Data prep definition:
Data preparation refers to the process of cleaning, transforming, and enriching raw data into a desired format for downstream analytics or machine learning models, often involving steps like handling missing data, data type conversion, normalization, and feature extraction.
In data engineering, data preparation can refer to a broad range of activities on a large scale, using distributed systems. Data cleaning (or cleansing), imputating or transforming are just some techniques used in data prep.
Data prep example using Python:
Let's look at a simple example where we'll ingest a CSV file, clean the data, and transform it via imputation before using in a hypothetical machine learning model. Please note that you need to have the necessary Python libraries installed in your Python environment to run this code.
Let's say we have a simple input file called data.csv
:
Age,Salary,City,Is_Smoker
25,50000,New York,Yes
32,70000,Los Angeles,No
29,,San Francisco,Yes
42,90000,New York,No
36,60000,Los Angeles,Yes
27,65000,New York,Yes
,75000,Los Angeles,No
33,80000,San Francisco,Yes
40,95000,New York,No
Let's assume that we want to predict the Is_Smoker
column in the data. We can define target
as this column and use the rest of the columns as features.
In this example, the Age
and Salary
columns are numeric, the City
column is categorical, and the Is_Smoker
column is binary. There are also some missing values in the Age
and Salary
columns. So our little model cannot run with these missing values.
We will run a simple data preparation script to fill these in with the mean value of the respective column.
import pandas as pd
from sklearn.model_selection import train_test_split
from sklearn.preprocessing import StandardScaler
# Load data from CSV file
data = pd.read_csv('data.csv')
print("Original data:\n", data.head(), "\n")
# Handle missing data - Simple imputation: fill missing values with mean
data = data.fillna(data.mean())
