Dagster Data Engineering Glossary:
Linearizability
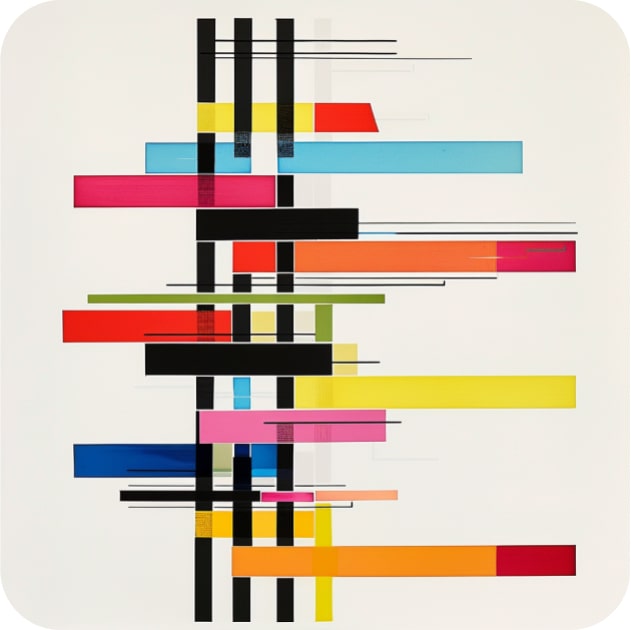
Linearizability definition:
Linearizability is a correctness condition that ensures that all operations on a distributed system (such as reads, writes, and updates to a data store) appear to occur instantaneously at some point between their start and end times. It constrains what outputs are possible when an object is accessed by multiple processes concurrently. This means that if one operation finishes before another operation starts, the first operation must appear to happen before the second in the system's global order of operations.
As a concept, Linearizability is used in the field of distributed systems and data engineering as it pertains to the consistency of operations in a distributed data store. It is a specific form of consistency model that aims to simplify the understanding and reasoning about the behavior of distributed systems.
Linearizability is not related to the concept of linearizing data.
Key Properties of Linearizability
- Atomicity: Each operation is atomic, meaning it either happens entirely or not at all. There's no partial visibility of operations to the clients.
- Ordering: Operations are ordered in a way that respects real-time. If operation A completes before operation B starts, then operation A must appear to have happened before operation B in the system's history.
- Consistency: The system's state after any sequence of operations must be the same as if the operations had been executed in some sequential order that is consistent with the real-time ordering of the operations.
Importance of Linearizability in Distributed Systems
Linearizability is crucial for distributed systems because it provides a straightforward and intuitive way of understanding the system's behavior. When a system is linearizable, developers and users can reason about its state and operations as if they were dealing with a single, atomic system, even though the operations may be distributed across multiple nodes and may involve complex coordination.
Examples and Applications of Linearizability
- Distributed databases: Ensuring that all replicas of a piece of data reflect the same sequence of updates, even in the presence of network partitions and failures.
- Concurrent data structures: Like concurrent queues or maps in multi-threaded programming, where operations by different threads must reflect a single, coherent order of execution.
Challenges and Trade-offs
Implementing linearizability in distributed systems comes with its challenges, primarily related to performance and availability. Ensuring strict ordering and atomicity of operations can lead to increased latency, as operations might need to wait for coordination among nodes. Additionally, in the presence of network partitions or node failures, maintaining linearizability can lead to decreased availability since the system might need to block operations until it can guarantee their order and atomicity.
In practice, systems often have to balance between strict linearizability and other considerations like availability and partition tolerance (as per the CAP theorem), leading to various consistency models such as eventual consistency, causal consistency, and others that relax some constraints to achieve better performance or availability under certain conditions.
Linearizability and Data Orchestration
Data orchestration handles the complexities of dependencies, scheduling, and the execution of tasks across distributed architectures.
Therefore, the relationship between linearizability and data orchestration in a distributed system is foundational to ensuring that data remains consistent and that workflows execute reliably. Since linearizability provides a guarantee that operations on data are executed in a manner that respects the chronological order of events, it is crucial for data orchestration, which relies on the predictable and consistent state of data to manage dependencies between tasks effectively.
For instance, in a data pipeline that involves reading data from a distributed database, transforming it, and then writing it back to another store, linearizability ensures that the read operations see the most recent writes in the correct order. This consistency is vital for the orchestration layer to correctly sequence tasks, manage dependencies, and ensure that each step of the pipeline operates on the correct version of the data.
An example of linearizability in Python
Linearizability is a difficult concept to capture outside of a distributed system. This said, to illustrate the concept using just a simple Python example, let's simulate a basic scenario involving a bank account with threaded operations like deposit and withdrawal.
In a linearizable system, if these operations are performed in a certain order, their effects should appear in that same order, regardless of any delays or the distribution of these operations across different threads or processes.
We'll create a simple BankAccount
class with thread-safe deposit and withdrawal methods, ensuring that if one operation completes before another starts, its effect is visible in the order of operations. We'll use threading to simulate concurrent operations and locks to ensure atomicity and maintain linearizability.
Artificial delays were added to the deposit and withdrawal operations to simulate the real-world latency that can occur in a distributed system. These delays help illustrate the importance of linearizability, as the system still ensures that all operations are processed in a consistent and orderly manner, despite the added complexity of concurrent operations and timing differences.
import threading
import random
import time
class BankAccount:
def __init__(self):
self.balance = 0
self.lock = threading.Lock()
def deposit(self, amount):
with self.lock: # Ensuring atomicity and order
# Simulate a delay
time.sleep(random.uniform(0.01, 0.1))
self.balance += amount
print(f"Deposited €{amount}, New Balance: {'€{:,.2f}'.format(self.balance)}")
def withdraw(self, amount):
with self.lock: # Ensuring atomicity and order
# Simulate a delay
time.sleep(random.uniform(0.01, 0.1))
if self.balance >= amount:
self.balance -= amount
print(f"Withdrew €{amount}, New Balance: {'€{:,.2f}'.format(self.balance)}")
else:
print(f"Insufficient funds for withdrawal of {amount}")
def perform_transactions(account):
for _ in range(10):
action = random.choice(['deposit', 'withdraw'])
amount = round(random.randint(1, 100000)/100,2)
if action == 'deposit':
account.deposit(amount)
else:
account.withdraw(amount)
## Create a bank account then fund it
account = BankAccount()
account.balance = 1000
## Simulate concurrent deposits and withdrawals with random amounts and delays
threads = [threading.Thread(target=perform_transactions, args=(account,)) for _ in range(10)]
for thread in threads:
thread.start()
for thread in threads:
thread.join()
final_balance= "€{:,.2f}".format(account.balance)
print(f"Final Balance: {final_balance}")
Our example will run for a while, executing transactions with random amounts, each subjected to a simulated network delay:
...
