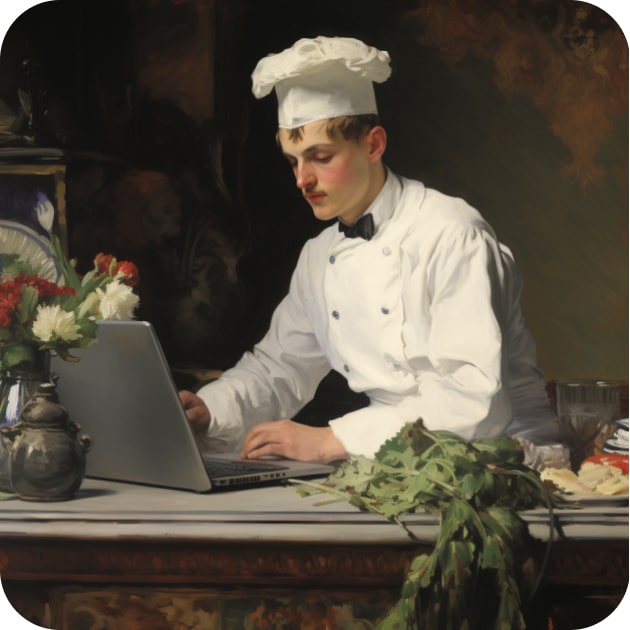
Preprocess
Primary Key
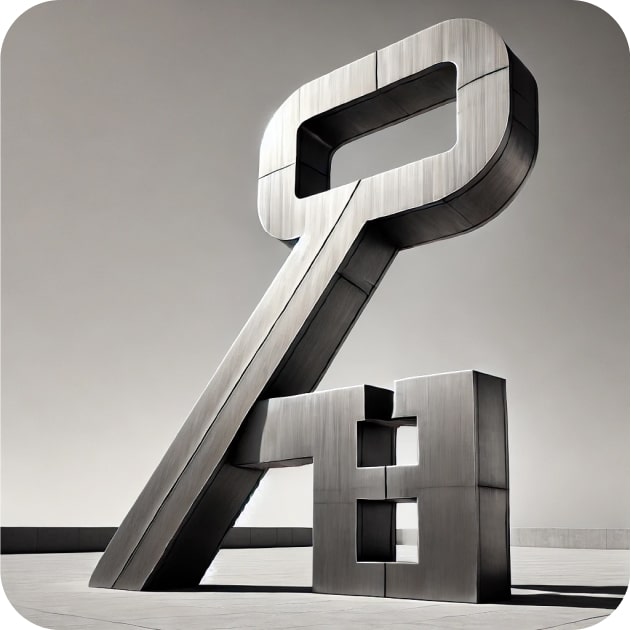
Replicate
Scaling
Schema Inference
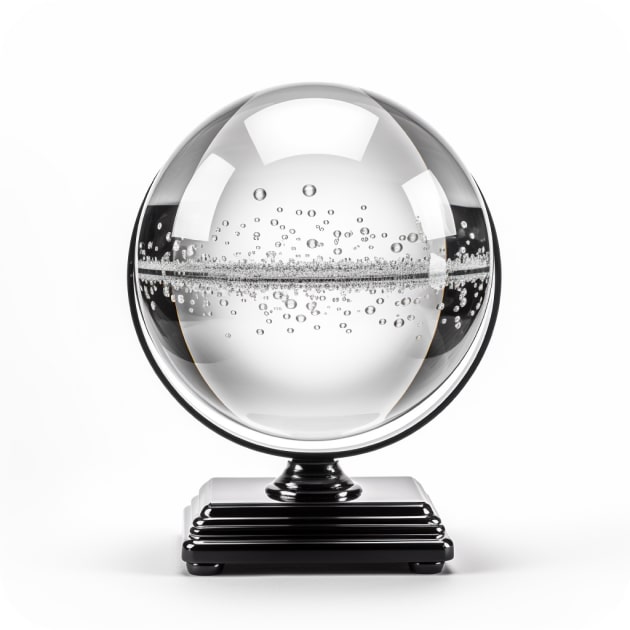
Schema Mapping
Secondary Index