print("Data after filling missing values:\n", data.head(), "\n")
# Define target variable
target = data['Is_Smoker']
data = data.drop('Is_Smoker', axis=1)
# Data Transformation - convert categorical data to numerical data using one-hot encoding
data = pd.get_dummies(data)
print("Data after one-hot encoding:\n", data.head(), "\n")
# Feature Scaling - Standardize features by removing the mean and scaling to unit variance
scaler = StandardScaler()
scaled_features = scaler.fit_transform(data)
print("Scaled features:\n", scaled_features[:5], "\n") # Print the first 5 rows
# Split data into training and testing sets
X_train, X_test, y_train, y_test = train_test_split(scaled_features, target, test_size=0.2, random_state=42)
print(f"Training set size: {len(X_train)}, Testing set size: {len(X_test)}")
In this code, we first define the target as the Is_Smoker
column from the data and then drop this column from the data. The remaining columns are used as features for the prediction. Note that the target column in this example is categorical, so you might need to convert it into numerical data if your model requires that.
In this example:
scaled_features
andtarget
are the feature matrix and the target vector respectively. They are split into training (X_train, y_train) and test (X_test, y_test) subsets.test_size
is a float between 0.0 and 1.0 and represents the proportion of the dataset to include in the test split. In this case, 20% of the data will be used for the test set and the remaining 80% for the training set.random_state
is the seed used by the random number generator for shuffling the data. Setting arandom_state
ensures that the splits you generate are reproducible.
Note: Scikit-Learn uses stratified sampling by default when splitting a dataset using the train_test_split()
function when the target variable is binary or multiclass classification. Stratified sampling aims to ensure that each split is representative of all strata of the data. This is particularly useful when the data is imbalanced.