Deposited €178.81, New Balance: €5,075.31
Deposited €828.37, New Balance: €5,903.68
Withdrew €164.68, New Balance: €5,739.00
Withdrew €203.17, New Balance: €5,535.83
Withdrew €638.13, New Balance: €4,897.70
Deposited €456.11, New Balance: €5,353.81
Withdrew €281.57, New Balance: €5,072.24
Withdrew €480.37, New Balance: €4,591.87
Final Balance: €4,591.87
This example illustrates the three key aspects of linearizability meantioned earlier:
- Atomicity: Each deposit and withdrawal operation is atomic, meaning it's treated as a single, indivisible action thanks to the lock. This ensures that the operation either fully completes or doesn't happen at all if it's interrupted.
- Ordering: The lock also guarantees that operations are processed in the order they are received. If two threads try to perform operations at the same time, the lock forces one to wait until the other is finished, maintaining a sequential order of effects on the balance.
- Consistency: The final state of the bank account (its balance) reflects the total sequence of operations performed on it, as if they had been executed one after another, in some order, without interference from other operations.
This simple Python example models a linearizable system where the sequence and effects of operations (deposits and withdrawals) are predictable and consistent, mirroring the key principles of linearizability in distributed systems and data engineering.
Append
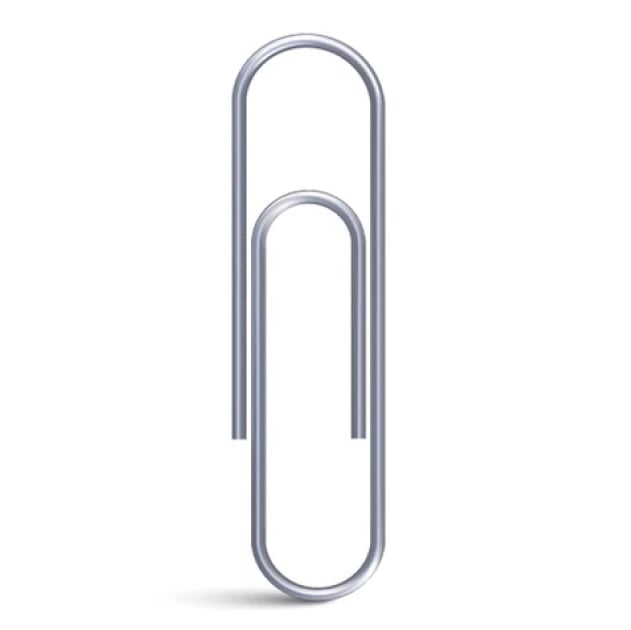
Archive
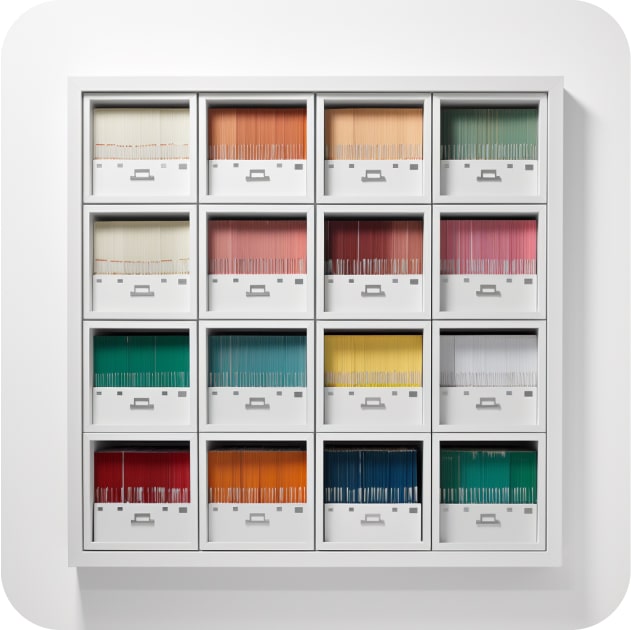
Augment
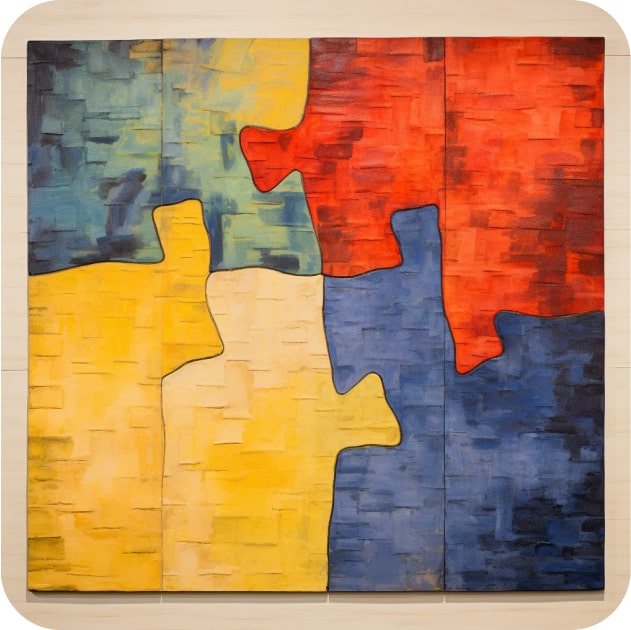
Auto-materialize
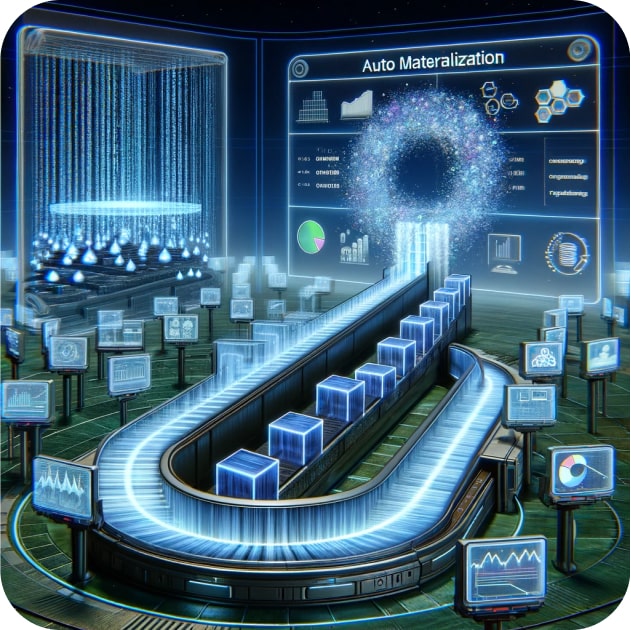
Backup
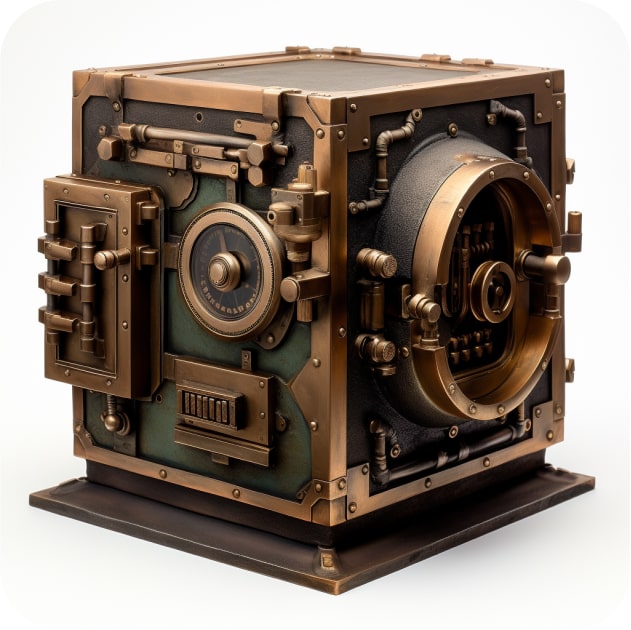
Batch Processing
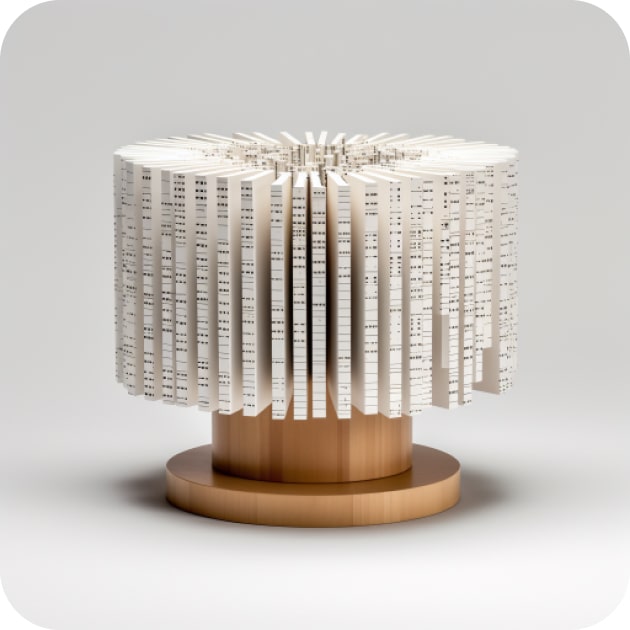
Cache
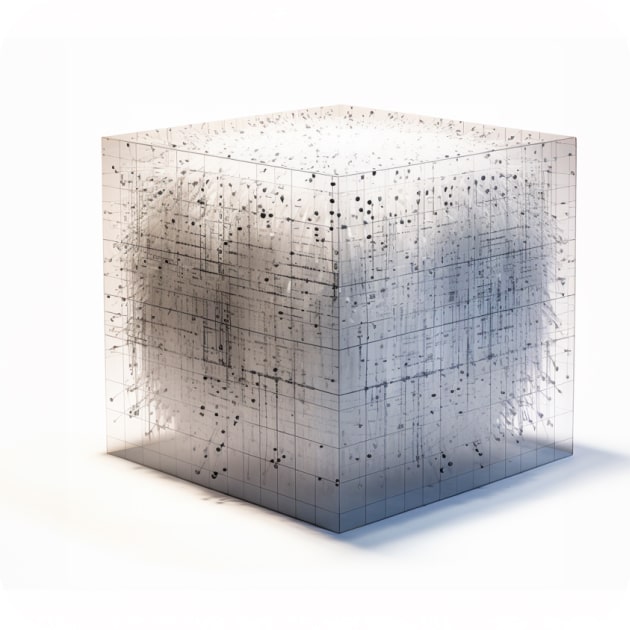
Categorize
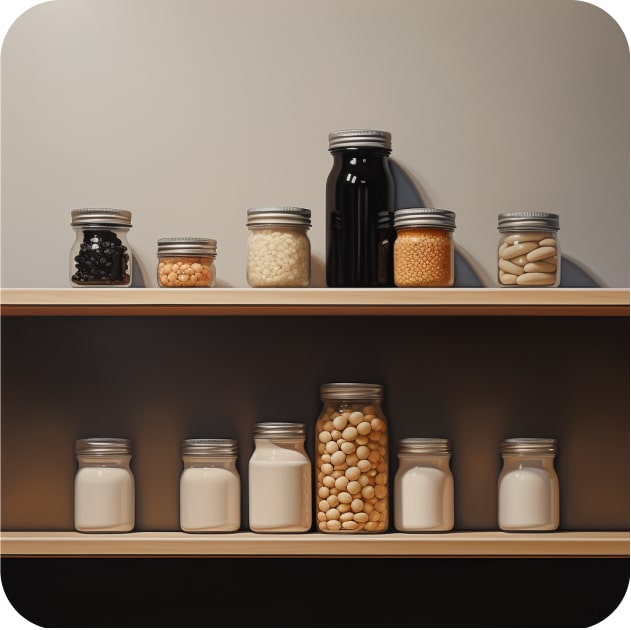
Checkpointing