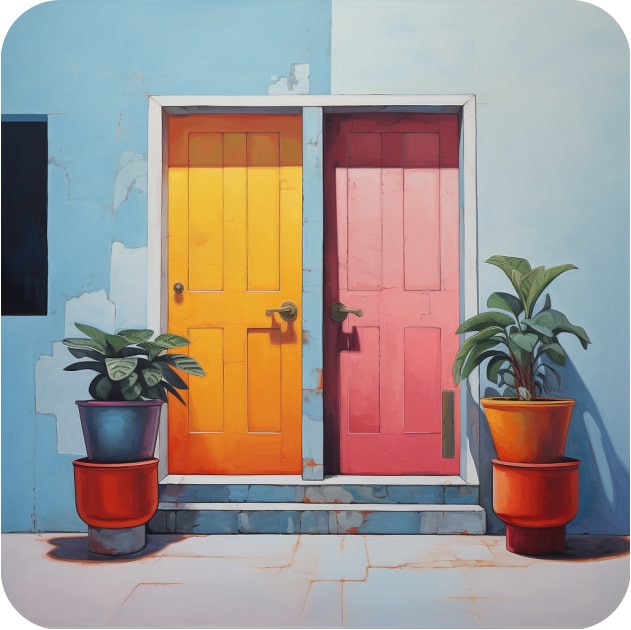
Software-defined Asset
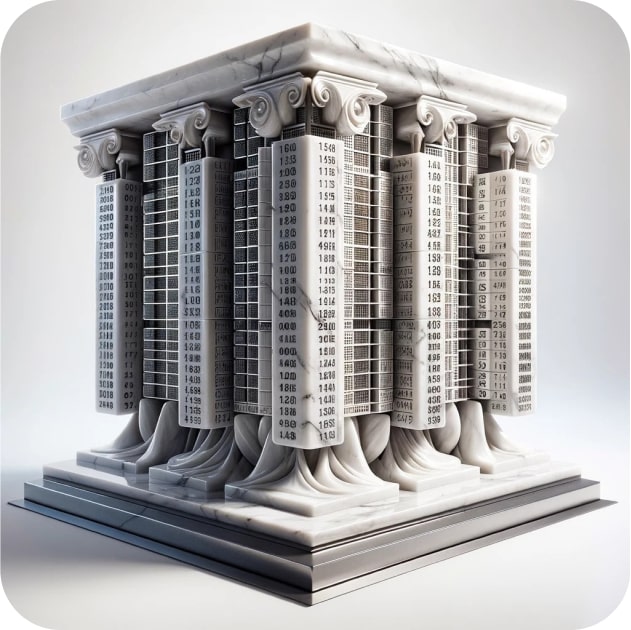
Synchronize
Validate
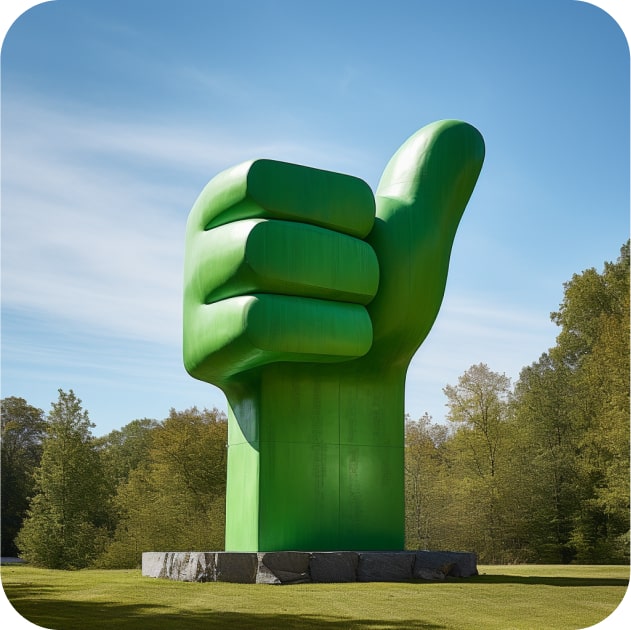
Version
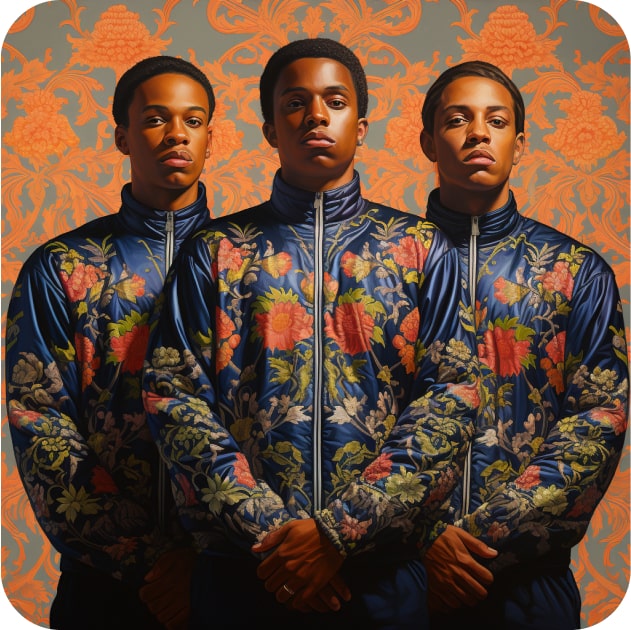