This script prints the first few rows of the original data, the data after filling missing values, and the data after one-hot encoding. It also prints the first 5 rows of the scaled features and the sizes of the training and testing sets.
train_test_split()
Using It is worth explaining the train_test_split()
function from the sklearn.model_selection
module of the Scikit-Learn library in Python. This function is used to split the data into two sets: a training set and a test set.
These two sets are used for different purposes in machine learning:
The training set is used to train the model, meaning that the model learns from this data to make predictions or decisions.
The test set is used to evaluate the model's performance, meaning that the model uses this data to make predictions and then compares its predictions to the actual values to assess its accuracy.
The train_test_split()
function shuffles the dataset using a pseudorandom number generator before making the split. This is important to ensure that the training and testing sets are representative of the overall distribution of the data.
Since we included a number of output (print
) statements, our example will produce the following:
Original data:
Age Salary City Is_Smoker
0 25.0 50000.0 New York Yes
1 32.0 70000.0 Los Angeles No
2 29.0 NaN San Francisco Yes
3 42.0 90000.0 New York No
4 36.0 60000.0 Los Angeles Yes
Data after filling missing values:
Age Salary City Is_Smoker
0 25.0 50000.0 New York Yes
1 32.0 70000.0 Los Angeles No
2 29.0 73125.0 San Francisco Yes
3 42.0 90000.0 New York No
4 36.0 60000.0 Los Angeles Yes
Data after one-hot encoding:
Age Salary City_Los Angeles City_New York City_San Francisco
0 25.0 50000.0 0 1 0
1 32.0 70000.0 1 0 0
2 29.0 73125.0 0 0 1
3 42.0 90000.0 0 1 0
4 36.0 60000.0 1 0 0
Scaled features:
[[-1.5 -1.73607121 -0.70710678 1.11803399 -0.53452248]