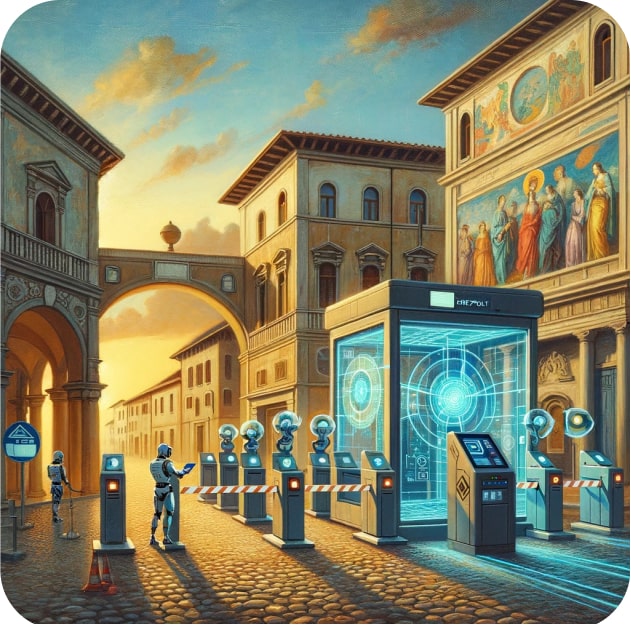
Deduplicate
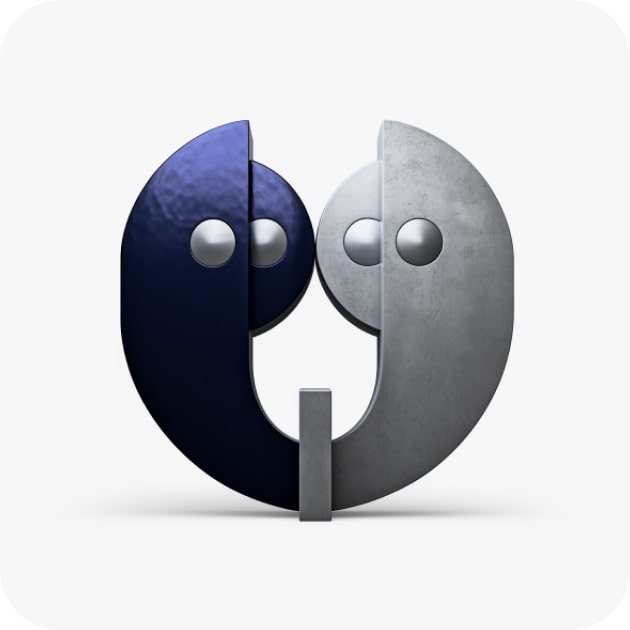
Deserialize
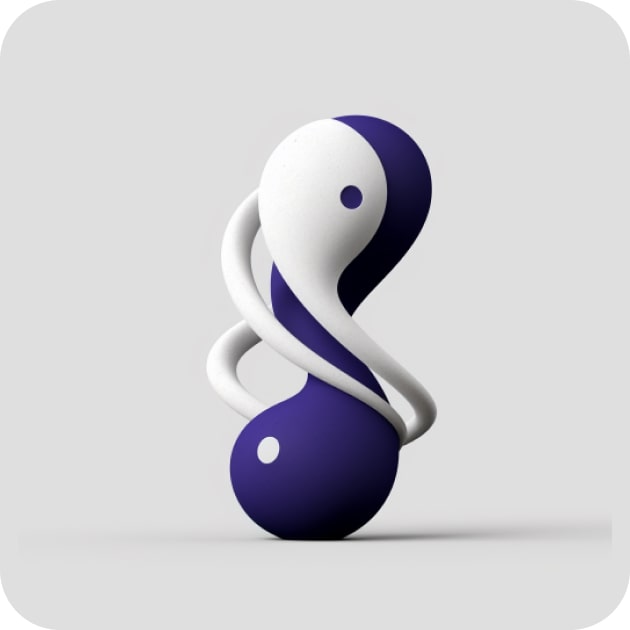
Dimensionality
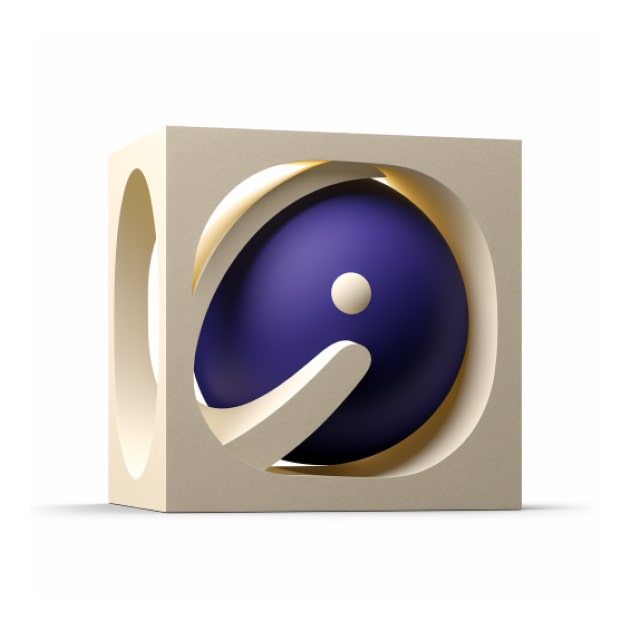
Encapsulate
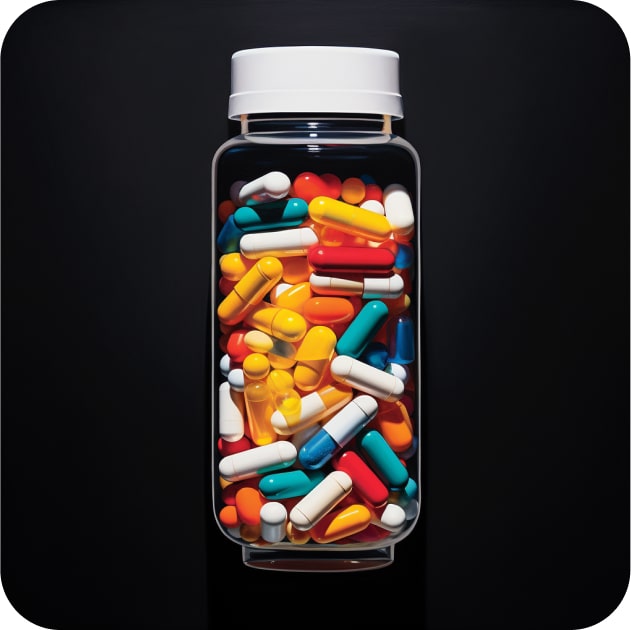
Enrich
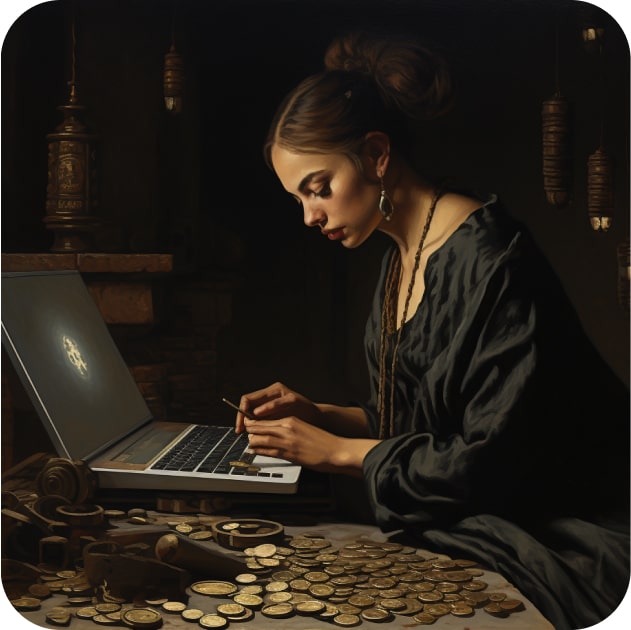
Export
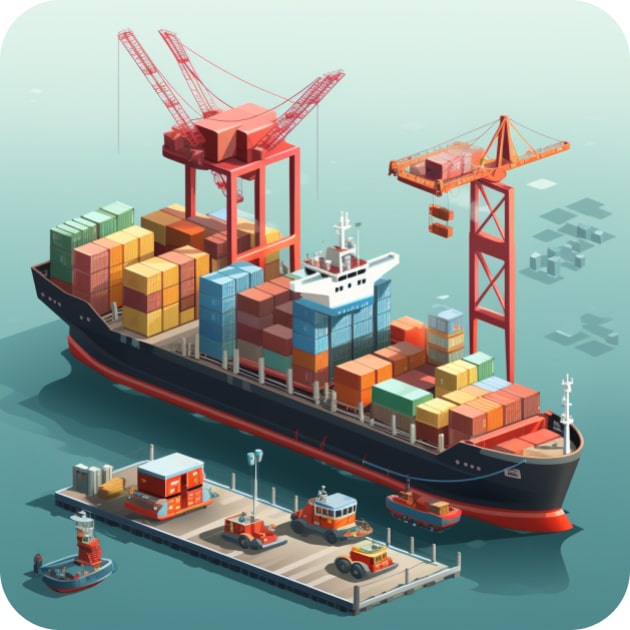
Graph Theory
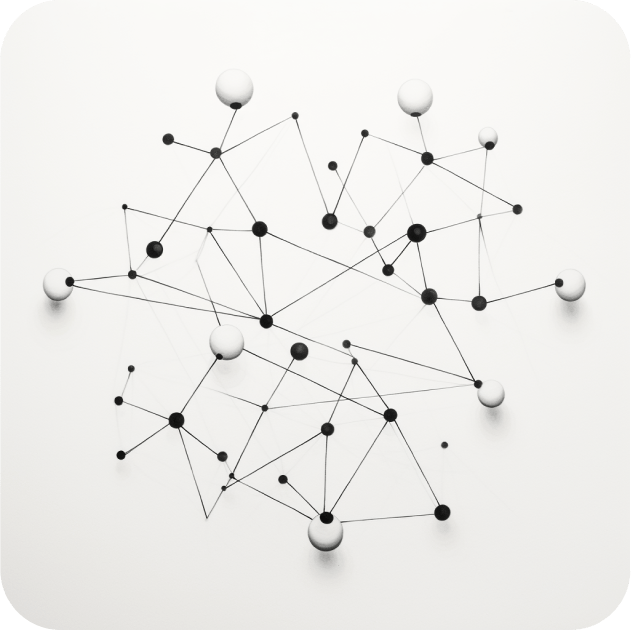
Idempotent
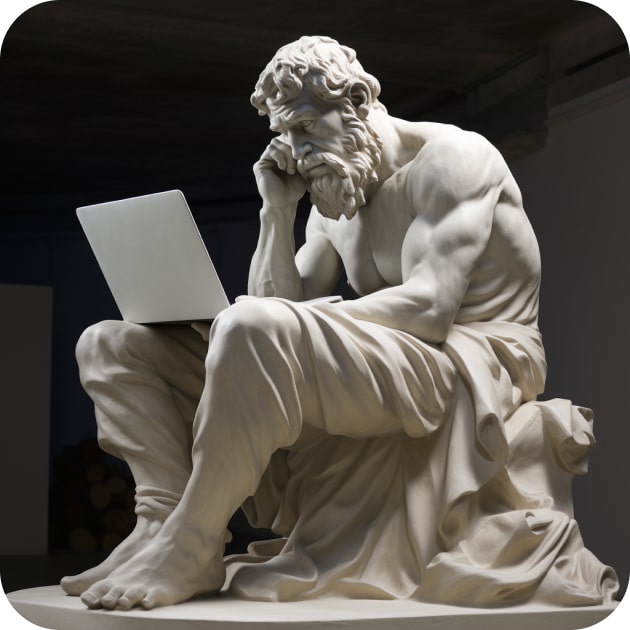
Index
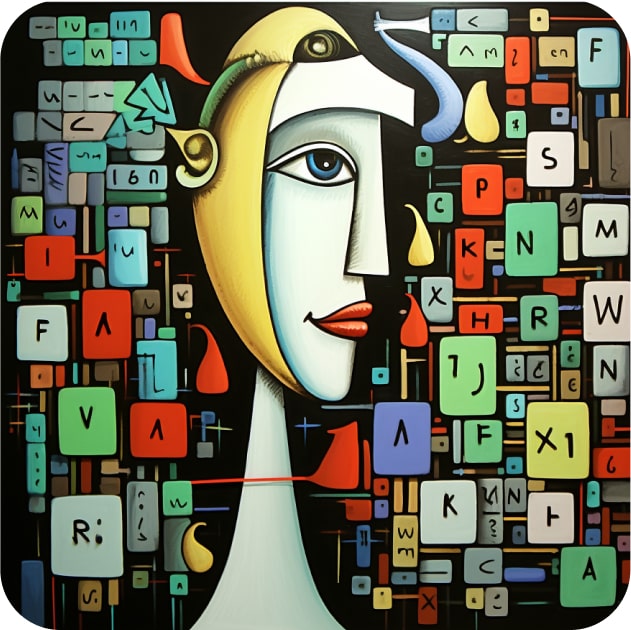
Integrate
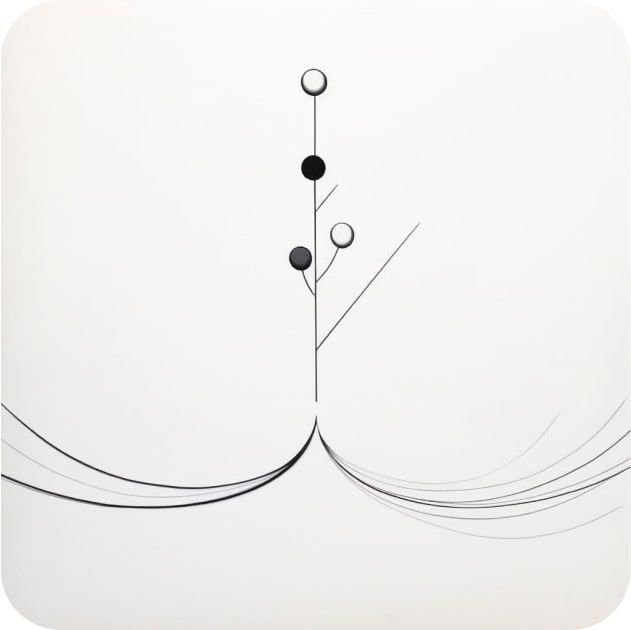
Lineage
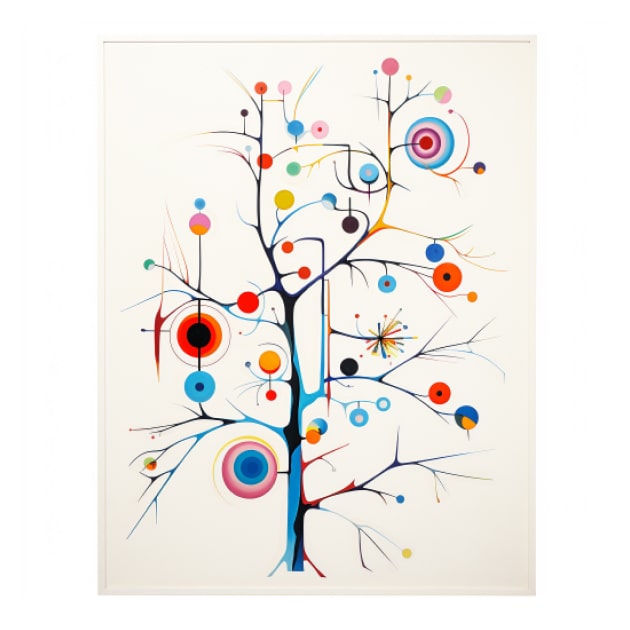
Materialize
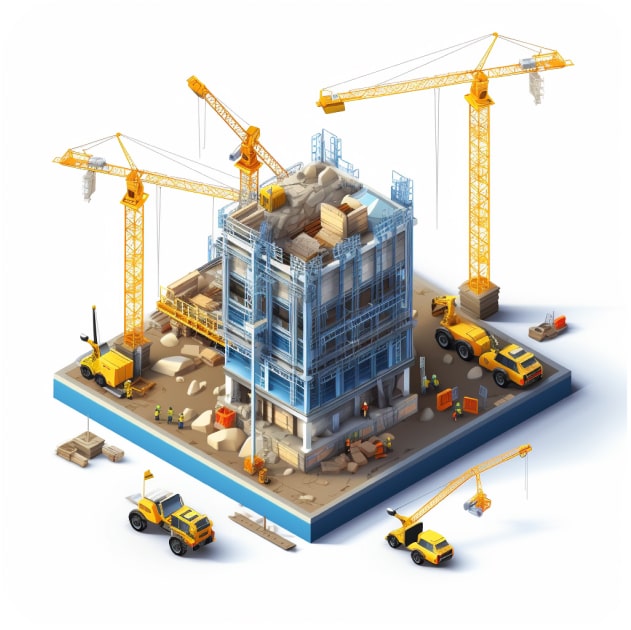
Memoize
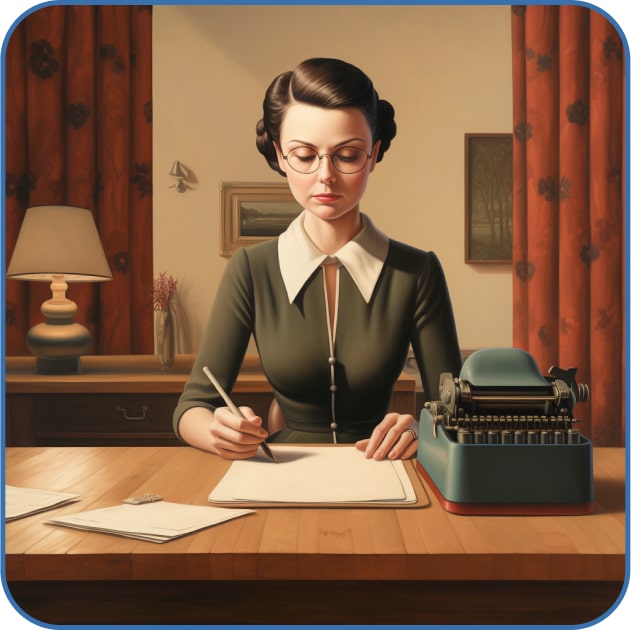
Merge
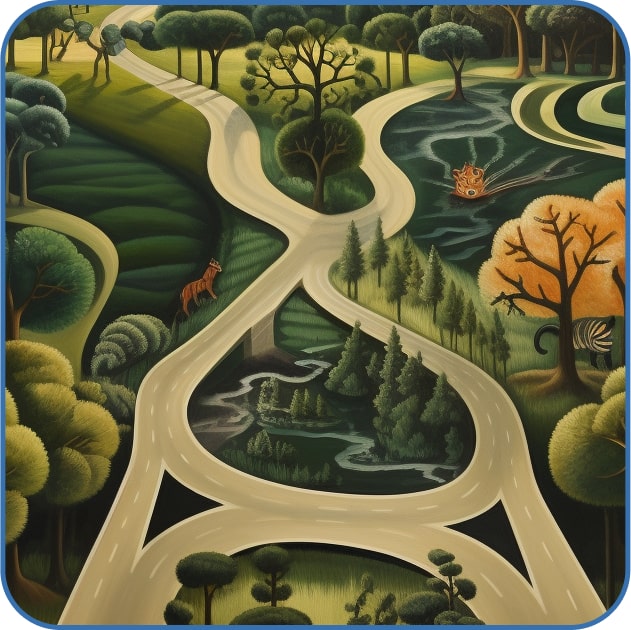
Model
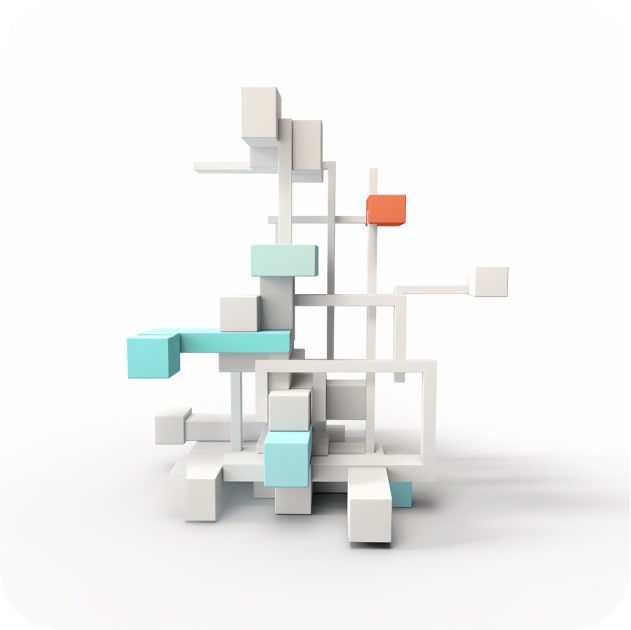
Monitor
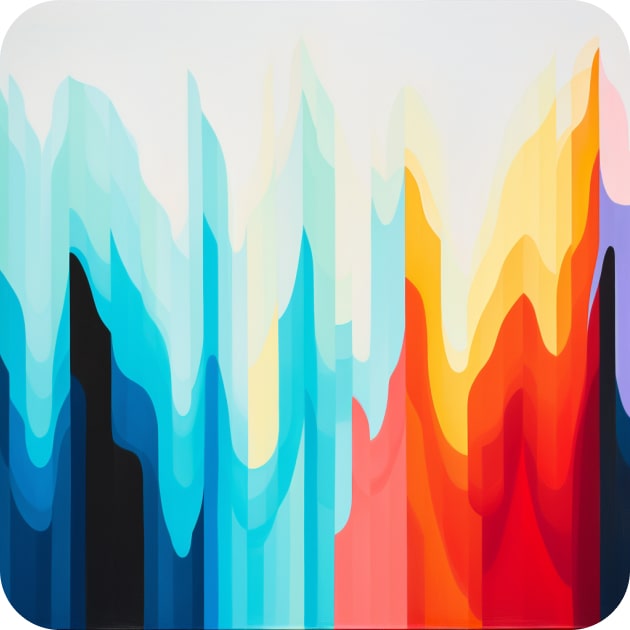
Named Entity Recognition
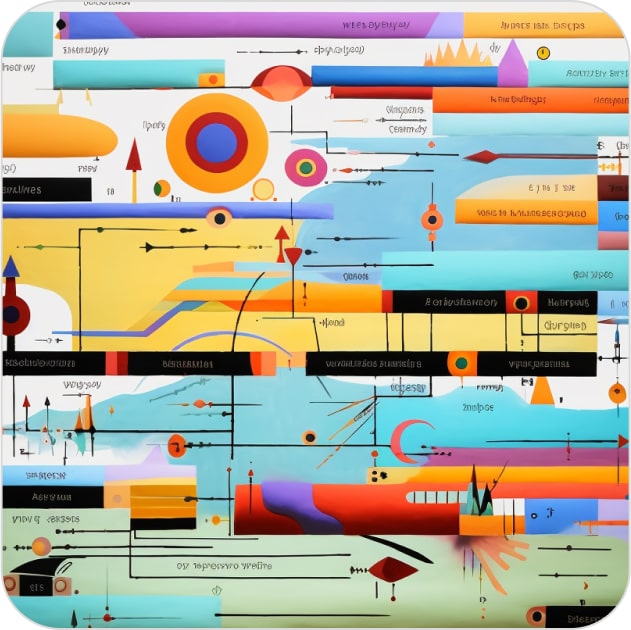
Parse
Partition