[-0.1875 -0.23460422 1.41421356 -0.89442719 -0.53452248]
[-0.75 0. -0.70710678 -0.89442719 1.87082869]
[ 1.6875 1.26686278 -0.70710678 1.11803399 -0.53452248]
[ 0.5625 -0.98533771 1.41421356 -0.89442719 -0.53452248]]
Training set size: 7, Testing set size: 2
Append
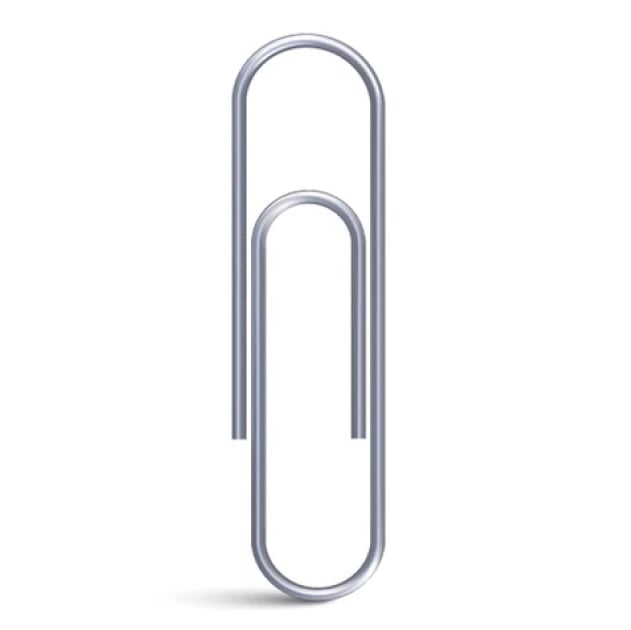
Archive
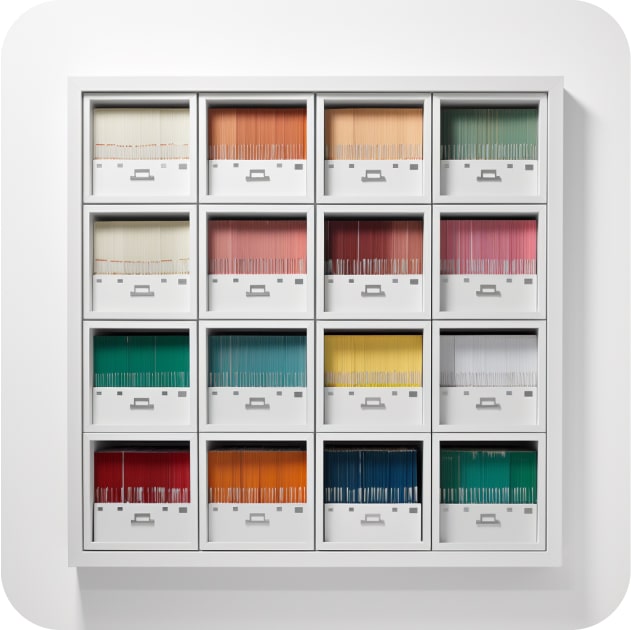
Augment
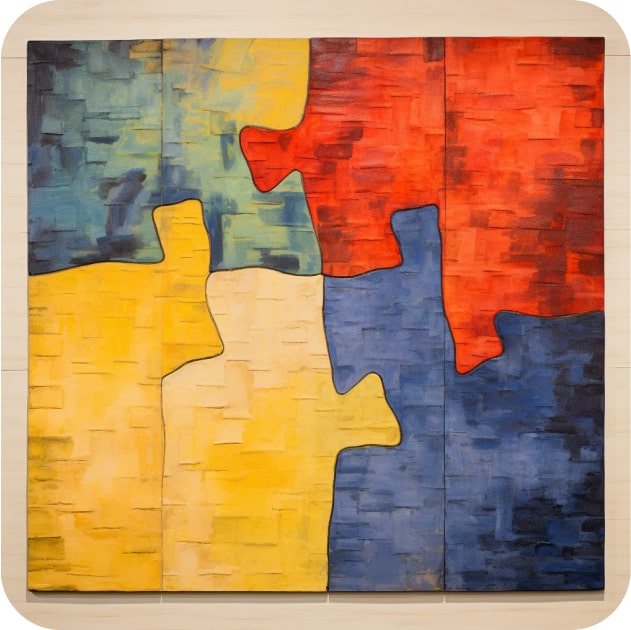
Auto-materialize
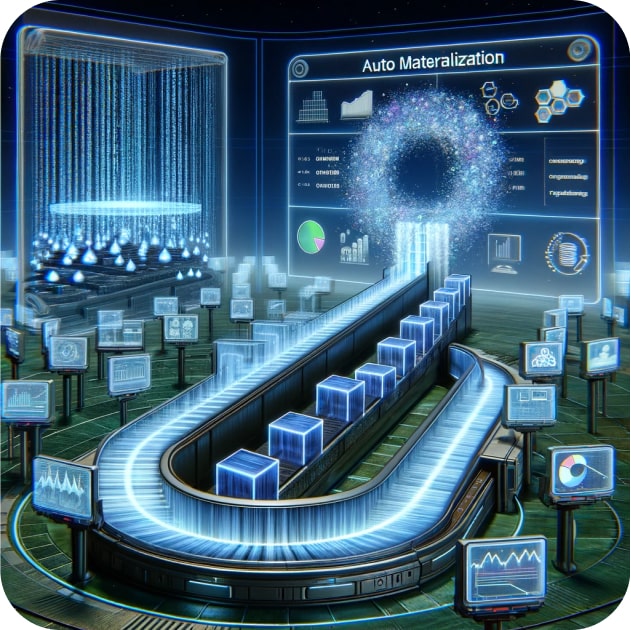
Backup
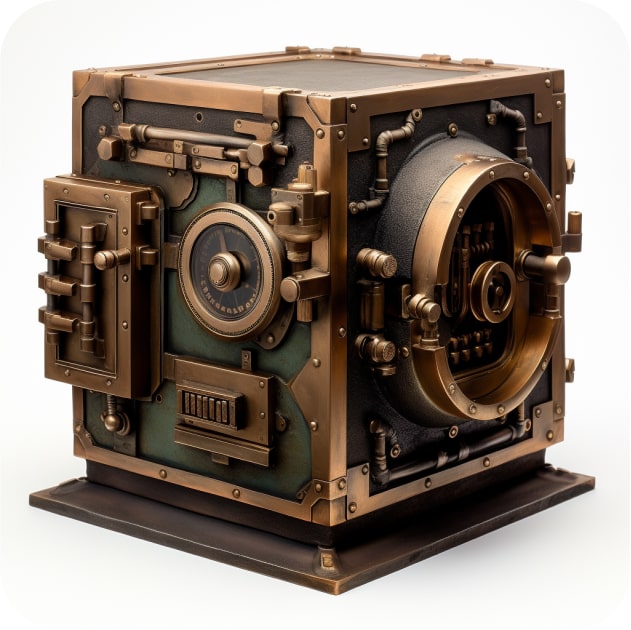
Batch Processing
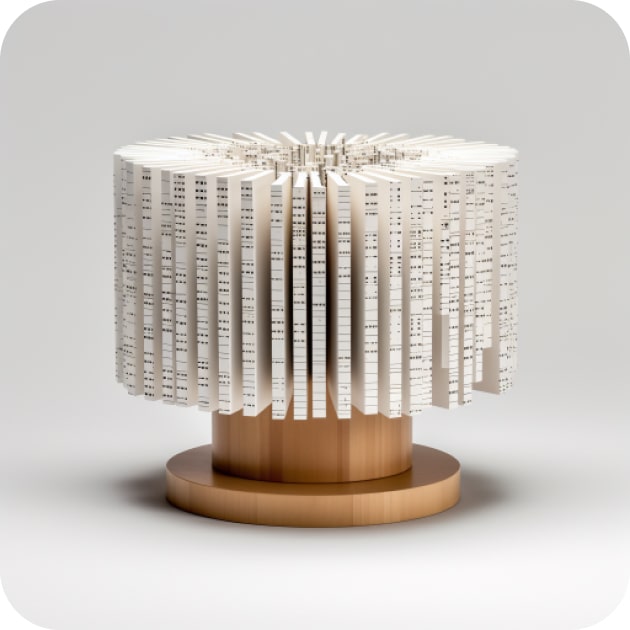
Cache
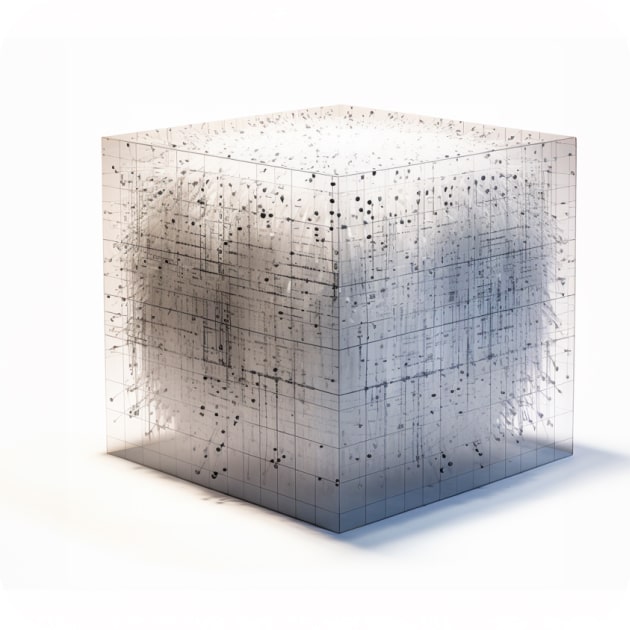
Categorize
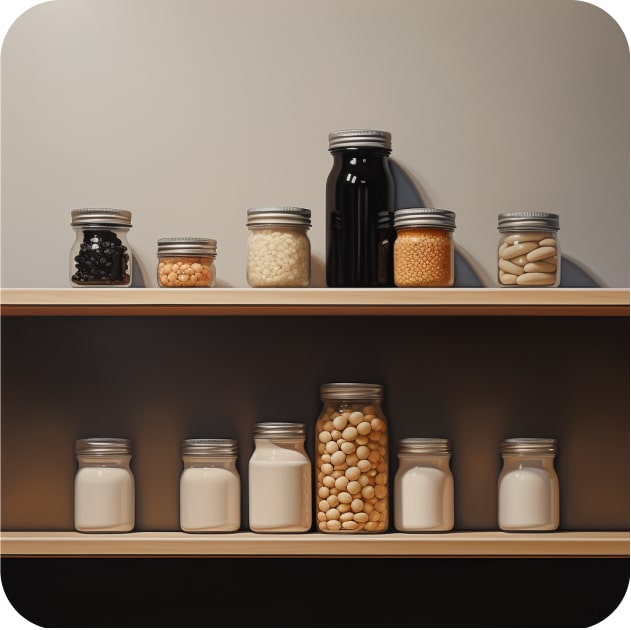
Deduplicate
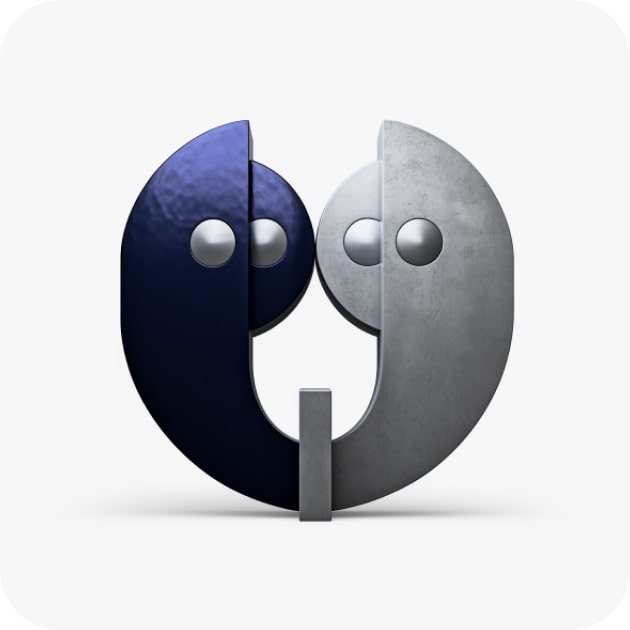
Deserialize
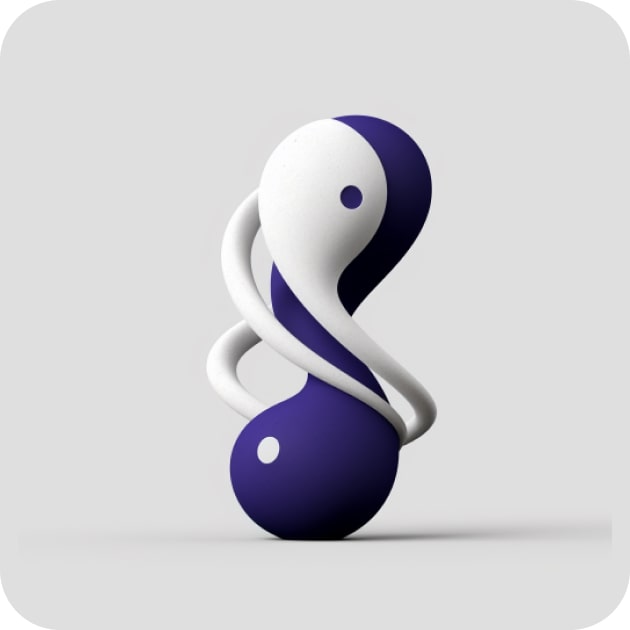
Dimensionality
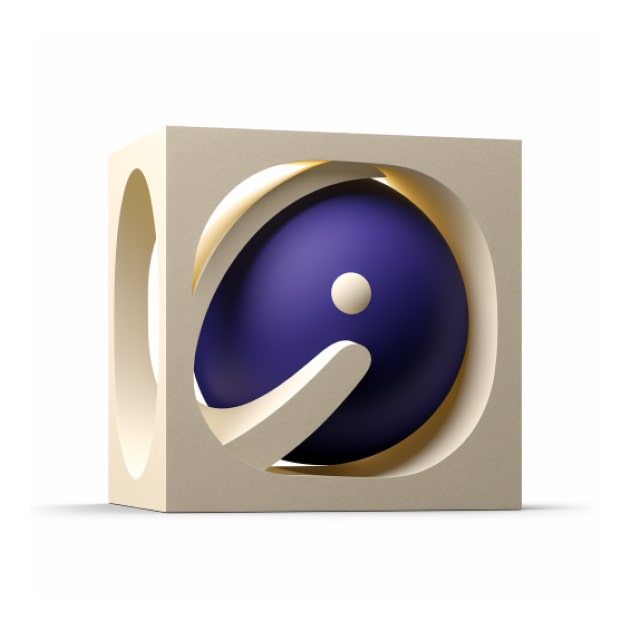
Encapsulate
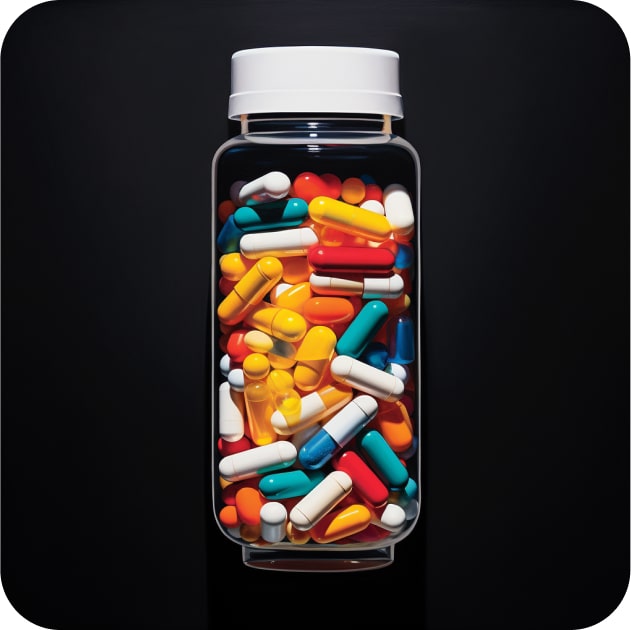
Enrich
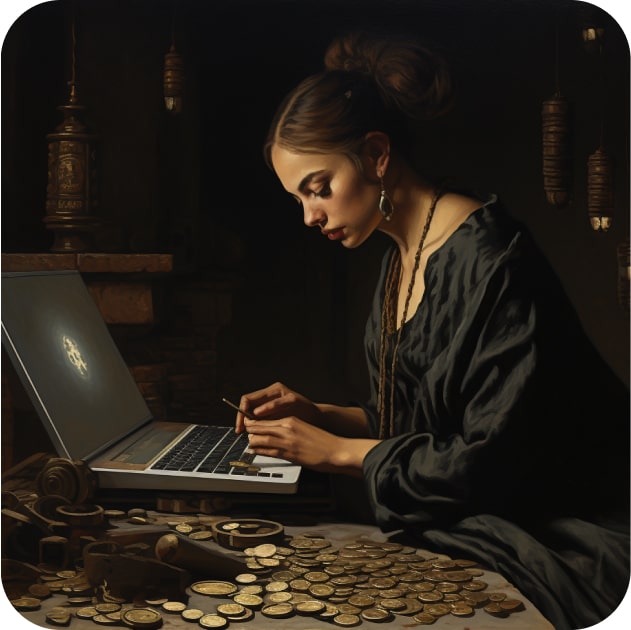
Export
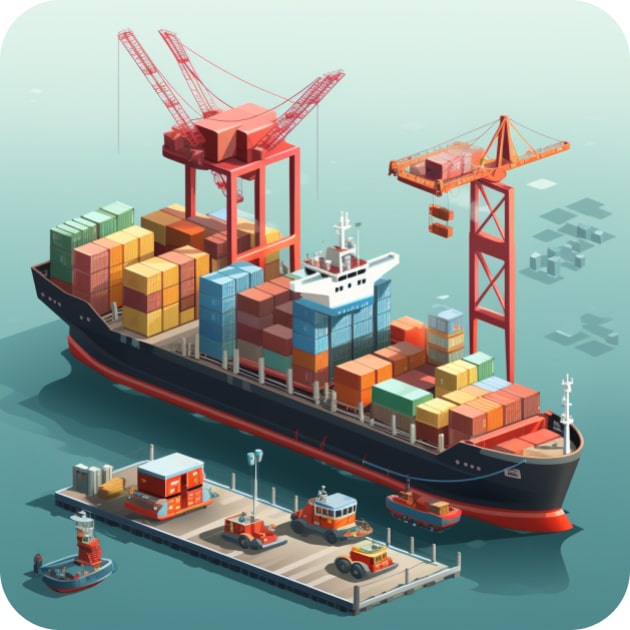
Graph Theory
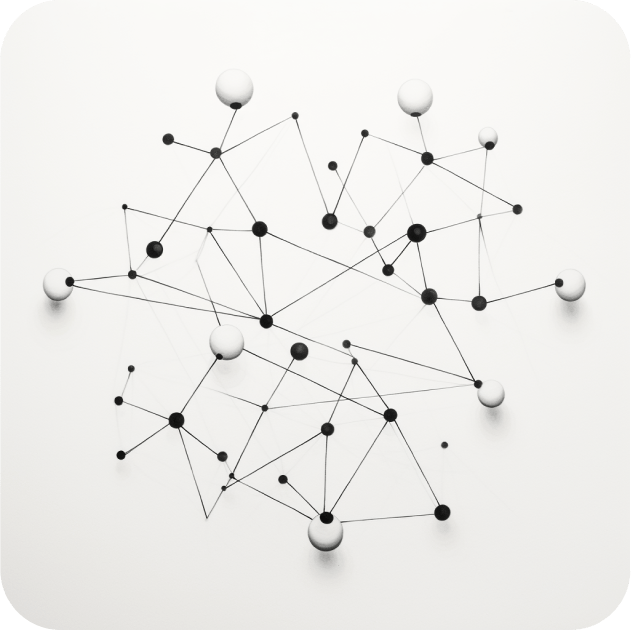
Idempotent
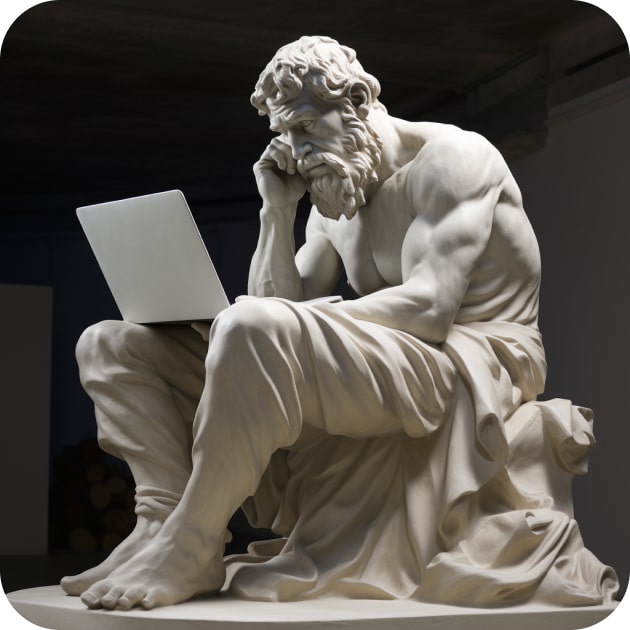
Index
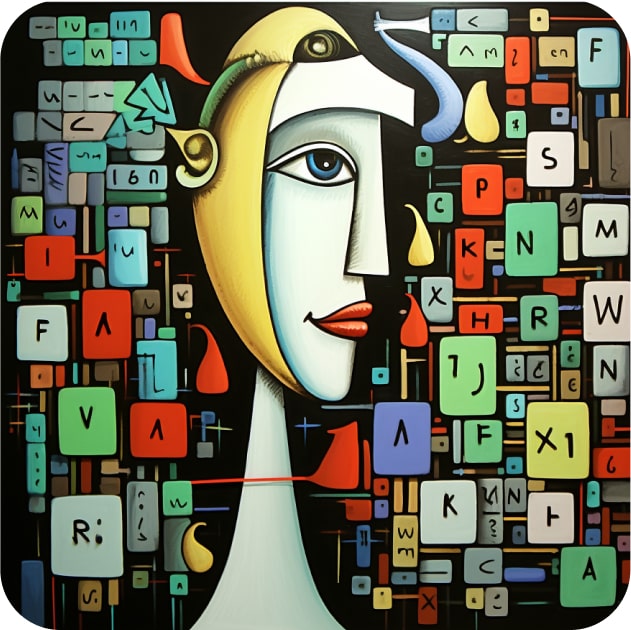
Integrate
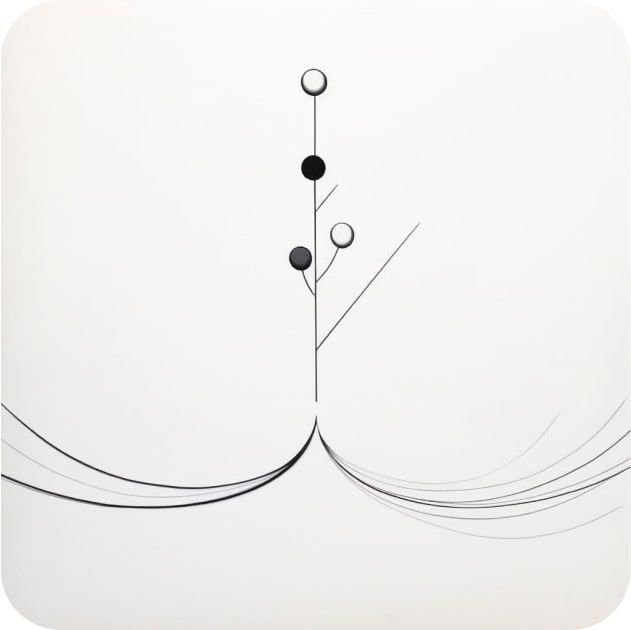
Lineage
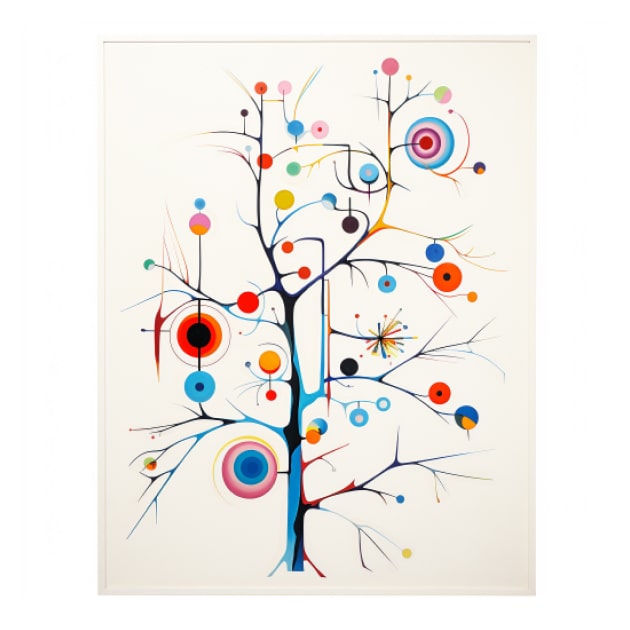
Linearizability
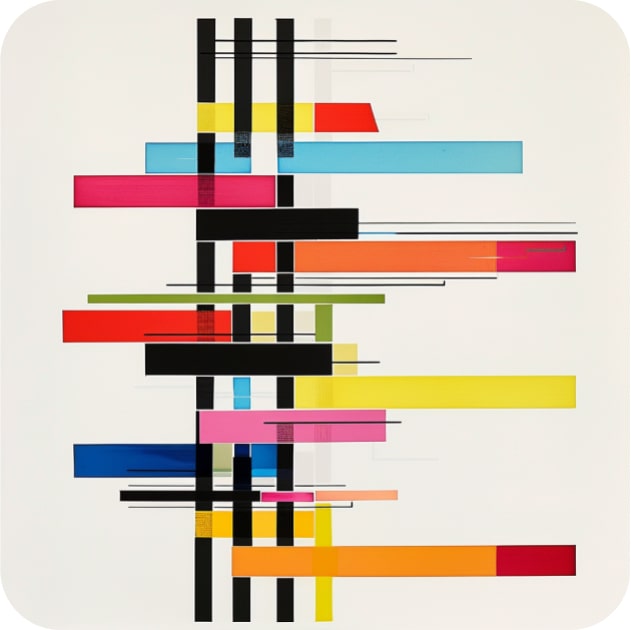
Materialize
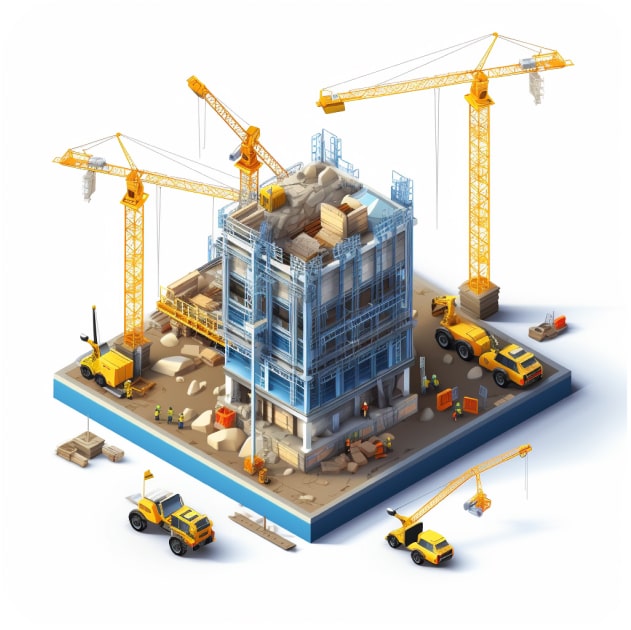
Memoize
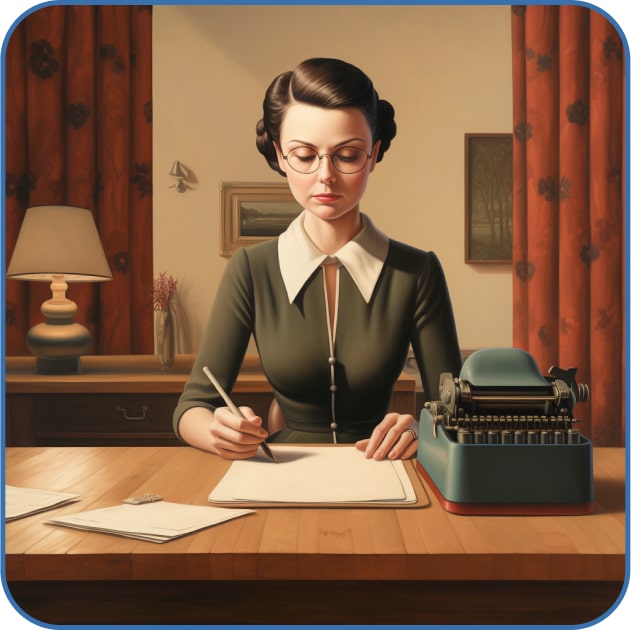
Merge
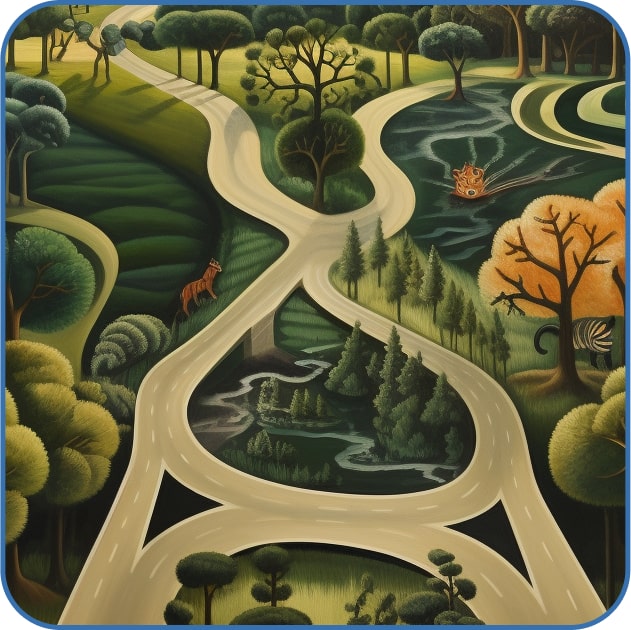
Model
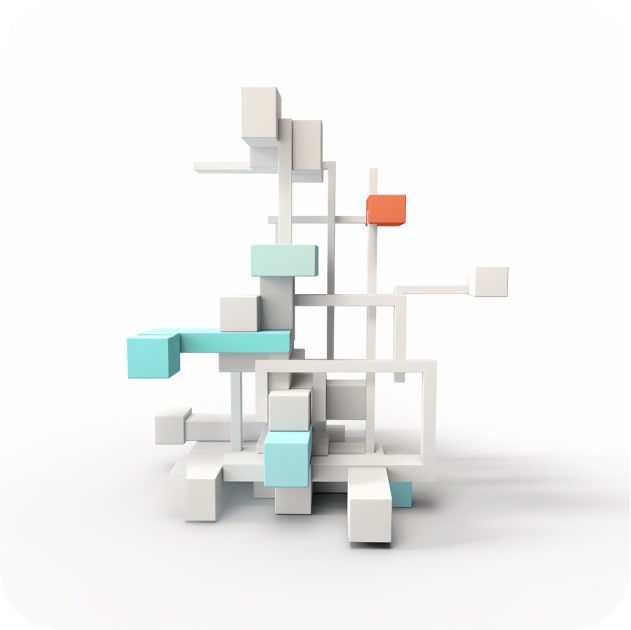
Monitor
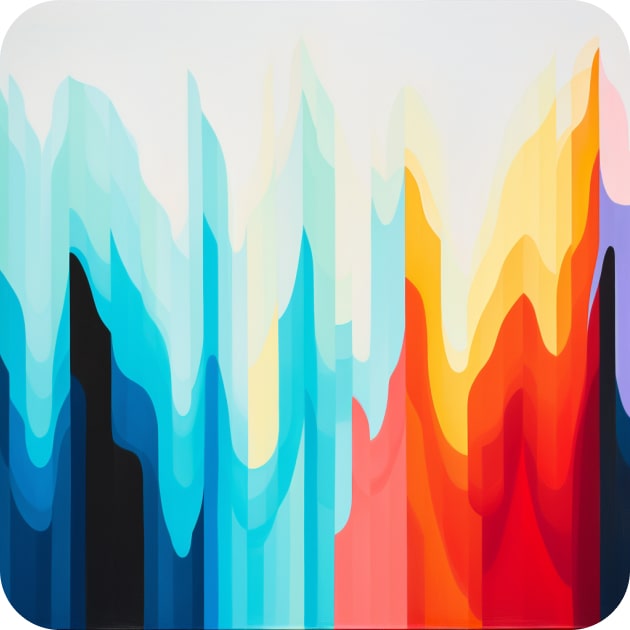
Named Entity Recognition
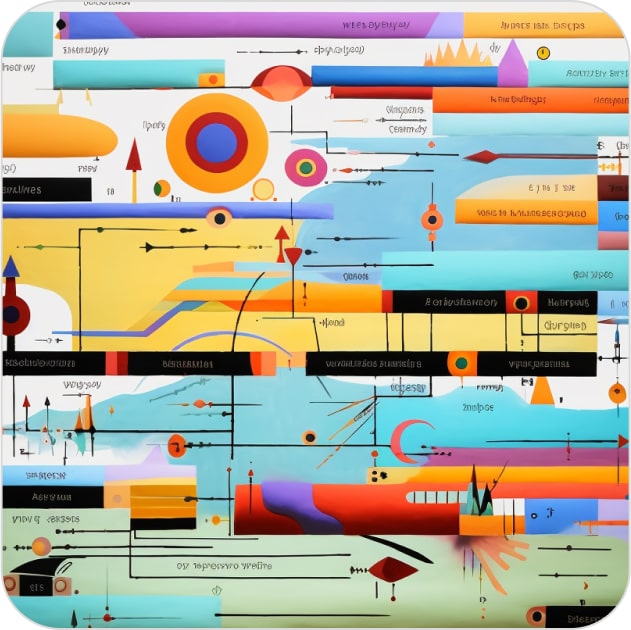
Parse
Partition
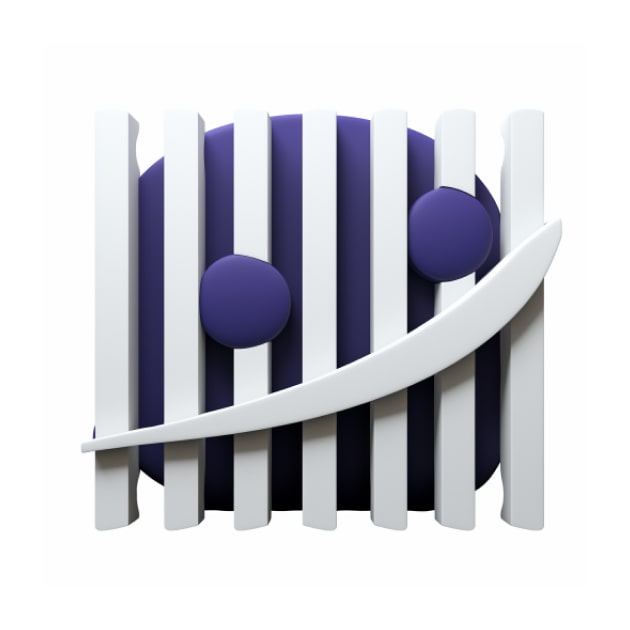
Preprocess
Replicate
Scaling
Schema Inference
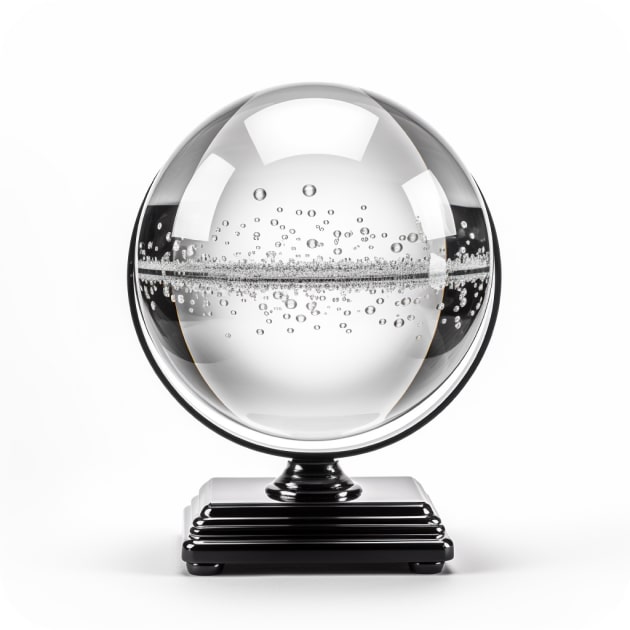
Schema Mapping
Secondary Index
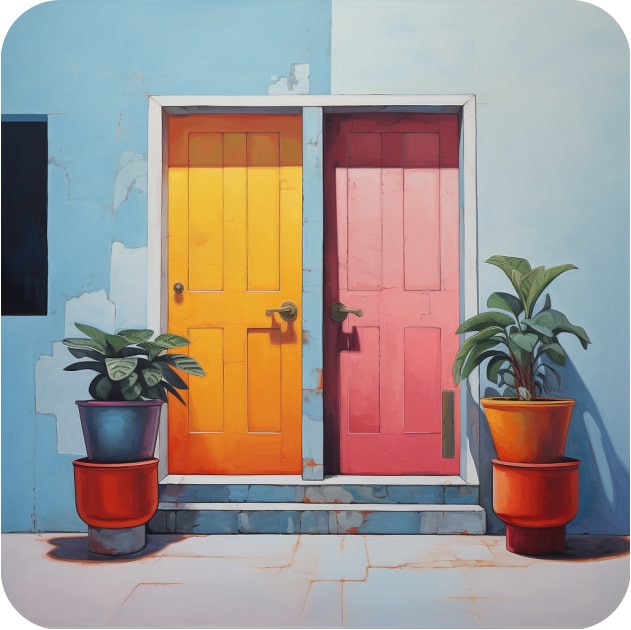
Software-defined Asset
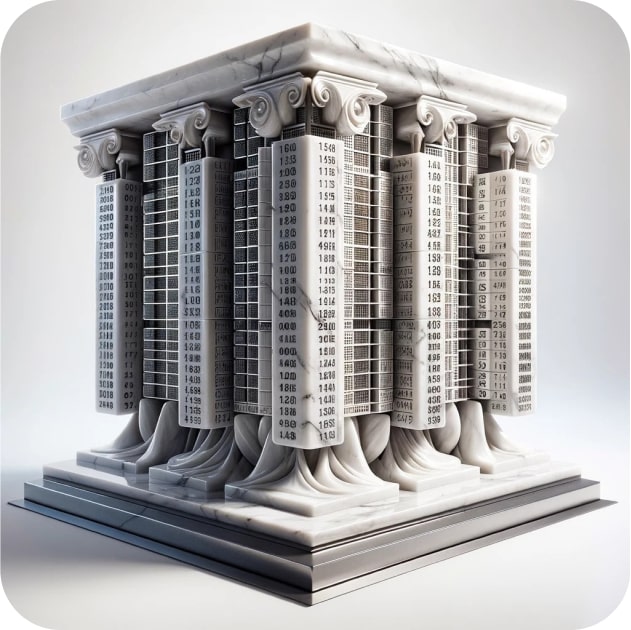
Synchronize
Validate
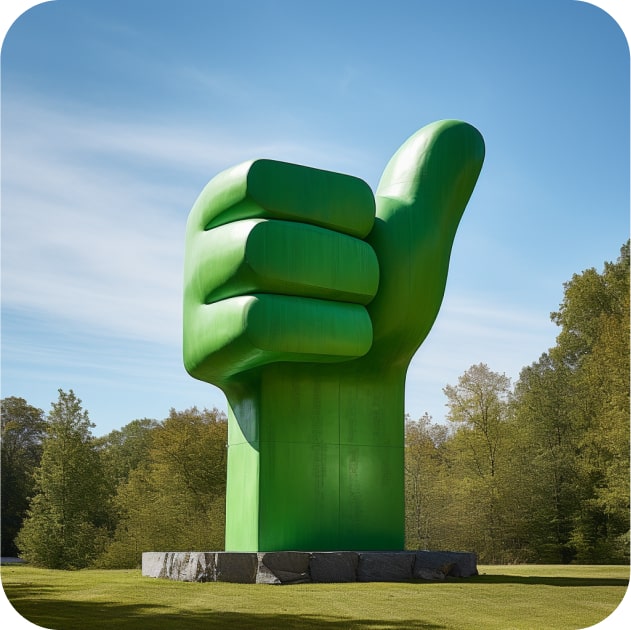
Version
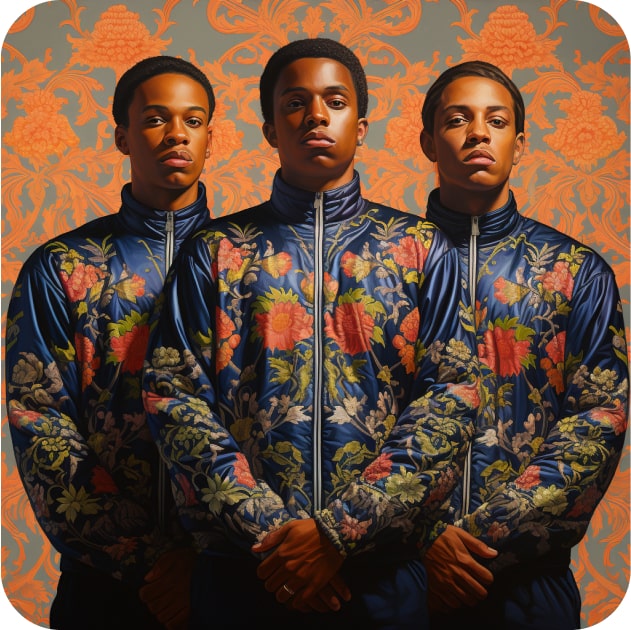