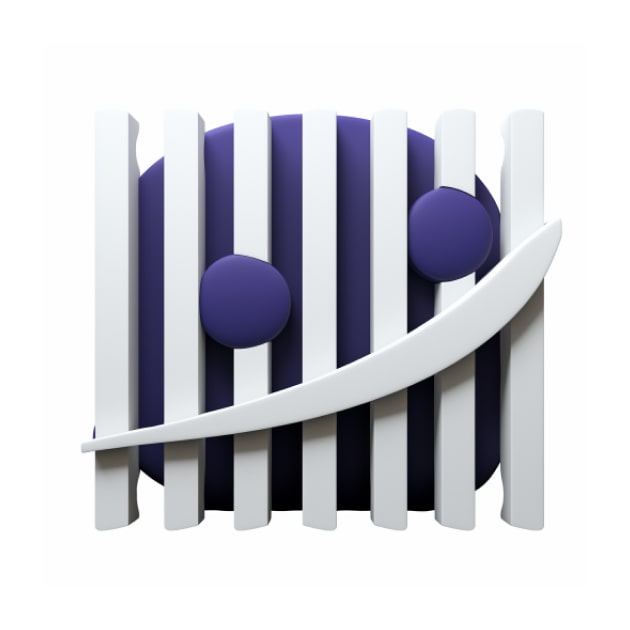
Prep
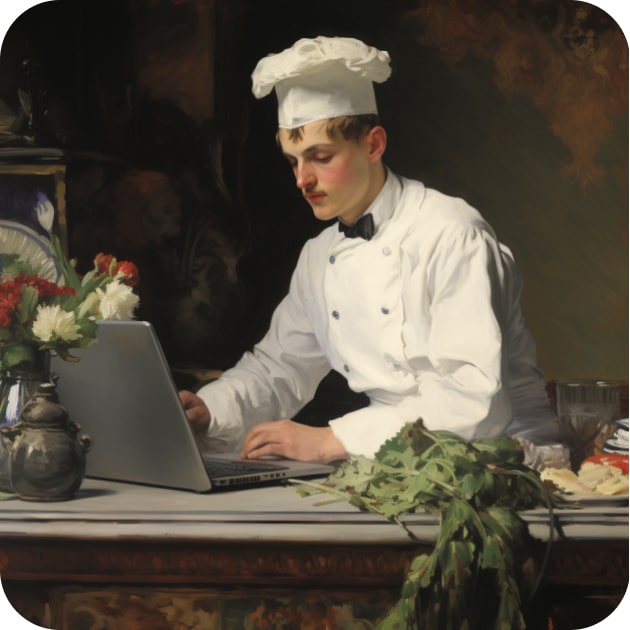
Preprocess
Primary Key
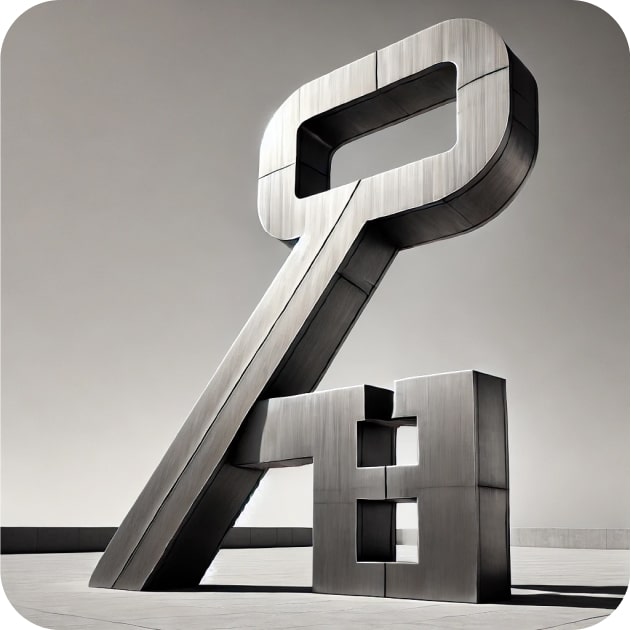
Replicate
Scaling
Schema Inference
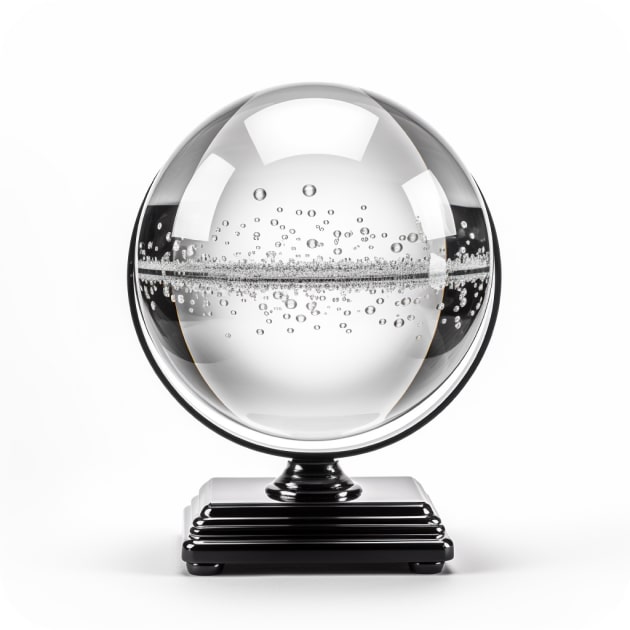
Schema Mapping
Secondary Index
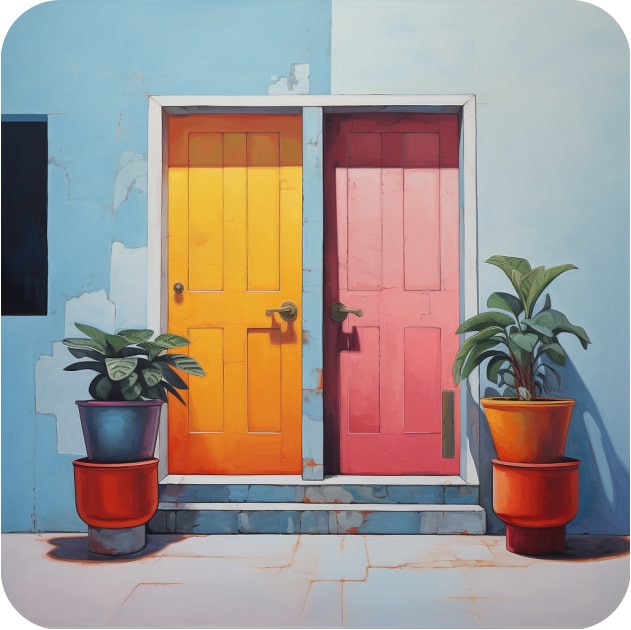
Software-defined Asset
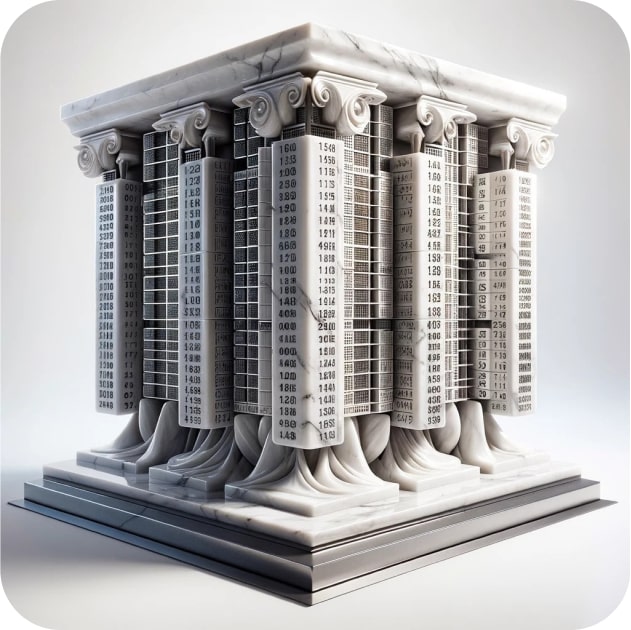
Synchronize
Validate
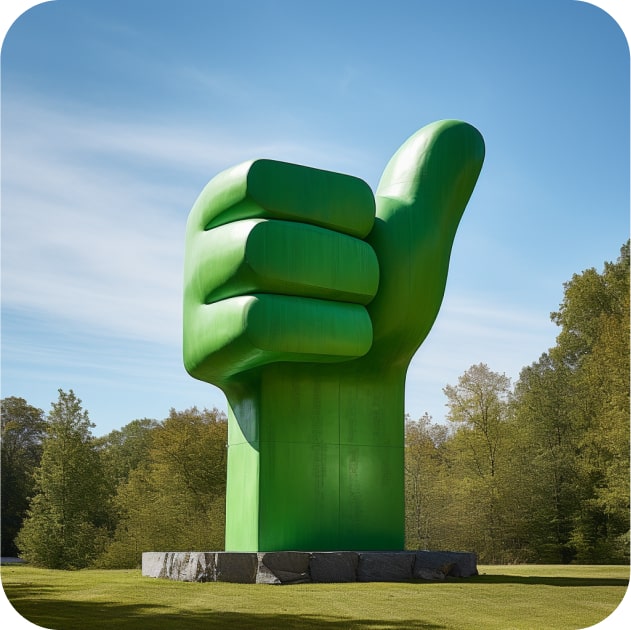
Version
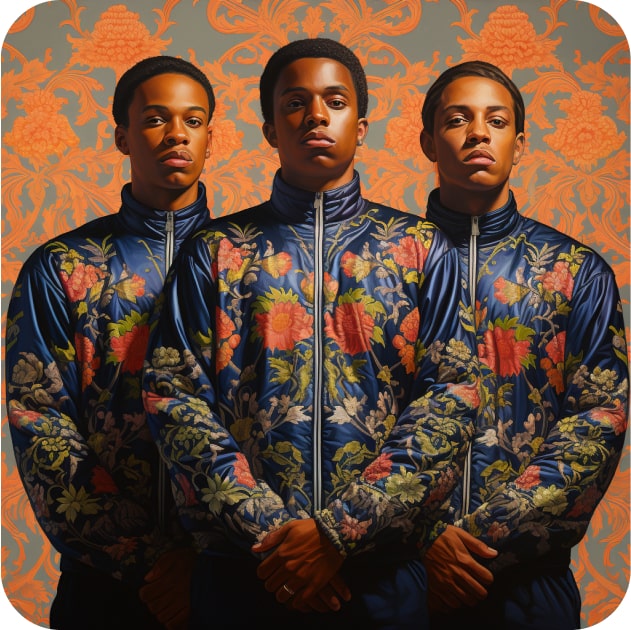